« January 2007 | Main | March 2007 »
February 25, 2007
Things to read while the simulator runs; part 3
Who knew that you could fold a single piece of paper into a silverfish, or a chinese dragon? This story is about a physicist who dropped out of research to become a professional oragami folder. I'm still wrapping my head around that picture of the dragon... (via 3 Quarks Daily)
I've long been interested in video games (purely on an academic basis, I assure you), and have often found myself defending them to non-gamers. Sometime soon, I'll blog about a recent trip to a SFI business workshop that focused on how to make various kinds of office work more interesting by borrowing ideas from games, but that's not the point of this entry. Rather, I want to point you to the results of a comprehensive meta-analysis of the connection between violent behavior and violent video games. The conclusion? That current research doesn't support any conclusions. (via Ars Technica)
As someone who spent various numbers of grade-school years in both tracked gifted programs and non-tracked general education, Alexandre Borovik's notes on how mathematically gifted children's brains operate differently from normal children resonated deeply with me. One that's probably quite prevalent is
[A mathematically] able child can show no apparent interest in mathematics whatsoever because he or she is bored by a dull curriculum and uninspiring teacher.
Apparently, Alexandre has a whole book on the topic of "What is it that makes a mathematician." (via Mathematics under the Microscope)
Update Feb 27: Yesterday, I came across an interesting article in the New York Magazine about the problem with praising children for their intelligence rather than their hard work. The research described herein seems both simple and persuasive - praising kids for their intelligence encourages them to not take risks that might make them look unintelligent, while praising kids for their hard work encourages them to apply themselves, even to problems beyond their capabilities. I can't help but wonder if the tendency to praise mathematically talented kids for being smart, rather than hard working, somehow tends to push girls out of the field. And finally, on the subject of hard work, Richard Hamming says that this is partially what distinguishes great researchers from the merely good. End Update.
Finally, here's a great little video. Not sure about the whole "teaching the Machine" thing, but otherwise, spot on and very well done.
posted February 25, 2007 01:23 AM in Things to Read | permalink | Comments (0)
February 24, 2007
Time traveling birds
If anyone still needed to be convinced that birds are strange, strange creatures - creatures bizarrely capable of much of what we consider to be inherently human behavior - they need only grok the recent collapse of the Bischof-Kohler hypothesis [1] (tip to New Scientist). Of course, if you're like me, you hadn't heard of the B-K hypothesis previously, and its collapse would have gone completely unnoticed except for my ongoing fascination with bird brains. In a brief letter to Nature, four experimental psychologists describe a simple experiment with western scrub-jays (Aphelocoma californica). The experiment [2] regulated the amount of food available to the jays in the evening and the mornings; on one morning, the jays would be given no food, but on the previous evening they were given more food than they needed. The jays spontaneously and consistently cached some of that extra food for the following morning, when they would be hungry and without food. The researchers say,
These results challenge the Bischof-Kohler hypothesis by demonstrating that caching on one day was controlled by the next day's motivational state and available resources.
So, we can now add time travel to the growing list of human-like behaviors that birds seem to exhibit (others include rudimentary language, basic knowledge of the integers, creativity, tool use, object permanence, cause-and-effect, and that most human of behaviors, deception). I also find it interesting that birds typically only exhibit one or a few of these abilities, suggesting not only that they are located or controlled by different parts of their brains, but also that they are not all essential to survival. It certainly wouldn't surprise me if these behaviors turned out to be a lot more common in the animal world than we give them credit.
Update, April 3, 2007: Carl Zimmer writes in the New York Times Science section about different kinds of time-traveling behavior in several species (including rats, monkeys and humans), and writes at length about the scrub jay's abilities. He also blogs about his article and links to some of the recent science on the topic. End Update
Raby, Alexis, Dickinson and Clayton, "Planning for the future by western scrub-jays." Nature 445 919-921 (2007).
-----
[1] The Bischof-Kohler hypothesis says, in short, that the ability to (mentally) travel in time is uniquely human. It's clear that humans can do this because we talk about it all the time - we can call up vivid, episodic memories of past experiences or make complex plans for future contingencies in a way not reminiscent of operant conditioning. Since animals can't talk to us directly, we can only hope for indirect evidence that animals can (mentall) time travel. There's some evidence that primates can time travel (for instance), but since they're our cousins anyway, perhaps that's not too surprising. Given the complexity of their behavior, I imagine that elephants would also pass the time-travel test.
[2] I find it amusing that the researchers called their experiment "planning for breakfast," but maybe that's because normally scientific prose is so filled with jargon that a completely sensicle and common name for a scientific term seems like a bolt out of the blue.
posted February 24, 2007 08:37 AM in Obsession with birds | permalink | Comments (0)
February 22, 2007
TravelBlog: Korea
Photos from my trip to Korea are now up on my flickr stream.
Normally, I'd write a lengthy entry of my reflections on my time in Korea. But, since this trip was primarily for business, it wasn't quite the same kind of trip I normally make. Normally, I won't lug my computer around, stay in fancy hotels, or spend much time with people who speak my language. Having cut my teeth backpacking solo in Europe many years ago, I much prefer being "close to the ground" where I can get a better sense of what the local culture is like (with the obvious caveat that this can be hard to do when you stick out like a sore thumb and don't speak the language!).
So, I have only a few thoughts this time. For instance, Korea and Japan are extremely similar in many ways, both culturally, visually, and in the overwhelming ubiquity of digital technology. Much of the traditional architecture looks roughly the same to my unaccustomed eye, although I'm told there are important differences. One that stood out the most was the Korean emphasis on greens (for instance, this structure) versus the Japanese emphasis on reds (like this one). Another architectural observation is that many of the buildings look like they were inexpensive derivatives of the brutalist architectural movement, i.e., lots of uninteresting, high-rise concrete buildings, probably hastily erected after the Korean War flattened most everything that was there before.
On the technological side (which admittedly fascinated me more than the architecture did), I was amazed at how pervasive digital technology was, not only with mobile phones (which did television, text messaging, calls, etc., and have that little hook from which you can hang a personalizing charm - I really wish American wireless providers puts these on the handsets...), but in elevators (door-close buttons that work instantly!), parking lots (which, with a pressure pad under each parking space, display at their entrance the precise number of available spots!), and public busses, among many others. The thing that sets these countries apart from America (and much of Europe) in their use of these technologies is that they use it to explicitly make everyday activities easier and more convenient. In these ways, I think of Korea and Japan as true first-world countries, while Western nations feel more like third-world countries.
Socially, the country seems to be experiencing a similar kind of shift as Japan, with the older generation trying to preserve the social norms and traditions, while the younger generation is actively defining their own way of life. Sometimes this means embracing traditions (such as the first-place, second-place, third-place method of going out on the town), but I think it typically means moving away from them. This dynamic is pervasive in Western countries, as well, but because the social norms are that much stronger in these Eastern nations, the contrast seem that much more palpable at times.
I found Seoul to be an extremely fashionable city, and particularly so with the younger generation. Typically, it seemed, women, old and young, are elegant and beautiful, while men are sharply dressed. (I did see some people who would have fit in well in Harajuku.) If shopping is your bag, it's excellent for both club faddle and custom-made business attire. Plus, clothing (much of which is apparently Made in China), transportation (trains, busses, taxis, subways), and food are all pretty inexpensive. I had just about gotten used to the local cuisine by the time I left - it has a lot of beef and pork in it, and is quite spicy.
From talks with people who know much more than I, the educational system in Korea seems quite similar to that of Japan (and, to some extent, of China), with the main emphasis being on memorization, performance on standardized tests, respect for elders (even if they're wrong), etc. Kids in both countries, and at all ages, spend a lot of extra time studying for the various entrance exams for different grade schools, college and university. It's my own opinion that this kind of approach to education tends to discourage creative problem solving and ingenuity. These things are, I think, perhaps somehow less discouraged by the more chaotic style of education in the US, although I'm not an expert on education systems. I wonder whether the profound emphasis in these Eastern nations on mastering standardized bodies of knowledge has its roots in the ancient scholasticism that focused mastering an extremely large body of cultural, historical and mathematical facts. (Perhaps some of my several Korean and Japanese readers can share their perspective? If any of you have been to the US, I'd love to hear your impression our of system.)
Endnote: One thing that I found very strange was seeing Hyundai Santa Fe SUVs all over Seoul. Supposedly, car manufacturers usually change the name of a car when they sell it in a different country, but it was nice to see a little bit of home in Korea!
posted February 22, 2007 01:17 AM in Travel | permalink | Comments (2)
February 20, 2007
Tying-in with digg
I'm starting to tie digg into the posts here. Assuming you like what I write, please digg the stories.
posted February 20, 2007 01:12 PM in Blog Maintenance | permalink | Comments (0)
February 17, 2007
What makes a good (peer) reviewer?
The peer review process is widely criticized for its failings, and many of them are legitimate complaints. But, to paraphrase Churchill, No one pretends that peer review is perfect or all-wise. In fact, peer review is the worst of all systems, except for all the others. Still, peer review is itself only occasionally studied. So, that makes the work of two medical researchers all the more interesting. Callaham and Tercier studied about 3000 reviews of about 1500 manuscripts by about 300 reviewers over a four-year period, and the corresponding quality scores given these reviews by editors.
Our study confirms that there are no easily identifiable types of formal training or experience that predict reviewer performance. Skill in scientific peer review may be as ill defined and hard to impart as is “common sense.” Without a better understanding of those skills, it seems unlikely journals and editors will be successful in systematically improving their selection of reviewers. This inability to predict performance makes it imperative that all but the smallest journals implement routine review ratings systems to routinely monitor the quality of their reviews (and thus the quality of the science they publish).
The other choice results of their study include a consistent negative correlation between the quality of the review and the number of years of experience. That is, younger reviewers write better reviews. To anyone in academia, this should be a truism for obvious reasons. Ironically, service on an Institutional Review Board (IRB; permission from such a board is required to conduct experiments with human subjects) consistently correlated with lower-quality reviews. The caveat here, of course, is that both these and the other factors were only slightly significant.
I've been reviewing for a variety of journals and conferences (across Computer Science, Physics, Biology and Political Science) for a number of years now, and I still find myself trying to write thoughtful, and sometimes lengthy, reviews. I think this is because I honestly believe in the system of peer review, and always appreciate thoughtful reviews myself. Over the years, I've changed some things about how I review papers. I often start earlier now, write a first draft of the review, and then put it down for several days. This lets my thoughts settle on the important points of the paper, rather than on the details that jump out initially. If the paper is good, I try to make small constructive suggestions. If the paper isn't so good, I try to point out the positive aspects, and couch my criticism on firm scientific grounds. In both, I try to see the large context that the results fit into. For some manuscripts, these things are harder than others, particularly if the work seems to have been done hastily, the methodology suspect or poorly described, the conclusions overly broad, etc. My hope is that, once I have a more time-consuming position, I'll have developed some tricks and habits that let me continue to be thoughtful in my reviews, but able to spend less time doing them.
Callaham and Tercier, "The Relationship of Previous Training and Experience of Journal Peer Reviewers to Subsequent Review Quality." PLoS Medicine 4(1): e40 (2007).
Tip to Ars Technica, which has its own take on the study.
posted February 17, 2007 04:47 PM in Simply Academic | permalink | Comments (0)
February 16, 2007
Greatest fear
A scientist's greatest fear...
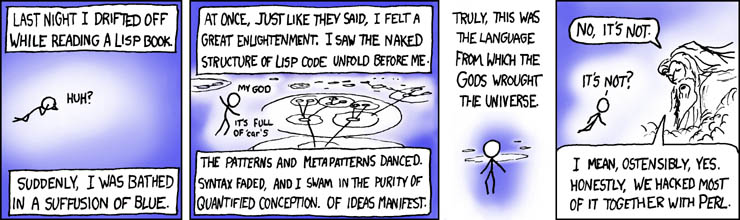
Courtesy of xkcd.
posted February 16, 2007 11:43 AM in Humor | permalink | Comments (0)
February 15, 2007
Fast-modularity made really fast
The other day on the arxiv mailing, a very nice paper appeared (cs.CY/0702048) that optimizes the performance of the fast-modularity algorithm that I worked on with Newman and Moore several years ago. Our algorithm's best running time was O(n log^2 n) on sparse graphs with roughly balanced dendrograms, and we applied it to a large network of about a half million vertices (my implementation is available here).
Shortly after we posted the paper on the arxiv, I began studying its behavior on synthetic networks to understand whether a highly right-skewed distribution of community sizes in the final partition was a natural feature of the real-world network we studied, or whether it was caused by the algorithm itself [1]. I discovered that the distribution probably was not entirely a natural feature because the algorithm almost always produces a few super-communities, i.e., clusters that contain a large fraction of the entire network, even on synthetic networks with no significant community structure. For instance, in the network we analyzed, the top 10 communities account for 87% of the vertices.
Wakita and Tsurumi's paper begins with this observation and then shows that the emergence of these super-communities actually slows the algorithm down considerably, making the running time more like O(n^2) than we would like. They then show that by forcing the algorithm to prefer to merge communities of like sizes - and thus guaranteeing that the dendrogram it constructs will be fairly balanced - the algorithm achieves the bound of essentially linear running time that we proved in our paper. This speed-up yields truly impressive results - they cluster a 4 million node network in about a half an hour - and I certainly hope they make their implementation available to the public. If I have some extra time (unlikely), I may simply modify my own implementation. (Alternatively, if someone would like to make that modification, I'm happy to host their code on this site.)
Community analysis algorithm proposed by Clauset, Newman, and Moore (CNM algorithm) finds community structure in social networks. Unfortunately, CNM algorithm does not scale well and its use is practically limited to networks whose sizes are up to 500,000 nodes. The paper identifies that this inefficiency is caused from merging communities in unbalanced manner. The paper introduces three kinds of metrics (consolidation ratio) to control the process of community analysis trying to balance the sizes of the communities being merged. Three flavors of CNM algorithms are built incorporating those metrics. The proposed techniques are tested using data sets obtained from existing social networking service that hosts 5.5 million users. All the methods exhibit dramatic improvement of execution efficiency in comparison with the original CNM algorithm and shows high scalability. The fastest method processes a network with 1 million nodes in 5 minutes and a network with 4 million nodes in 35 minutes, respectively. Another one processes a network with 500,000 nodes in 50 minutes (7 times faster than the original algorithm), finds community structures that has improved modularity, and scales to a network with 5.5 million.
K. Wakita and T. Tsurumi, "Finding Community Structure in Mega-scale Social Networks." e-print (2007) cs.CY/0702048
Update 14 April 2008: Ken Wakita tells me that their code is now publicly available online.
-----
[1] Like many heuristics, fast-modularity achieves its speed by being highly biased in the set of solutions it considers. See footnote 7 in the previous post. So, without knowing more about why the algorithm behaves in the way it does, a number of things are not clear, e.g., how close to the maximum modularity the partition it returns is, how sensitive its partition is to small perturbations in the input (removing or adding an edge), whether supplementary information such as the dendrogram formed by the sequence of agglomerations is at all meaningful, whether there is an extremely different partitioning with roughly the same modularity, etc. You get the idea. This is why it's wise to be cautious in over-interpreting the output of these biased methods.
posted February 15, 2007 08:23 AM in Computer Science | permalink | Comments (0)
February 12, 2007
BK21 - Workshop on Complex Systems
Recently, I've been in Korea attending the BK21 Workshop on Complex Systems [1]. The workshop itself was held on Saturday at the Korean Advanced Institute for Science and Technology (KAIST) [2] where Hawoong Jeong played the part of an extremely hospitable host. The workshop's billing was on complex systems in general, but mainly the six invited speakers emphasized complex networks [3]. Here are some thoughts that popped out during, or as a result of, some of the lectures, along with some less specific thoughts.
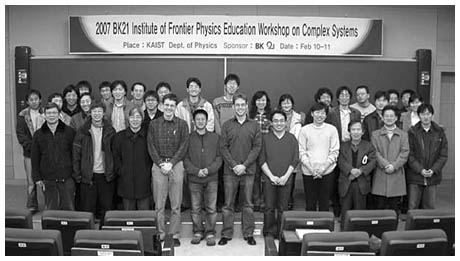
Methods of Statistical Mechanics in Complex Network Theory I've enjoyed hearing Juyong Park (Notre Dame University) describe his work on the Exponential Random Graph (ERG) model of networks before, and enjoyed it again today. The ERG model was originally produced by some sociologists and statisticians, but you wouldn't believe it since it fits so well into the statistical mechanics framework. What makes it useful is that, assuming you properly encode your constraints or dynamics into the system, standard statistical mechanics tools let you understand the average features of the resulting set of random graphs. That is, it's a very nice way to define a set of graphs that have particular kinds of structure, but are otherwise random [4].
Even after chatting afterward with Juyong about ERGs in the context of some questions I have on community structure, I'm still not entirely clear how to use this framework to answer complicated questions. It seems that the encoding step I mention above is highly non-trivial, and that even modestly complicated questions, at best, quickly lead first to intractable equations and then to MCMC simulations. At worst, they lead to a wasted day and a broken pencil. Still, if we can develop more powerful tools for the analysis of (realistic) ERG models, I think there's a lot ERGs can offer the field of network theory. I have a personal little goal now to learn enough about ERGs to demonstrate their utility to my students by making them work through some rudimentary calculations with them.
Functional vs Anatomical Connectivity in Complex Brain Networks
Unfortunately, I was somewhat lost during the talk by Changsong Zhou (University of Potsdam) on the dynamics of neuronal networks. Fortunately, while bopping around Seoul's Insadong area a few days later, I had a chance to have him elucidate networks and neuroscience.
Having seen a number of talks on synchronization in complex networks, I've always been puzzled as to why that is the property people seem to care about for dynamic processes on static networks, and not on their information processing or functional behavior. Changsong clarified - if your basic network unit is an oscillator, then synchronization is how information spreads from one location to another. It still seems to me that, at least in neuronal networks, small groups of neurons should behavior more like functional / information processing units rather than like oscillators, but perhaps I'm still missing something here.
The Hierarchical Organization of Network
Oh, and I gave a talk about my work with Mark Newman and Cris Moore on hierarchical random graphs. This was the third (fourth?) time I've given a version of this talk, and I felt pretty good about the set of results I chose to present, and the balance of emphasis I placed on the methodological details and the big picture.
Also, at the last minute before the talk, and because this venue seemed like a good place to make a little plug for statistical rigor, I threw in some slides about the trouble with fitting power laws wily-nily to the tails of distributions [5]. Pleasantly, I saw a lot of nodding heads in the audience during this segment - as if their owners were unconsciously assenting to their understanding. I'd like to think that by showing how power laws and non-power laws can be statistically (and visually!) indistinguishable, most people will be more careful in the language they use in their conclusions and in their modeling assumptions, but I doubt that some will easily give up the comfort that comes with being able to call everything in complex systems a "power law" [6].
Some less specific thoughts
I think a lot of work in the future (or rather, a lot more than has been done in the past) is going to be on biochemical networks. Since biological systems are extremely complicated, this brings up a problem with the way we reduce them to networks, i.e., what we call nodes and what we call edges. For biologists, almost any abstraction we choose is going to be too simple, since it will throw away important aspects like strange neuronal behavior, non-linear dependence, quantum effects, and spatial issues like protein docking. But at the same time, a model that incorporates all of these things will be too complicated to do solve or simulate. My sympathies here actually lay more with the biologists than the networks people (who are mostly physicists), since I'm more interested in understanding how nature actually works than in telling merely plausible stories about the data (which is, in my opinion, what a lot of physicists who work in complex systems do, including those working on networks).
Still, with systems as messy as biological ones, there's a benefit to being able to give an answer at all, even if it's only approximately correct. The hard part in this approach is to accurately estimate the size of the error bars that go with the estimate. That is, rough answers are sufficient (for now) so long as within that roughness lies the true answer. When we use networks built from uncertain data (most networks), and when we use an algorithm-dependent methodology [7] to analyze that data (most analyses), it's not particularly clear how to accurately estimate the corresponding uncertainty in our conclusions. This is a major problem for empirical network science, and I haven't seen many studies handle it well. The moral here is that so long as we don't properly understand how our methods can mislead us, it's not clear what they're actually telling us!
-----
[1] Although I've known about this event for about a month now, I didn't learn until I arrived what the BK21 part meant. BK21 is an educational development program sponsored by the Korean Ministry of Education (jointly with Cornell and Seoul National University) that emphasizes advanced education in seven areas (information technology, biotech, mechanical engineering, chemical engineering, materials science, physics, and chemistry).
[2] KAIST is located in Daejeon, who's main claim to fame is its focus on science and technology - in 1993, Daejeon hosted a three month long international event called Expo '93. On the way into town yesterday, I saw various remnants of the event, including a hill with hedges grown to spell "EXPO '93".
[3] Given the billing, I suppose it's fitting that someone from SFI was there, although its easy to argue these days that "complex systems" is mainstream science, and that complex networks are one of the more visible aspects of the field. What's surprising is that no one from Cornell (the BK21's US partner) was there.
[4] This is very similar to a maximum entropy argument, which physicists adore and machine-learning people are conflicted about.
[5]
A: This shows a distribution that we might find in the literature - it has a heavy looking tail that could be a power law, and a slow roll-off in the lower tail.
B: If I eyeball the distribution, at about x=1000, it starts looking particularly straight.
C: If I fit a power-law model to the data above this point, it visually appears to follow the data pretty well. In fact, this kind of fit is much better than many of the ones seen in the physics or biology literature since it's to the cumulative distribution function, rather than the raw density function.
D: The rub, of course, is that this distribution doesn't have a power-law tail at all, but is a log-normal distribution. The only reason it looks like it has a power-law tail is that, for a finite-sized sample, the tail always looks like a power law. The more data we have, the farther out in the tail we have to go to find the "power law" and the steeper the estimated slope will be. If we have some theoretical reason to expect the slope to be less than some value, then this fact may save us from mistaking a lognormal for a power law. The bad news is that it can take a lot more data than we typically have for this to help us.
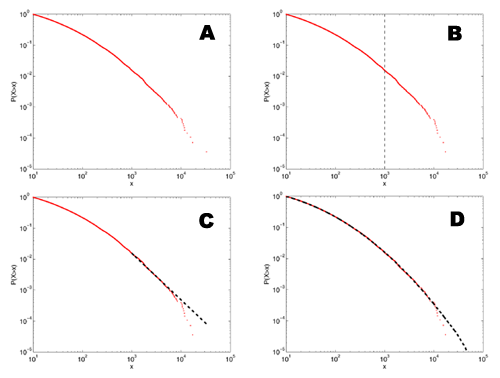
[6] Power laws have been ridiculously accused of being "the signature of complex systems." Although maybe the person who uttered this statement was being pejorative, in which case, and with much chagrin, I'd have to agree.
[7] What do I mean by "algorithm-dependent methodology"? I basically mean a heuristic approach, i.e., a technique that seems reasonable on the surface, but which ignores whether the solution it produces is correct or optimal. Heuristics often produce "pretty good" solutions, and are often necessary when the optimization version of a problem is hard (e.g., NP-hard). The cost of using a heuristic to find a pretty good solution (in contrast to an approximately correct solution, a la approximation algorithms) is typically that it can also produce quite poor solutions on some problems, and, more importantly here, that they are inherently biased in the range of solutions they do produce. This bias is typically directly a function of the assumptions used to build the heuristic, and, because it can be tricky to analyze, is the reason that uncertainty analysis of heuristics is extremely hard, and thus why computer scientists frown upon their use. Basically, the only reason you should ever use a heuristic is if the more honest methods are too slow for the size of your input. In contrast, an algorithm-independent method is one that is at least unbiased in the way it produces solutions, although it still may not produce an optimum one. MCMC and simulated annealing are examples of unbiased heuristics.
posted February 12, 2007 08:14 AM in Complex Systems | permalink | Comments (0)
February 03, 2007
Modernizing Kevin Bacon
Via Mason Porter and Arcane Gazebo comes a pointer to a modernization (geekification?) of the Kevin Bacon game, this time using Wikipedia (and suitably enough, this version has its own wikipedia page). Here's how it works:
- Go to Wikipedia.
- Click the random article link in the sidebar.
- Open a second random article in another tab.
- Try to find a chain of links (as short as possible) starting from the first article that leads to the second.
Many people are fascinated by the fact that these short paths, which is popularly called the "small world phenomenon." This behavior isn't really so amazing, since even purely random graphs have very short paths between arbitrary nodes. What makes the success of these games truly strange is the fact that we can find these short paths using only the information at our current location in the search, and some kind of mental representation of movies and actors / human knowledge and concepts. That is, both the movie-actors network and wikipedia are locally navigable.
The US road system is only partially navigable in this sense. For instance, with only a rudimentary mental map of the country, you could probably get between major cities pretty easily using only the information you see on highway signs. But, cities are not locally navigable because the street signs give you no indication of where to find anything. In order to efficiently navigate them, you either need a global map of the city in hand, or a complex mental map of the city (this is basically what cab drivers do it, but they devote a huge amount of mental space to creating it).
Mason also points me to a tool that will find you the shortest path between two wikipedia articles. However, I'm sure this program isn't finding the path the way a human would. Instead, I'm sure that it's just running a breadth-first search from both pages and returning the path formed when the two trees first touch. What would be more interesting, I think, would be a lexicographic webcrawler that would navigate from the one page to the other using only the text available on its current page (and potentially its history of where it's been), and some kind of simple model of concepts / human knowledge (actually, it would only need a function to tell it whether one concept is closer to its target or not). If such a program could produce chains between random articles that are about as short as those that humans produce, then that would be pretty impressive.
(These questions all relate to the process of navigation on a static network, but an equally important question is the one about how the network produces the structure necessary to be locally navigable in the first place. Although it's a highly idealized and unrealistic model, I humbly point to the results of my first big research project in graduate school as potentially having something to say on this topic.)
posted February 3, 2007 03:09 PM in Complex Systems | permalink | Comments (0)