January 29, 2014
PLOS mandates data availability. Is this a good thing?
The Public Library of Science, aka PLOS, recently announced a new policy on the availability of the data used in all papers published in all PLOS journals. The mandate is simple: all data must be either already publicly available, or be made publicly available before publication, except under certain circumstances [1].
On the face of it, this is fantastic news. It is wholly in line with PLOS’s larger mission of making the outcomes of science open to all, and supports the morally correct goal of making all scientific knowledge accessible to every human. It should also help preserve data for posterity, as apparently a paper’s underlying data becomes increasingly hard to find as the paper ages [2]. But, I think the the truth is more complicated.
PLOS claims that it has always encouraged authors to make their data publicly available, and I imagine that in the vast majority of cases, those data are in fact available. But the policy does change two things: (i) data availability is now a requirement for publication, and (ii) the data are supposed to be deposited in a third-party repository that makes them available without restriction or attached to the paper as supplementary files. The first part ensures that authors who would previously decline or ignore the request for open data must now fall into line. The second part means that a mere promise by the authors to share the data with others is now insufficient. It is the second part where things get complicated, and the first part is meaningless without practical solutions to the second part.
First, the argument for wanting all data associated with scientific papers to be publicly available is a good one, and I think it is also the right one. If scientific papers are in the public domain [3], but the data underlying their results are not, then have we really advanced human knowledge? In fact, it depends on what kind of knowledge the paper is claiming to have produced. If the knowledge is purely conceptual or mathematical, then the important parts are already contained in the paper itself. This situation covers only a smallish fraction of papers. The vast majority report figures, tables or values derived from empirical data, taken from an experiment or an observational study. If those underlying data are not available to others, then the claims in the paper cannot be exactly replicated.
Some people argue that if the data are unavailable, then the claims of a paper cannot be evaluated at all, but that is naive. Sometimes it is crucial to use exactly the same data, for instance, if you are trying to understand whether the authors made a mistake, whether the data are corrupted in some way, or understand a particular method. For these efforts, data availability is clearly helpful.
But, science aspires for general knowledge and understanding, and thus getting results using different data of the same type but which are still consistent with the original claims is actually a larger step forward than simply following exactly the same steps of the original paper. Making all data available may thus have an unintended consequence of reducing the amount of time scientists spend trying to generalize, because it will be easier and faster to simply reuse the existing data rather than work out how to collect a new, slightly different data set or understand the details that went into collecting the original data in the first place. As a result, data availability is likely to increase the rate at which erroneous claims are published. In fields like network science, this kind of data reuse is the norm, and thus gives us some guidance about what kinds of issues other fields might encounter as data sharing becomes more common [4].
Of course, reusing existing data really does have genuine benefits, and in most cases these almost surely outweigh the more nebulous costs I just described. For instance, data availability means that errors can be more quickly identified because we can look at the original data to find them. Science is usually self-correcting anyway, but having the original data available is likely to increase the rate at which erroneous claims are identified and corrected [5]. And, perhaps more importantly, other scientists can use the original data in ways that the original authors did not imagine.
Second, and more critically for PLOS’s new policy, there are practical problems associated with passing research data to a third party for storage. The first problem is deciding who counts as an acceptable third party. If there is any lesson from the Internet age, it is that third parties have a tendency to disappear, in the long run, taking all of their data with them [6]. This is true both for private and public entities, as continued existence depends on continued funding, and continued funding, when that funding comes from users or the government, is a big assumption. For instance, the National Science Foundation is responsible for funding the first few years of many centers and institutes, but NSF makes it a policy to make few or no long-term commitments on the time scales PLOS’s policy assumes. Who then should qualify as a third party? In my mind, there is only one possibility: university libraries, who already have a mandate to preserve knowledge, should be tapped to also store the data associated with the papers they already store. I can think of no other type of entity with as long a time horizon, as stable a funding horizon, and as strong a mandate for doing exactly this thing. PLOS’s policy does not suggest that libraries are an acceptable repository (perhaps because libraries themselves fulfill this role only rarely right now), and only provides the vague guidance that authors should follow the standards of their field and choose a reasonable third party. This kind of statement seems fine for fields with well-developed standards, but it will likely generate enormous confusion in all other fields.
This brings us to another major problem with the storage of research data. Most data sets are small enough to be included as supplementary files associated with the paper, and this seems right and reasonable. But, some data sets are really big, and these pose special problems. For instance, last year I published an open access paper in Scientific Reports that used a 20TB data set of scoring dynamics in a massive online game. Data sets of that scale might be uncommon today, but they still pose a real logistical problem for passing it to a third party for storage and access. If someone requests a copy of the entire data set, who pays for the stack of hard drives required to send it to them? What happens when the third party has hundreds or thousands of such data sets, and receives dozens or more requests per day? These are questions that the scientific community is still trying to answer. Again, PLOS’s policy only pays lip service to this issue, saying that authors should contact PLOS for guidance on “datasets too large for sharing via repositories.”
The final major problem is that not all data should be shared. For instance, data from human-subjects research often includes sensitive information about the participants, e.g., names, addresses, private behavior, etc., and it is unethical to share such data [7]. PLOS’s policy explicitly covers this concern, saying that data on humans must adhere to the existing rules about preserving privacy, etc.
But what about large data sets on human behavior, such as mobile phone call records? These data sets promise to shed new light on human behavior of many kinds and help us understand how entire populations behave, but should these data sets be made publicly available? I am not sure. Research has shown, for instance, that it is not difficult to uniquely distinguish individuals within these large data sets [8] because each of us has distinguishing features to our particular patterns of behavior. Several other papers have demonstrated that portions of these large data sets can be deanonymized, by matching these unique signatures across data sets. For such data sets, the only way to preserve privacy might be to not make the data available. Additionally, many of these really big data sets are collected by private companies, as the byproduct of their business, at a scale that scientists cannot achieve independently. These companies generally only provide access to the data if the data is not shared publicly, because they consider the data to be theirs [9]. If PLOS’s policy were universal, such data sets would seem to become inaccessible to science, and human knowledge would be unable to advance along any lines that require such data [10]. That does not seem like a good outcome.
PLOS does seem to acknowledge this issue, but in a very weak way, saying that “established guidelines” should be followed and privacy should be protected. For proprietary data sets, PLOS only makes this vague statement: “If license agreements apply, authors should note the process necessary for other researchers to obtain a license.” At face value, it would seem to imply that proprietary data sets are allowed, so long as other researchers are free to try to license them themselves, but the devil will be in the details of whether PLOS accepts such instructions or demands additional action as a requirement for publication. I’m not sure what to expect there.
On balance, I like and am excited about PLOS’s new data availability policy. It will certainly add some overhead to finalizing a paper for submission, but it will also make it easier to get data from previously published papers. And, I do think that PLOS put some thought into many of the issues identified above. I also sincerely hope they understand that some flexibility will go a long way in dealing with the practical issues of trying to achieve the ideal of open science, at least until we the community figure out the best way to handle these practical issues.
-----
[1] PLOS's Data Access for the Open Access Literature policy goes into effect 1 March 2014.
[2] See “The availability of Research Data Declines Rapidly with Article Age” by Vines et al. Cell 24(1), 94-97 (2013).
[3] Which, if they are published at a regular “restricted” access journal, they are not.
[4] For instance, there is a popular version of the Zachary Karate Club network that has an error, a single edge is missing, relative to the original paper. Fortunately, no one makes strong claims using this data set, so the error is not terrible, but I wonder how many people in network science know which version of the data set they use.
[5] There are some conditions for self-correction: there must be enough people thinking about a claim that someone might question its accuracy, one of these people must care enough to try to identify the error, and that person must also care enough to correct it, publicly. These circumstances are most common in big and highly competitive fields. Less so in niche fields or areas where only a few experts work.
[6] If you had a profile on Friendster or Myspace, do you know where your data is now?
[7] Federal law already prohibits sharing such sensitive information about human participants in research, and that law surely trumps any policy PLOS might want to put in place. I also expect that PLOS does not mean their policy to encourage the sharing of that sensitive information. That being said, their policy is not clear on what they would want shared in such cases.
[8] And, thus perhaps easier, although not easy, to identify specific individuals.
[9] And the courts seem to agree, with recent rulings deciding that a “database” can be copyrighted.
[10] It is a fair question as to whether alternative approaches to the same questions could be achieved without the proprietary data.
posted January 29, 2014 04:21 AM in Scientifically Speaking | permalink | Comments (3)
July 01, 2013
Small science for the win? Maybe not.
(A small note: with the multiplication of duties and obligations, both at home and at work, writing full-length blog entries has shifted to a lower priority relative to writing full-length scientific articles. Blogging will continue! But selectively so. Paper writing is moving ahead nicely.)
In June of this year, a paper appeared in PLOS ONE [1] that made a quantitative argument for public scientific funding agencies to pursue a "many small" approach in dividing up their budgets. The authors, Jean-Michel Fortin and David Currie (both at the University of Ottawa) argued from data that scientific impact is a decreasing function of total research funding. Thus, if a funding agency wants to maximize impact, it should fund lots of smaller or moderate-sized research grants rather than fund a small number of big grants to large centers or consortia. This paper made a big splash among scientists, especially the large number of us who rely on modest-sized grants to do our kind of science.
Many of us, I think, have felt in our guts that the long-running trend among funding agencies (especially in the US) toward making fewer but bigger grants was poisonous to science [2], but we lacked the data to support or quantify that feeling. Fortin and Currie seemed to provide exactly what we wanted: data showing that big grants are not worth it.
How could such a conclusion be wrong? Despite my sympathy, I'm not convinced they have it right. The problem is not the underlying data, but rather overly simplistic assumptions about how science works and fatal confounding effects.
Fortin and Currie ask whether certain measures of productivity, specifically number of publications or citations, vary as a function of total research funding. They fit simple allometric or power functions [3] to these data and then looked at whether the fitted function was super-linear (increasing return on investment; Big Science = Good), linear (proportional return; Big Science = sum of Small Science) or sub-linear (decreasing return; Big Science = Not Worth It). In every case, they identify a sub-linear relationship, i.e., increasing funding by X% yields less a progressively smaller proportional increase in productivity. In short, a few Big Sciences are less impactful and less productive than many Small Sciences.
But this conclusion only follows from the data if you believe that all scientific questions are equivalent in size and complexity, and that any paper could in principle be written by a research group of any funding level. These beliefs are certainly false, and this implies that the conclusions don't follow from the data.
Here's an illustration of the first problem. In the early days of particle physics, small research groups made significant progress because the equipment was sufficiently simple that a small team could build and operate it, and because the questions of scientific interest were accessible using such equipment. Small Science worked well. But today the situation is different. The questions of scientific interest are largely only accessible with enormously complex machines, run by large research teams supported by large budgets. Big Science is the only way to make progress because the tasks are so complex. Unfortunately, this fact is lost in Fortin and Currie's analysis because their productivity variables do not expose the difficulty of the scientific question being investigated, and are likely inversely correlated with difficulty.
This illustrates the second problem. The cost of answering a question seems largely independent of the number of papers required to describe the answer. There will only be a handful of papers published describing the experiments that discovered the Higgs boson, even though its discovery was possibly one of the costliest physics experiments so far. Furthermore, it is not clear that the few papers produced by a big team should necessarily be highly cited, as citations are produced by the publication of new papers, and if there are only a few research teams working in a particularly complex area, the number of new citations is not independent of the size of the teams.
In fact, by defining "impact" as counts related to paper production [4,5], Fortin and Currie may have inadvertently engineered the conclusion that Small Science will maximize impact. If project complexity correlates positively with grant size and negatively with paper production, then a decreasing return in paper/citation production as a function of grant size is almost sure to appear in any data. So while the empirical results seem correct, they are of ambiguous value. Furthermore, a blind emphasis on funding researchers to solve simple tasks (small grants), to pick all that low-hanging fruit people talk about, seems like an obvious way to maximize paper and citation production because simpler tasks can be solved faster and at lower cost than harder, more complex tasks. You might call this the "Washington approach" to funding, since it kicks the hard problems down the road, to be funded, maybe, in the future.
From my own perspective, I worry about the trend at funding agencies away from small grants. As Fortin and Currie point out, research has shown that grant reviewers are notoriously bad at predicting success [6] (and so are peer reviewers). This fact alone is a sound argument for a major emphasis on funding small projects: making a larger number of bets on who will be successful brings us closer to the expected or background success rate and minimizes the impact of luck (and reduces bias due to collusion effects). A mixed funding strategy is clearly the only viable solution, but what kind of mix is best? How much money should be devoted to big teams, to medium teams, and to small teams? I don't know, and I don't think there is any way to answer that question purely from looking at data. I would hope that the answer is chosen not only by considering things like project complexity, team efficiency, and true scientific impact, but also issues like funding young investigators, maintaining a large and diverse population of researchers, promoting independence among research teams, etc. We'll see what happens.
Update 1 July 2013: On Twitter, Kieron Flanagan asks "can we really imagine no particle physics w/out big sci... or just the kind of particle physics we have today?" To me, the answer seems clear: the more complex the project, the legitimately bigger the funding needs are. That funding manages the complexity, keeps the many moving parts moving together, and ultimately ensures a publication is produced. This kind of coordination is not free, and those dollars don't produce additional publications. Instead, their work allows for any publication at all. Without them, it would be like dividing up 13.25 billion dollars among about 3,000 small teams of scientists and expecting them to find the Higgs, without pooling their resources. So yes, no big science = no complex projects. Modern particle physics requires magnificently complex machines, without which, we'd have some nice mathematical models, but no ability to test them.
To be honest, physics is perhaps too good an example of the utility of big science. The various publicly-supported research centers and institutes, or large grants to individual labs, are a much softer target: if we divided up their large grants into a bunch of smaller ones, could we solve all the same problems and maybe more? Or, could we solve a different set of problems that would more greatly advance our understanding of the world (which is distinct from producing more papers or more citations)? This last question is hard because it means choosing between questions, rather than being more efficient at answering the same questions.
-----
[1] J.-M. Fortin and D.J. Currie, Big Science vs. Little Science: How Scientific Impact Scales with Funding. PLoS ONE 8(6): e65263 (2013).
[2] By imposing large coordination costs on the actual conduct of science, by restricting the range of scientific questions being investigated, by increasing the cost of failure to win a big grant (because the proposals are enormous and require a huge effort to produce), by channeling graduate student training into big projects where only a few individuals actually gain experience being independent researchers, etc. Admittedly, these claims are based on what you might call "domain expertise," rather than hard data.
[3] Functions of the form log y = A*log x + B, which looks like a straight line on a log-log plot. These bivariate functions can be fitted using standard regression techniques. I was happy to see the authors include non-parametric fits to the same data, which seem to mostly agree with the parametric fits.
[4] Citation counts are just a proxy for papers, since only new papers can increase an old paper's count. Also, it's well known that the larger and more active fields (like biomedical research or machine learning) produce more citations (and thus larger citation counts) than smaller, less active fields (like number theory or experimental particle physics). From this perspective, if a funding agency took Fortin and Currie's advice literally, they would cut funding completely for all the "less productive" fields like pure mathematics, economics, computer systems, etc., and devote that money to "more productive" fields like biomedical research and whoever publishes in the International Conference on World Wide Web.
[5] Fortin and Currie do seem to be aware of this problem, but proceed anyway. Their sole caveat is simply thus: "Campbell et al. [12] discuss caveats of the use of bibliometric measures of scientific productivity such as the ones we used."
[6] For instance, see Berg, Nature 489, 203 (2012).
posted July 1, 2013 03:50 PM in Scientifically Speaking | permalink | Comments (0)
August 18, 2012
Wanting to hire
Postdoctoral Fellowship in Study of Networks
Along with Cris Moore, I am looking to hire a postdoc in the area of complex networks and statistical inference. There are two such positions available, one located at the Santa Fe Institute (working with Cris) and one at the University of Colorado Boulder (working with me). Both are funded by a grant from DARPA to investigate the use of generative models to perform statistical inference in complex networks. The larger team includes Mark Newman at the University of Michigan, and there will be ample opportunity to travel and collaborate among the three institutions.
The grant has a particular emphasis on community detection methods, including methods for detecting changes in community structure in dynamic graphs; functional groups that are not merely "clumps" of densely connected nodes; predicting missing links and identifying spurious ones; building on incomplete or noisy information about the network, generalizing from known attributes of nodes and edges to unknown ones; and identifying surprising or anomalous structures or events.
If you are interested in applying, or know someone who has a strong quantitative background in physics, statistics or computer science, see the application information.
The application deadline is 13 January 2013 with an anticipated start date of May or August 2013.
posted August 18, 2012 11:43 AM in Networks | permalink | Comments (0)
May 21, 2012
If it disagrees with experiment, it is wrong
The eloquent Feynman on the essential nature of science. And, he nails it exactly: science is a process of making certain types of guesses about the world around us (what we call "theories" or hypotheses), deriving their consequences (what we call "predictions") and then comparing those consequences with experiment (what we call "validation" or "testing").
Although he doesn't elaborate them, the two transitions around the middle step are, I think, quite crucial.
First, how do we derive the consequences of our theories? It depends, in fact, on what kind of theory it is. Classically, these were mathematical equations and deriving the consequences meant deriving mathematical structures from them, in the form of relationships between variables. Today, with the rise of computational science, theories can be algorithmic and stochastic in nature, and this makes the derivation of their consequences trickier. The degree to which we can derive clean consequences from our theory is a measure of how well we have done in specifying our guess, but not a measure of how likely our guess is to be true. If a theory makes no measurable predictions, i.e., if there are no consequences that can be compared with experiment or if there is no experiment that could disagree with the theory, then it is not a scientific theory. Science is the process of learning about the world around us and measuring our mistakes relative to our expectations is how we learn. Thus, a theory that can make no mistakes teaches us nothing. [1]
Second, how do we compare our predictions with experiment? Here, the answer is clear: using statistics. This part remains true regardless of what kind of theory we have. If the theory predicts that two variables should be related in a certain way, when we measure those variables, we must decide to what degree the data support that relation. This is a subtle point even for experienced scientists because it requires making specific but defensible choices about what constitutes a genuine deviation from the target pattern and how large a deviation is allowed before the theory is discarded (or must be modified) [2]. Choosing an optimistic threshold is what gets many papers into trouble.
For experimental science, designing a better experiment can make it easier to compare predictions with data [3], although complicated experiments necessarily require sensitive comparisons, i.e., statistics. For observational science (which includes astronomy, paleontology, as well as many social and biological questions), we are often stuck with the data we can get rather than the data we want, and here careful statistics is the only path forward. The difficulty is knowing just how small or large a deviation is allowed by your theory. Here again, Feynman has something to say about what is required to be a good scientist:
I'm talking about a specific, extra type of integrity that is not lying, but bending over backwards to show how you are maybe wrong, that you ought to have when acting as a scientist. And this is our responsibility as scientists...
This is a very high standard to meet. But, that is the point. Most people, scientists included, find it difficult to be proven wrong, and Feynman is advocating the active self-infliction of these psychic wounds. I've heard a number of (sometimes quite eminent) scientists argue that it is not their responsibility to disprove their theories, only to show that their theory is plausible. Other people can repeat the experiments and check if it holds under other conditions. At its extreme, this is a very cynical perspective, because it can take a very long time for the self-corrective nature of science to get around to disproving celebrated but ultimately false ideas.
The problem, I think, is that externalizing the validation step in science, i.e., lowering the bar of what qualifies as a "plausible" claim, assumes that other scientists will actually check the work. But that's not really the case since almost all the glory and priority goes to the proposer not the tester. There's little incentive to close that loop. Further, we teach the next generation of scientists to hold themselves to a lower standard of evidence, and this almost surely limits the forward progress of science. The solution is to strive for that high standard of truth, to torture our pet theories until the false ones confess and we are left with the good ideas [4].
-----
[1] Duty obliges me to recommend Mayo's classic book "Error and the Growth of Experimental Knowledge." If you read one book on the role of statistics in science, read this one.
[2] A good historical example of precisely this problem is Millikan's efforts to measure the charge on an electron. The most insightful analysis I know of is the second chapter of Goodstein's "Fact or Fraud". The key point is that Millikan had a very specific and scientifically grounded notion of what constituted a real deviation from his theory, and he used this notion to guide his data collection efforts. Fundamentally, the "controversy" over his results is about this specific question.
[3] I imagine Lord Rutherford would not be pleased with his disciples in high energy physics.
[4] There's a soft middle ground here as to how much should be done by the investigator and how much should be done by others. Fights with referees during the peer-review process often seem to come down to a disagreement about how much and what kind of torture methods should be used before publication. This kind of adversarial relationship with referees is also a problem, I think, as it encourages authors to do what is conventional rather than what is right, and it encourages referees to nitpick or to move the goal posts.
posted May 21, 2012 04:29 PM in Scientifically Speaking | permalink | Comments (1)
March 12, 2012
Friends for the win!
Individuals often compete for personal status, for jobs, for mates, and groups of people, whether formalized as an organization or not, often compete for glory, for dominance, for financial rewards. Although the most visible form of human competition today is probably professional sports, competition via computer games is an increasingly common form of entertainment for regular people [1]. And, most of us play these games socially, playing with or against our friends, but sometimes playing with strangers.
Last year, with Winter Mason, I started a project aimed at understanding the dynamics of complex competitions where decisions are made largely in real-time under large uncertainties at both the player and the game level [2]. The idea was to investigate whether there are general patterns in the way humans compete in these environments, how well we could explain those patterns in terms of exogenous effects like player skill versus endogenous effects due to the rules of the game or the game environment, and whether we could build better tools for either predicting the outcome of competitions or for designing better games overall.
This is all very high minded, but the starting point was much more mundane: the rich and detailed data that Bungie made available for their blockbuster MMOFPS [3] Halo: Reach. It is hard to describe just how Big this data is. When I blogged about this project last summer, Reach players had already produced a staggering 700,000,000 competitions. The number now stands at 958,887,052 (and counting) [4]. Through a web API, Bungie let us download statistics about each and every game of Reach ever played.
These data provided the raw behavioral information about what happens inside the 4-on-4 or 8-on-8, etc. player-versus-player competitions (as well as data on the various player-versus-environment game types). But they didn't tell us much about who was playing. To gather this information, we launched an anonymous web survey in which we asked Reach players to tell us a little about themselves, how they play Halo and who they play it with. The goal was to get real data from real players so that we could understand the role that friendships play in determining success by both the individual and the team in these complex competitive environments.
What we found was cool and surprising in several ways. Friendships, it turns out, are extremely important in shaping not only the performance of individuals, but also their teams. Friendships also shape the way we play the game.
Before diving into the friendships stuff, let's start with some cute results from the survey. First, we had 1191 unique individuals represented in the survey. The distribution of reported ages looks like this:
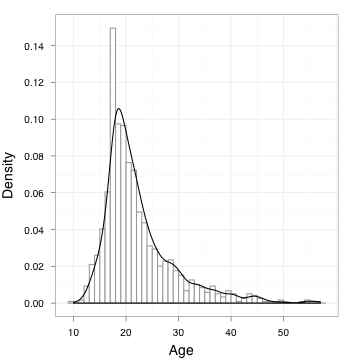
Unsurprisingly, there's a large population of college-aged players, and the median age was 20 years old [5]. This contrasts with the statistics for MMORPGs, where the plurality of players are in their 30s. In our sample, only about 13% of players reported being 30 or older, so Reach is largely played by younger adults. Another interesting point is that the average number of hours per week spent playing video games of all kinds by our participants was 23.3 (3.3 hours per day). This might seem high to non-gamers, but it is slightly lower than the 25.9 reported for MMORPG players and the 27.5 reported in 2007 by the industry association for all gamers [6]. The point is that our survey participants were not unusually serious gamers [7].
By looking at the game histories of each of our participants, we did discover several interesting age-related patterns. First, unlike the stereotype described so vividly in Gus Mastrapa's Wired Magazine article "21st-Century Shooters Are No Country for Old Men," older players are, in fact, better at the game (kills per game) than younger players, and this is especially true for the team-oriented players. Here's the figure:
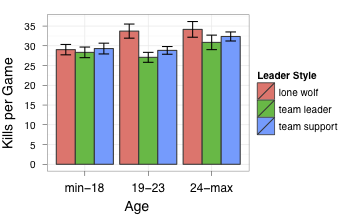
The difference in the number of kills between the age groups is not large, but it is definitely an increase. Also, we define "older players" as being at least 24 years old (the oldest 30% of the population), which may not be what everyone thinks of as being "old". Second, in Reach, it's possible to make an "own goal" by killing a player on your own team. If this happens, it counts as a penalty against the team and may result in the offending player being booted from the competition. What we found is that younger players (at most 17) do this anti-social act much more often than older (18 or older) players:
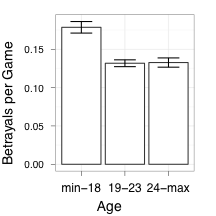
Age does seem to correlate with the preferred style of playing, with younger players (slightly) favoring the "lone wolf" style. This supports one popular perception about younger players, but it turns out that younger players are not actually better at this role than older players (see the previous figure). That all being said, most players (almost 80%) prefer team-oriented roles. That is, Reach players seem to be strongly motivated by the social aspects of the game.
In fact, players seem to structure their activities within the game around opportunities to play with friends. Using fairly simple heuristics like looking at the length of "runs" of two players playing together, we can fairly easily recover the ground-truth labels on friendship we collected. That is, we asked our survey respondents to tell us who among all the other players they played games with were their friends. Accurately guessing these friendship labels turns out not to be a hard task when you have access to the game history alone.
Given that we can identify the friends, we can now ask whether playing with them changes a person's behavior in the game or changes their success. The answers are yes and yes. Two places we see a strong friendship effect are again with the betrayals, and with "assists," where two players cooperate to score a point.
It turn out that friendship matters a lot and encourages strong pro-social (cooperative) behavior within a competition. As the number of friends on a given team increase, the number of "assists," where two players cooperate to score a point, increases while the number betrayals decreases. And, these are large effects, with the assist rate increasing by almost 50% and the betrayal rate decreasing by almost 25% between a team of all friends and a team of all strangers [8].
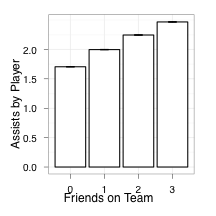
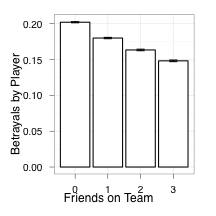
Friendship also has positive effects on both the performance of individual players and the team overall. That is, friends who play together tend to play better together than when they play on their own, even if they play with other good players. This shows up both in the net number of points scored by a player (above and beyond what you'd expect based on skill alone) and the probability of winning, both of which increase with the proportion of friends on the team.
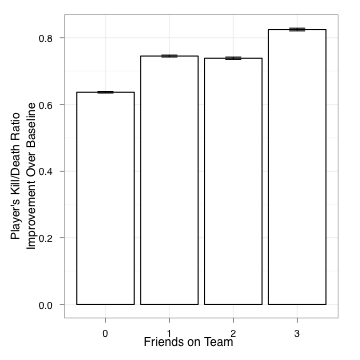

What's important about this "friends for the win" effect is that it appears despite Reach's best efforts to eliminate it. That is, when Reach assembles a new competition from the pool of currently online players, it explicitly tries to balance the teams so that they have equal skill levels. From a game designer perspective, balance is important because a mismatch might lead to a frustrating user experience: a fun competition is a close match. But, the algorithm Reach uses [9] does not control for the synergistic effect that comes from playing with friends, an effect that we see clearly in the data.
It is not really surprising that teams composed of "friends" [10] do better than teams composed of strangers. Friends have likely spent considerable time practicing together and thus may be able to effectively anticipate or adapt to each others' actions or strategies without an explicit need for verbal (and thus time consuming) communication or coordination. Friends may be able to more efficiently divide up multi-person tasks by falling into familiar, and pre-determined and practiced, roles. And, these benefits are precisely what sports teams and military units are aiming to reap when they train together. What's nice is that we see these effects appear even in a virtual environment like Halo, suggesting that they may be fairly universal, and not merely limited to the traditional domains like sports and war, where practicing together has a long tradition.
There's more, of course, but these were some results that seemed particularly interesting. If you'd like to read the rest, there's an arxiv version of the paper available [12].
-----
[1] The entertainment software association claims that in 2011, 72% of American households play computer or video games. It's not clear exactly what they count as "playing" a game (probably something like "did you do it a non-zero number of times over all of 2011"), but it's certainly a very common form of entertainment today. The report I linked to above is filled with made-for-media factoids and you can absorb the entire 13 page document in about 30 seconds of skimming.
[2] This contrasts with classic game theory where generally much more of the game structure is known to the players and decision-making is typically not so highly constrained. That being said, there are some interesting extensions of game theory to similar domains.
[3] World of Warcraft, a social online RPG-style computer game played by millions, is called a massively multiplayer online role playing game, or MMORPG. But Halo: Reach, a social console FPS-style game played by millions, should probably be called a massively multiplayer first person shooter, or MMOFPS.
[4] To give you a sense of the raw popularity of this game, and how quickly its popularity faded, the first 130,000,000 competitions were generated within the first 2 weeks after the game was released on 14 September 2010. That rate of 10M games per day then gradually declined to about 2M games per day by 6 months later. That is an immense amount of Halo.
[5] You'll notice the anomalously large spike at 18. This is almost surely due to under-18 year olds misreporting their age in order to bypass the IRB-required parental consent step in the survey. But, the left-tail of the distribution does not look badly distorted and a large number of under-18s did successfully participate despite the extra consent step.
[6] The MMORPG number is likely fairly accurate. The industry association number may not be.
[7] But they were certainly unusually skilled at Reach relative to the typical player. This is not surprising given that we advertised the study through Halo community forums, where folks with a serious emotional investment in the game tend to hang out.
[8] The fact that the betrayal rate does not go to zero suggests that friendship only goes so far toward encouraging purely pro-social behavior.
[9] It uses the TrueSkill algorithm, which by design assumes that the skill of a team is the sum of the skills of the individual team members.
[10] As a caveat, it's true that we have not been precise about what exactly we mean by friendship here. We did not tell our survey respondents exactly what "friendship" meant, but instead allowed them to decide for themselves who was and wasn't an "online" or "offline" friend. Respondents did use the distinct labels, so they do mean something. That being said, it is possible, even plausible, that people labeled as "online friends" were, in fact, simply familiar individuals with whom they have practice a great deal, rather than some stronger notion. Or, it could indicate a stronger bond. It's not clear.
[11] Winter Mason and Aaron Clauset, "Friends FTW! Friendship and competition in Halo: Reach." Preprint, arxiv:1203.2268 (2012).
posted March 12, 2012 06:48 PM in Scientifically Speaking | permalink | Comments (3)
September 09, 2011
What is the probability of a 9/11-size terrorist attack?
Sunday is the 10-year anniversary of the 9/11 terrorist attacks. As a commemoration of the day, I'm going to investigate answers to a very simple question: what is the probability of a 9/11-size or larger terrorist attack?
There are many ways we could try to answer this question. Most of them don't involve using data, math and computers (my favorite tools), so we will ignore those. Even using quantitative tools, approaches differ based on how strong are the assumptions they make about the social and political processes that generate terrorist attacks. We'll come back to this point throughout the analysis.
Before doing anything new, it's worth repeating something old. For the better part of the past 8 years that I've been studying the large-scale patterns and dynamics of global terrorism (see for instance, here and here), I've emphasized the importance of taking an objective approach to the topic. Terrorist attacks may seem inherently random or capricious or even strategic, but the empirical evidence demonstrates that there are patterns and that these patterns can be understood scientifically. Earthquakes and seismology serves as an illustrative example. Earthquakes are extremely difficult to predict, that is, to say beforehand when, where and how big they will be. And yet, plate tectonics and geophysics tells us a great deal about where and why they happen and the famous Gutenberg-Richter law tells us roughly how often quakes of different sizes occur. That is, we're quite good at estimating the long-time frequencies of earthquakes because larger scales allows us to leverage a lot of empirical and geological data. The cost is that we lose the ability to make specific statements about individual earthquakes, but the advantage is insight into the fundamental patterns and processes.
The same can be done for terrorism. There's now a rich and extensive modern record of terrorist attacks worldwide [1], and there's no reason we can't mine this data for interesting observations about global patterns in the frequencies and severities of terrorist attacks. This is where I started back in 2003 and 2004, when Maxwell Young and I started digging around in global terrorism data. Catastrophic events like 9/11, which (officially) killed 2749 people in New York City, might seem so utterly unique that they must be one-off events. In their particulars, this is almost surely true. But, when we look at how often events of different sizes (number of fatalities) occur in the historical record of 13,407 deadly events worldwide [2], we see something remarkable: their relative frequencies follow a very simple pattern.

The figure shows the fraction of events that killed at least x individuals, where I've divided them into "severe" attacks (10 or more fatalities) and "normal" attacks (less than 10 fatalities). The lions share (92.4%) of these events are of the "normal" type, killing less than 10 individuals, but 7.6% are "severe", killing 10 or more. Long-time readers have likely heard this story before and know where it's going. The solid line on the figure shows the best-fitting power-law distribution for these data [3]. What's remarkable is that 9/11 is very close to the curve, suggesting that statistically speaking, it is not an outlier at all.
A first estimate: In 2009, the Department of Defense received the results of a commissioned report on "rare events", with a particular emphasis on large terrorist attacks. In section 3, the report walks us through a simple calculation of the probability of a 9/11-sized attack or larger, based on my power-law model. It concludes that there was a 23% chance of an event that killed 2749 or more between 1968 and 2006. [4] The most notable thing about this calculation is that its magnitude makes it clear that 9/11 should not be considered a statistical outlier on the basis of its severity.
How we can we do better: Although probably in the right ballpark, the DoD estimate makes several strong assumptions. First, it assumes that the power-law model holds over the entire range of severities (that is x>0). Second, it assumes that the model I published in 2005 is perfectly accurate, meaning both the parameter estimates and the functional form. Third, it assumes that events are generated independently by a stationary process, meaning that the production rate of events over time has not changed nor has the underlying social or political processes that determine the frequency or severity of events. We can improve our estimates by improving on these assumptions.
A second estimate: The first assumption is the easiest to fix. Empirically, 7.6% of events are "severe", killing at least 10 people. But, the power-law model assumed by the DoD report predicts that only 4.2% of events are severe. This means that the DoD model is underestimating the probability of a 9/11-sized event, that is, the 23% estimate is too low. We can correct this difference by using a piecewise model: with probability 0.076 we generate a "severe" event whose size is given by a power-law that starts at x=10; otherwise we generate a "normal" event by choosing a severity from the empirical distribution for 0 < x < 10 . [5] Walking through the same calculations as before, this yields an improved estimate of a 32.6% chance of a 9/11-sized or larger event between 1968-2008.
A third estimate: The second assumption is also not hard to improve on. Because our power-law model is estimated from finite empirical data, we cannot know the alpha parameter perfectly. Our uncertainty in alpha should propagate through to our estimate of the probability of catastrophic events. A simple way to capture this uncertainty is to use a computational bootstrap resampling procedure to generate many synthetic data sets like our empirical one. Estimating the alpha parameter for each of these yields an ensemble of models that represents our uncertainty in the model specification that comes from the empirical data.
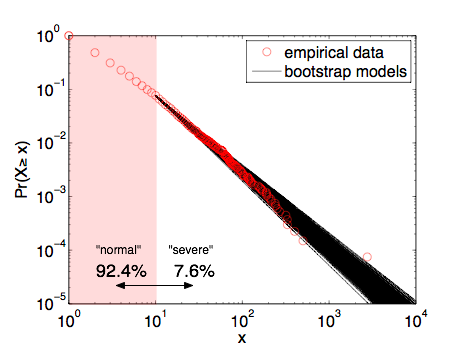
This figure overlays 1000 of these bootstrap models, showing that they do make slightly different estimates of the probability of 9/11-sized events or larger. As a sanity check, we find that the mean of these bootstrap parameters is alpha=2.397 with a standard deviation of 0.043 (quite close to the 2.4+/-0.1 value I published in 2009 [6]). Continuing with the simulation approach, we can numerically estimate the probability of a 9/11-sized or larger event by drawing synthetic data sets from the models in the ensemble and then asking what fraction of those events are 9/11-sized or larger. Using 10,000 repetitions yields an improved estimate of 40.3%.
Some perspective: Having now gone through three calculations, it's notable that the probability of a 9/11-sized or larger event has almost doubled as we've improved our estimates. There are still additional improvements we could do, however, and these might push the number back down. For instance, although the power-law model is a statistically plausible model of the frequency-severity data, it's not the only such model. Alternatives like the stretched exponential or the log-normal decay faster than the power law, and if we were to add them to the ensemble of models in our simulation, they would likely yield 9/11-sized or larger events with lower frequencies and thus likely pull the probability estimate down somewhat. [7]
Peering into the future: Showing that catastrophic terrorist attacks like 9/11 are not in fact statistical outliers given the sheer magnitude and diversity of terrorist attacks worldwide over the past 40 years is all well and good, you say. But, what about the future? In principle, these same models could be easily used to make such an estimate. The critical piece of information for doing so, however, is a clear estimate of the trend in the number of events each year. The larger that number, the greater the risk under these models of severe events. That is, under a fixed model like this, the probability of catastrophic events is directly related to the overall level of terrorism worldwide. Let's look at the data.
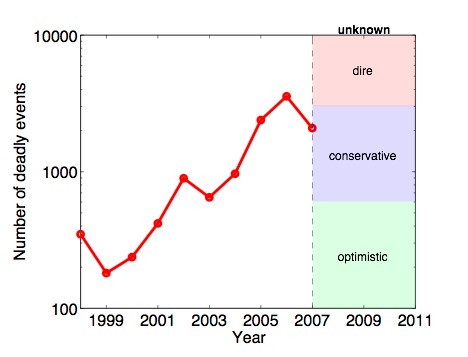
Do you see a trend here? It's difficult to say, especially with the changing nature of the conflicts in Iraq and Afghanistan, where many of the terrorist attacks of the past 8 years have been concentrated. It seems unlikely, however, that we will return to the 2001 levels (200-400 events per year; the optimist's scenario). A dire forecast would have the level continue to increase toward a scary 10,000 events per year. A more conservative forecast, however, would have the rate continue as-is relative to 2007 (the last full year for which I have data), or maybe even decrease to roughly 1000 events per year. Using our estimates from above, 1000 events overall would generate about 75 "severe" events (more than 10 fatalities) per year. Plugging this number into our computational model above (third estimate approach), we get an estimate of roughly a 3% chance of a 9/11-sized or larger attack each year, or about a 30% chance over the next decade. Not a certainty by any means, but significantly greater than is comfortable. Notably, this probability is in the same ballpark for our estimates for the past 40 years, which goes to show that the overall level of terrorism worldwide has increased dramatically during those decades.
It bears repeating that this forecast is only as good as the models on which it is based, and there are many things we still don't know about the underlying social and political processes that generate events at the global scale. (In contrast to the models the National Hurricane Center uses to make hurricane track forecasts.) Our estimates for terrorism all assume a homogeneous and stationary process where event severities are independent random variables, but we would be foolish to believe that these assumptions are true in the strong sense. Technology, culture, international relations, democratic movements, urban planning, national security, etc. are all poorly understood and highly non-stationary processes that could change the underlying dynamics in the future, making our historical models less reliable than we would like. So, take these estimates for what they are, calculations and computations using reasonable but potentially wrong assumptions based on the best historical data and statistical models currently available. In that sense, it's remarkable that these models do as well as they do in making fairly accurate long-term probabilistic estimates, and it seems entirely reasonable to believe that better estimates can be had with better, more detailed models and data.
Update 9 Sept. 2011: In related news, there's a piece in the Boston Globe (free registration required) about the impact 9/11 had on what questions scientists investigate that discusses some of my work.
-----
[1] Estimates differ between databases, but the number of domestic or international terrorist attacks worldwide between 1968 and 2011 is somewhere in the vicinity of 50,000-100,000.
[2] The historical record here is my copy of the National Memorial Institute for the Prevention of Terrorism (MIPT) Terrorism Knowledge Base, which stores detailed information on 36,018 terrorist attacks worldwide from 1968 to 2008. Sadly, the Department of Homeland Security pulled the plug on the MIPT data collection effort a few years ago. The best remaining data collection effort is the one run by the University of Maryland's National Consortium for the Study of Terrorism and Response to Terrorism (START) program.
[3] For newer readers: a power-law distribution is a funny kind of probability distribution function. Power laws pop up all over the place in complex social and biological systems. If you'd like an example of how weird power-law distributed quantities can be, I highly recommend Clive Crook's 2006 piece in The Atlantic title "The Height of Inequality" in which he considers what the world would look like if human height were distributed as unequally as human wealth (a quantity that is very roughly power-law-like).
[4] If you're curious, here's how they did it. First, they took the power-law model and the parameter value I estimated (alpha=2.38) and computed the model's complementary cumulative distribution function. The "ccdf" tells you the probability of observing an event at least as large as x, for any choice of x. Plugging in x=2749 yields p=0.0000282. This gives the probability of any single event being 9/11-sized or larger. The report was using an older, smaller data set with N=9101 deadly events worldwide. The expected number of these events 9/11-sized or larger is then p*N=0.257. Finally, if events are independent then the probability that we observe at least one event 9/11-sized or larger in N trials is 1-exp(-p*N)=0.226. Thus, about a 23% chance.
[5] This changes the calculations only slightly. Using alpha=2.4 (the estimate I published in 2009), given that a "severe" event happens, the probability that it is at least as large as 9/11 is p=0.00038473 and there were only N=1024 of them from 1968-2008. Note that the probability is about a factor of 10 larger than the DoD estimate while the number of "severe" events is about a factor of 10 smaller, which implies that we should get a probability estimate close to theirs.
[6] In "Power-law distributions in empirical data," SIAM Review 51(4), 661-703 (2009), with Cosma Shalizi and Mark Newman.
[7] This improvement is mildly non-trivial, so perhaps too much effort for an already long-winded blog entry.
posted September 9, 2011 02:17 PM in Terrorism | permalink | Comments (1)
July 06, 2011
You're doing science with what?
I didn't get much research finished during my first year as a university professor [1], but I did start at least one new project. This project is new and exciting and fresh! Plus, it's my first genuine foray into social science, meaning that I had to get IRB approval in order to do it and that my main collaborator on the project is a psychologist Winter Mason, at Yahoo! Research.
What is this project, you say? It's The Halo: Reach Project. Yes, you read that correctly: it's a science project about a video game [2]. Well, almost. In fact, the project is only tangentially related to the game itself. Like other scientific studies involving video games [3], the game is just a means to a scientific end, which in this case is to study certain types of human behavior "in the wild." In this case, we're interested in team dynamics in competitive environments, and Halo: Reach happens to be a very large-scale experimental platform about precisely that, with 688,375,259 (and counting) games and roughly 1,000,000 regular players. The scale of this data alone is interesting, but so too is the possibility of using it to learn something about the dynamics of competition [4].
Competition between teams is not just a fundamental feature of professional sports, but also a feature of the capitalist economy, with firms being teams, as well as modern political life and perhaps even violent conflicts like civil or international wars. Despite the generality of the idea, however, it is not a forgone conclusion that the dynamics of competition with Halo: Reach will tell us anything at all about competition in these other environments. But, the joy of being a professional scientist is the joy of being able to find out. Plus, I get to enjoy the odd looks people give me when they ask the perennial question "What are you working on now?" and I reply something like "I'm studying Halo, the video game."
If you play Halo: Reach, please help us out by taking the web survey. If you don't play but want to help, please help us spread the word [5].
-----
[1] Which reminds me, I should blog about how that went. Another day.
[2] Which are now classified as protected speech under the 1st Amendment by the SCOTUS.
[3] In fact, most of the scientific studies about the behavior of people inside game environments (in contrast with studies about behavior outside game environments), like my good friend Nick Yee's studies of Everquest and World of Warcraft, have focused on the MMORPG world. Halo: Reach can be thought of as a MMO First-Person Shooter (MMOFPS), and these have received comparatively less study.
[4] So in a sense, this is another example of a computational social science project.
[5] For instance, you could tweet about it, blog about it or even send the following message to (e.g., your undergrad or graduate) mailing lists:
Subject: Scientists studying team dynamics looking for Halo: Reach volunteers.
Do you play Halo: Reach?
The University of Colorado at Boulder is conducting a scientific study of Halo: Reach players on team dynamics within the game. The idea is to sort out, for instance, what role training alone has on team play, what impact playing with regular teammates makes on your (and their) performance, what role age plays in playing style, etc. To answer these and other questions, they need your help.
Here's how it works: you simply answer some questions about yourself (demographics) and about the way you play Halo: Reach and which gamertags you play with. The whole web survey takes about 10 minutes total. After that, if you're interested, they'll show you how your Halo: Reach service record stacks up against the universe of other Reach players.
Sign me up: https://www.cs.colorado.edu/haloreach/
More about the project: https://www.cs.colorado.edu/haloreach/about
posted July 6, 2011 03:32 PM in Scientifically Speaking | permalink | Comments (9)
April 16, 2011
Storm, by Tim Minchin
Tim's 9-minute beat poem sums things up probably better than I've ever done when I've played the same role.
Tip to Cris Moore.
posted April 16, 2011 04:09 PM in Scientifically Speaking | permalink | Comments (0)
February 13, 2011
Proximate vs. Ultimate Causes
Jon Wilkins, a former colleague of mine at the Santa Fe Institute, has discovered a new talent: writing web comics. [1] Using the clever comic-strip generator framework provided by Strip Generator, he's begun producing comics that cleverly explain important ideas in evolutionary biology. In his latest comic, Jon explains the difference between proximate and ultimate causes, a distinction the US media seems unaware of, as evidenced by their irritating fawning over the role of social media like Twitter, Facebook, etc. in the recent popular uprisings in the Middle East. Please keep them coming, Jon.
-----
[1] Jon has several other talents worth mentioning: he's an evolutionary biologist, an award-winning poet, a consumer of macroeconomic quantities of coffee as well as a genuinely nice guy.
posted February 13, 2011 04:58 PM in Scientifically Speaking | permalink | Comments (0)
February 02, 2011
Whither computational paleobiology?
This week I'm in Washington DC, hanging out at the Smithsonian (aka the National Museum of Natural History) with paleontologists, paleobiologists, paleobotanists, palaeoentomologist, geochronographers, geochemists, macrostratigraphers and other types of rock scientists. The meeting is an NSF-sponsored workshop on the Deep Time Earth-Life Observatory Network (DETELON) project, which is a community effort to persuade NSF to fund large-ish interdisciplinary groups of scientists exploring questions about earth-life system dynamics and processes using data drawn from deep time (i.e., the fossil record).
One of the motivations here is the possibility to draw many different skill sets and data sources together in a synergistic way to shed new light on fundamental questions about how the biosphere interacts with (i.e., drives and is driven by) geological processes and how it works at the large scale, potentially in ways that might be relevant to understanding the changing biosphere today. My role in all this is to represent the potential of mathematical and computational modeling, especially of biotic processes.
I like this idea. Paleobiology is a wonderfully fascinating field, not just because it involves studying fossils (and dinosaurs; who doesn't love dinosaurs?), but also because it's a field rich with interesting puzzles. Surprisingly, the fossil record, or rather, the geological record (which includes things that are not strictly fossils), is incredibly rich, and the paleo folks have become very sophisticated in extracting information from it. Like many other sciences, they're now experiencing a data glut, brought on by the combination of several hundred years of hard work, a large community of data collectors and museums, along with computers and other modern technologies that make extracting, measuring and storing the data easier to do at scale. And, they're building large, central data repositories (for instance, this one and this one), which span the entire globe and all of time. What's lacking in many ways is the set of tools that can allow the field to automatically extract knowledge and build models around these big data bases in novel ways.
Enter "computational paleobiology", which draws on the tools and methods of computer science (and statistics and machine learning and physics) and the questions of paleobiology, ecology, macroevolution, etc. At this point, there aren't many people who would call themselves a computational paleobiologist (or computational paleo-anything), which is unfortunate. But, if you think evolution and fossils are cool, if you like data with interesting stories, if you like developing clever algorithms for hard inference problems or if you like developing mathematical or computational models for complex systems, if you like having an impact on real scientific questions, and if you like a wide-open field, then I think this might be the field for you.
posted February 2, 2011 08:26 PM in Interdisciplinarity | permalink | Comments (6)
January 08, 2011
Labyrinths, caves and ... power laws?
Late last year, I saw a few references on Facebook to some beautiful pictures of big caves in Vietnam. I didn't realize until later that this wasn't just any big cave, but probably the largest cave in the world Hang Son Doong, which was first surveyed only two years ago. National Geographic did a short article about it and its photo gallery was the source of the beautiful images.




From the article:
In the spring of 2009, [Jonathan] Sims was a member of the first expedition to enter Hang Son Doong, or "mountain river cave," in a remote part of central Vietnam. Hidden in rugged Phong Nha-Ke Bang National Park near the border with Laos, the cave is part of a network of 150 or so caves, many still not surveyed, in the Annamite Mountains. During the first expedition, the team explored two and a half miles of Hang Son Doong before a 200-foot wall of muddy calcite stopped them. They named it the Great Wall of Vietnam. Above it they could make out an open space and traces of light, but they had no idea what lay on the other side. A year later, they have returned —- seven hard-core British cavers, a few scientists, and a crew of porters -— to climb the wall, if they can, measure the passage, and push on, if possible, all the way to the end of the cave.
... An enormous shaft of sunlight plunges into the cave like a waterfall. The hole in the ceiling through which the light cascades is unbelievably large, at least 300 feet across. The light, penetrating deep into the cave, reveals for the first time the mind-blowing proportions of Hang Son Doong. The passage is perhaps 300 feet wide, the ceiling nearly 800 feet tall: room enough for an entire New York City block of 40-story buildings. There are actually wispy clouds up near the ceiling.
The light beaming from above reveals a tower of calcite on the cave floor that is more than 200 feet tall, smothered by ferns, palms, and other jungle plants. Stalactites hang around the edges of the massive skylight like petrified icicles. Vines dangle hundreds of feet from the surface; swifts are diving and cutting in the brilliant column of sunshine. The tableau could have been created by an artist imagining how the world looked millions of years ago.
This cave is truly mind-boggling in size, as the pictures above make clear. Many caves have their own ecosystems, powered either by nutrients flowing in from the surface (sometimes carried by animals like bats that use caves as nesting grounds or garbage dumps) or coming out of the earth itself. It will be interesting to see what weird wonders scientists find in these caves. The third picture shows a forest in the cave where a roof collapse lets light (and life) in.
Coincidentally, only a few weeks before these appeared, I received an email from a gentleman in Norway curious about power-law distributions and caves. As it turns out, an avid American caver named Bob Gulden maintains a global database of cave size information, including data on the longest caves in the world, the deepest caves in the world, and the same for the United States, among other things [1]. The Hang Son Doong cave doesn't show up on Bob's list of the big caves likely because it is not an extremely long or deep cave [2]. Rather, it simply has some of the largest open spaces and towering features. The longest cave in the world is the Mammoth Cave System in Kentucky at 390 miles in length.
The question I was posed by my emailer was basically, does the distribution of large cave lengths that Bob has tabulated follow a power-law distribution? And if so, what does that tell us about the number of shorter caves out there waiting to be discovered? In meters, the top three cave lengths are 627,644, 241,595 and 230,140, while the 300th longest cave is only 15,144 meters long. That huge variance immediately tells us that we're dealing with a heavy-tailed distribution. Being more sophisticated, and doing the statistics properly, here's what the distribution looks like along with a maximum likelihood power-law fit.
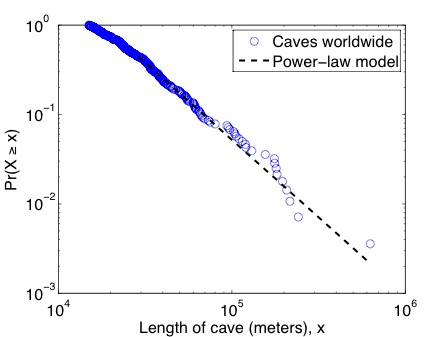
This is not a bad looking fit. The maximum likelihood exponent is alpha = 2.73 +/- 0.19 for the 125 caves 28,968m or longer. Most notably, the p-value for the fitted model is p = 0.38 +/- 0.03, implying that the power-law hypothesis is at least a plausible explanation for the length distribution of the very longest caves in the world [3]. Who would have guessed?
What this means for less long caves is more ambiguous. From a purely statistical point of view, more than half of the 280 caves I analyzed [4] are excluded from the power-law region. This could be a sampling artifact in the sense that shorter caves are probably harder to find than longer ones and thus they are under-represented in our data collection relative to their true frequency. In fact, given that even long caves are quite difficult to find and accurately survey, especially if they are in remote places, have few surface openings, are under water or have many tight squeezes, it seems highly likely that there is also under-reporting and possibly even mis-measurement at the upper end of the distribution, although how much seems difficult to say [5].
If these data are representative of the underlying distribution of worldwide cave lengths, then the non-power-law behavior at the short end could also indicate a mixture of distributional structures, with the power-law-like behavior only applying for the very longest of caves. This then begs the question: what kind of geological or mixture of geological processes produce such a heavy-tailed distribution of cave lengths, and might there be different processes controlling the creation of long versus short caves? Trivial hypotheses based on equating this distribution with fracture length distributions, earthquake sizes, or even the self-similarity of erosion networks might work, but they also might not. For instance, the formation process may depend on the type of rock the cave is in, and rock type does seem to vary among the top 300 longest caves.
For any mechanistic explanation, there's another interesting unknown here, which is how long do caves typically last? For instance, I imagine some kind of geological shuffling process that occasionally creates pockets of air while moving large amounts of rock around. Through additional shuffling, these proto-caves can occasionally become connected and the probability of connecting many pockets together to form a very long cave must depend on the time scale of cave lifetimes. That is, the processes that create the pockets can also eliminate them or divide them. Intuitively, very long caves should be fairly short-lived because they have many more places where a geological shift could disconnect them. Were such events independent, this would yield an effective Poisson process which is clearly not going to produce extremely long caves, although mixtures of these across different time or length scales might do the trick. Other processes may also end up being important, for instance, erosion, which can quickly carve pockets out of certain types of rock, the proximity to a tectonic plate boundary, where geological shuffling is most common, etc. Adding these effects to the simple probabilistic model outlined above could be quite complicated, but would also give us some idea of where to look for new big caves, which would be cool.
-----
[1] Unsurprisingly, there's a whole National Speleological Society devoted to caving, which even publishes a scientific journal called the Journal of Cave and Karst Studies. An earlier version of Bob's list, listing only the top 53 caves, appeared in JCKS in 2006.
[2] For the "world's longest" list, Bob tracks only caves with length greater than 15000 meters, or about 9.3 miles in length. But see footnote [4].
[3] Said more precisely, the p-value is sufficiently large that we cannot reject the hypothesis that the fluctuations (deviations) observed between the fitted model and the data are simply iid sampling noise.
[4] Bob updates his list pretty regularly, so these numbers are from the list as of today, while the data I pulled and analyzed was from 18 December 2010.
[5] There are other lists of long caves, for instance, one formerly maintained by Eric Madelaine in France (here). Eric's list seems to have been dormant since 2006 but has a total of 1628 entries including caves as short as 3000 meters. Additionally, some measurements differ from Bob's list. Here, the maximum likelihood power-law region is much larger, extending down to xmin = 3,950m and including 1214 caves, the tail heavier, with alpha = 2.27 +/- 0.06, but the power-law hypothesis is highly unlikely, with p = 0.05 +/- 0.03. What this means, of course, is still fairly unclear, as many of the same sampling and measurement issues described above apply here just as forcefully. What I think these analyses do tell us is that cave length is a fairly non-trivial variable, and that there are likely interesting geological processes shaping its clear heavy-tailed structure. Whether it's a nice power law or not is really beside the point, and real progress would take the form of a mechanistic hypothesis that can be tested.
Update 8 January 2011: As an afterthought, given that the larger data set with the wider "power-law region" in [5] yields a much heavier value of alpha relative to Bob's smaller data set (alpha = 2.27 vs. 2.73), and the former is largely a superset of the latter, it seems to me that the power-law-like structure is probably a sampling artifact. Thus, a much larger data set (n>10,000) seems likely to show significant curvature throughout most of the range of cave lengths (which would explain why the exponent increased from the smaller xmin to the larger one) or perhaps simply statistically significant deviations relative to the simple power-law model. The extreme upper tail (Bob's data) would still hint at power-law structure, but this might merely indicate some geologically induced finite-size limit.
posted January 8, 2011 11:54 AM in Scientifically Speaking | permalink | Comments (6)
December 14, 2010
Statistical Analysis of Terrorism
Yesterday, my work on global statistical patterns in terrorism [1] was featured in a long article in the magazine Miller-McCune called The Physics of Terrorism, written by Michael Haederle [2].
Much of the article focuses on the weird empirical fact that the frequency of severe terrorist attacks is well described by a power-law distribution [3,4], although it also discusses my work on robust patterns of behavior in terrorist groups, for instance, showing that they typically increase the frequency of their attacks as they get older (and bigger and more experienced), and moreover that they do it in a highly predictable way. There are several points I like most about Michael's article. First, he emphasizes that these patterns are not just nice statistical descriptions of things we already know, but rather they show that some things we thought were fundamentally different and unpredictable are actually related and that we can learn something about large but rare events by studying the more common smaller events. And second, he emphasizes the fact that these patterns can actually be used to make quantitative, model-based statistical forecasts about the future, something current methods in counter-terrorism struggle with.
Of course, there's a tremendous amount of hard-nosed scientific work that remains to be done to develop these empirical observations into practical tools, and I think it's important to recognize that they will not be a silver bullet for counter-terrorism, but they do show us that much more can be done here than has been traditionally believed and that there are potentially fundamental constraints on terrorism that could serve as leverage points if exploited appropriately. That is, so to speak, there's a forest out there that we've been missing by focusing only on the trees, and that thinking about forests as a whole can in fact help us understand some things about the behavior of trees. I don't think studying large-scale statistical patterns in terrorism or other kinds of human conflict takes away from the important work of studying individual conflicts, but I do think it adds quite a bit to our understanding overall, especially if we want to think about the long-term. How does that saying go again? Oh right, "those who do not learn from history are doomed to repeat it" (George Santayana, 1863-1952) [5].
The Miller-McCune article is fairly long, but here are a few good excerpts that capture the points pretty well:
Last summer, physicist Aaron Clauset was telling a group of undergraduates who were touring the Santa Fe Institute about the unexpected mathematical symmetries he had found while studying global terrorist attacks over the past four decades. Their professor made a comment that brought Clauset up short. "He was surprised that I could think about such a morbid topic in such a dry, scientific way," Clauset recalls. "And I hadn’t even thought about that. It was just … I think in some ways, in order to do this, you have to separate yourself from the emotional aspects of it."
But it is his terrorism research that seems to be getting Clauset the most attention these days. He is one of a handful of U.S. and European scientists searching for universal patterns hidden in human conflicts — patterns that might one day allow them to predict long-term threats. Rather than study historical grievances, violent ideologies and social networks the way most counterterrorism researchers do, Clauset and his colleagues disregard the unique traits of terrorist groups and focus entirely on outcomes — the violence they commit.
“When you start averaging over the differences, you see there are patterns in the way terrorists’ campaigns progress and the frequency and severity of the attacks,” he says. “This gives you hope that terrorism is understandable from a scientific perspective.” The research is no mere academic exercise. Clauset hopes, for example, that his work will enable predictions of when terrorists might get their hands on a nuclear, biological or chemical weapon — and when they might use it.
It is a bird’s-eye view, a strategic vision — a bit blurry in its details — rather than a tactical one. As legions of counterinsurgency analysts and operatives are trying, 24-style, to avert the next strike by al-Qaeda or the Taliban, Clauset’s method is unlikely to predict exactly where or when an attack might occur. Instead, he deals in probabilities that unfold over months, years and decades — probability calculations that nevertheless could help government agencies make crucial decisions about how to allocate resources to prevent big attacks or deal with their fallout.
-----
[1] Here are the relevant scientific papers:
On the Frequency of Severe Terrorist Attacks, by A. Clauset, M. Young and K. S. Gledistch. Journal of Conflict Resolution 51(1), 58 - 88 (2007).
Power-law distributions in empirical data, by A. Clauset, C. R. Shalizi and M. E. J. Newman. SIAM Review 51(4), 661-703 (2009).
A generalized aggregation-disintegration model for the frequency of severe terrorist attacks, by A. Clauset and F. W. Wiegel. Journal of Conflict Resolution 54(1), 179-197 (2010).
The Strategic Calculus of Terrorism: Substitution and Competition in the Israel-Palestine Conflict, by A. Clauset, L. Heger, M. Young and K. S. Gleditsch Cooperation & Conflict 45(1), 6-33 (2010).
The developmental dynamics of terrorist organizations, by A. Clauset and K. S. Gleditsch. arxiv:0906.3287 (2009).
A novel explanation of the power-law form of the frequency of severe terrorist events: Reply to Saperstein, by A. Clauset, M. Young and K.S. Gleditsch. Forthcoming in Peace Economics, Peace Science and Public Policy.
[2] It was also slashdotted.
[3] If you're unfamiliar with power-law distributions, here's a brief explanation of how they're weird, taken from my 2010 article in JCR:
What distinguishes a power-law distribution from the more familiar Normal distribution is its heavy tail. That is, in a power law, there is a non-trivial amount of weight far from the distribution's center. This feature, in turn, implies that events orders of magnitude larger (or smaller) than the mean are relatively common. The latter point is particularly true when compared to a Normal distribution, where there is essentially no weight far from the mean.
Although there are many distributions that exhibit heavy tails, the power law is special and exhibits a straight line with slope alpha on doubly-logarithmic axes. (Note that some data being straight on log-log axes is a necessary, but not a sufficient condition of being power-law distributed.)
Power-law distributed quantities are not uncommon, and many characterize the distribution of familiar quantities. For instance, consider the populations of the 600 largest cities in the United States (from the 2000 Census). Among these, the average population is only x-bar =165,719, and metropolises like New York City and Los Angles seem to be "outliers" relative to this size. One clue that city sizes are not well explained by a Normal distribution is that the sample standard deviation sigma = 410,730 is significantly larger than the sample mean. Indeed, if we modeled the data in this way, we would expect to see 1.8 times fewer cities at least as large as Albuquerque (population 448,607) than we actually do. Further, because it is more than a dozen standard deviations above the mean, we would never expect to see a city as large as New York City (population 8,008,278), and largest we expect would be Indianapolis (population 781,870).
As a more whimsical second example, consider a world where the heights of Americans were distributed as a power law, with approximately the same average as the true distribution (which is convincingly Normal when certain exogenous factors are controlled). In this case, we would expect nearly 60,000 individuals to be as tall as the tallest adult male on record, at 2.72 meters. Further, we would expect ridiculous facts such as 10,000 individuals being as tall as an adult male giraffe, one individual as tall as the Empire State Building (381 meters), and 180 million diminutive individuals standing a mere 17 cm tall. In fact, this same analogy was recently used to describe the counter-intuitive nature of the extreme inequality in the wealth distribution in the United States, whose upper tail is often said to follow a power law.
Although much more can be said about power laws, we hope that the curious reader takes away a few basic facts from this brief introduction. First, heavy-tailed distributions do not conform to our expectations of a linear, or normally distributed, world. As such, the average value of a power law is not representative of the entire distribution, and events orders of magnitude larger than the mean are, in fact, relatively common. Second, the scaling property of power laws implies that, at least statistically, there is no qualitative difference between small, medium and extremely large events, as they are all succinctly described by a very simple statistical relationship.
[4] In some circles, power-law distributions have a bad reputation, which is not entirely undeserved given the way some scientists have claimed to find them everywhere they look. In this case, though, the data really do seem to follow a power-law distribution, even when you do the statistics properly. That is, the power-law claim is not just a crude approximation, but a bona fide and precise hypothesis that passes a fairly harsh statistical test.
[5] Also quoted as "Those who cannot remember the past are condemned to repeat their mistakes".
posted December 14, 2010 10:39 AM in Scientifically Speaking | permalink | Comments (6)
November 05, 2010
Nathan Explains Science, welcome to the blogosphere!
My good friend Nathan Collins, whom I worked with at the Santa Fe Institute, has now joined the blogosphere with Nathan Explains Science. Welcome!
Nathan is a former theoretical astrophysicist who holds a PhD in political science. The first time I met him, I thought this meant that he studied awesome things like galactic warfare, blackhole coverups, and the various environmental disasters that come with unregulated and rampant terraforming. Fortunately for us, he instead studies actual politics and social science, which is probably more useful (and sadly more interesting) than astro-politics.
Here's Nathan explaining why he's now also a blogger:
...this gives me an place to tell you about science news that I think is interesting but that isn't necessarily going to get published in Science News or Nature's news section. For a variety of reasons, social science news especially doesn't get discussed as science, and that's unfortunate because there are scientific results coming out of psychology, political science, and economics that are vitally important for understanding the problems we face and the solutions we should pursue. In fact, there are a lot of old results that people should know about but don't because social science news seems less attractive than, say, finding a galaxy farther away than any other.
And, if you want to read more about the science, try out these stories, in which Nathan explains the heck out of narcissism, what makes us vote, political grammar and baby introspection:
Is Narcissism Good For Business?
Narcissists, new experiments show, are great at convincing others that their ideas are creative even though they're just average. Still, groups with a handful of narcissists come up with better ideas than those with none, suggesting that self-love contributes to real-world success.
Sweaty Palms and Puppy Love: The Physiology of Voting
Does your heart race at the sight of puppies? Do pictures of vomit make you sweat? If so, you may be more likely to vote.
Politicians, Watch Your Grammar
As congressional midterm elections approach in the United States, politicians are touting their credentials, likability, and, yes, sometimes even their policy ideas. But they may be forgetting something crucial: grammar. A new study indicates that subtle changes in sentence structure can make the difference between whether voters view a politician as promising or unelectable.
‘Introspection’ Brain Networks Fully Formed at Birth
Could a fetus lying in the womb be planning its future? The question comes from the discovery that brain areas thought to be involved in introspection and other aspects of consciousness are fully formed in newborn babies...
More Evidence for Hidden Particles?
Like Lazarus from the dead, a controversial idea that there may be a new, superhard-to-spot kind of particle floating around the universe has made a comeback. Using a massive particle detector, physicists in Illinois have studied the way elusive particles called antineutrinos transform from type or "flavor" to another, and their data bolster a decade-old claim that the rate of such transformation is so high that it requires the existence of an even weirder, essentially undetectable type of neutrino. Ironically, the same team threw cold water on that idea just 3 years ago, and other researchers remain skeptical.
Update 6 November 2010: added a new story by Nathan, on hidden particles.
posted November 5, 2010 08:04 AM in Scientifically Speaking | permalink | Comments (0)
October 27, 2010
Story-telling, statistics, and other grave insults
The New York Times (and the NYT Magazine) has been running a series of pieces about math, science and society written by John Allen Paulos, a mathematics professor at Temple University and author of several popular books. His latest piece caught my eye because it's a topic close to my heart: stories vs. statistics. That is, when we seek to explain something [1], do we use statistics and quantitative arguments using mainly numbers or do we use stories and narratives featuring actors, motivations and conscious decisions? [2] Here are a few good excerpts from Paulos's latest piece:
...there is a tension between stories and statistics, and one under-appreciated contrast between them is simply the mindset with which we approach them. In listening to stories we tend to suspend disbelief in order to be entertained, whereas in evaluating statistics we generally have an opposite inclination to suspend belief in order not to be beguiled. A drily named distinction from formal statistics is relevant: we’re said to commit a Type I error when we observe something that is not really there and a Type II error when we fail to observe something that is there. There is no way to always avoid both types, and we have different error thresholds in different endeavors, but the type of error people feel more comfortable may be telling.
...
I’ll close with perhaps the most fundamental tension between stories and statistics. The focus of stories is on individual people rather than averages, on motives rather than movements, on point of view rather than the view from nowhere, context rather than raw data. Moreover, stories are open-ended and metaphorical rather than determinate and literal.
It seems to me that for science, the correct emphasis should be on the statistics. That is, we should be more worried about observing something that is not really there. But as humans, statistics is often too dry and too abstract for us to understand intuitively, to generate that comfortable internal feeling of understanding. Thus, our peers often demand that we give not only the statistical explanation but also a narrative one. Sometimes, this can be tricky because the structure of the two modes of explanation are in fundamental opposition, for instance, if the narrative must include notions of randomness or stochasticity. In such a case, there is no reason for any particular outcome, only reasons for ensembles or patterns of outcomes. The idea that things can happen for no reason is highly counter intuitive [3], and yet in the statistical sciences (which is today essentially all sciences), this is often a critical part of the correct explanation [4]. For the social sciences, I think this is an especially difficult balance to strike because our intuition about how the world works is built up from our own individual-level experiences, while many of the phenomena we care about are patterns above that level, at the group or population levels [5].
This is not a new observation and it is not a tension exclusive to the social sciences. For instance, here is Stephen J. Gould (1941-2002), the eminent American paleontologist, speaking about the differences between microevolution and macroevolution (excerpted from Ken McNamara's "Evolutionary Trends"):
In Flatland, E.A. Abbot's (1884) classic science-fiction fable about realms of perception, a sphere from the world of three dimensions enters the plane of two-dimensional Flatland (where it is perceived as an expanding circle). In a notable scene, he lifts a Flatlander out of his own world and into the third dimension. Imagine the conceptual reorientation demanded by such an utterly new and higher-order view. I do not suggest that the move from organism to species could be nearly so radical, or so enlightening, but I do fear that we have missed much by over reliance on familiar surroundings.
An instructive analogy might be made, in conclusion, to our successful descent into the world of genes, with resulting insight about the importance of neutralism in evolutionary change. We are organisms and tend to see the world of selection and adaptation as expressed in the good design of wings, legs, and brains. But randomness may predominate in the world of genes--and we might interpret the universe very differently if our primary vantage point resided at this lower level. We might then see a world of largely independent items, drifting in and out by the luck of the draw--but with little islands dotted about here and there, where selection reins in tempo and embryology ties things together. What, then, is the different order of a world still larger than ourselves? If we missed the world of genic neutrality because we are too big, then what are we not seeing because we are too small? We are like genes in some larger world of change among species in the vastness of geological time. What are we missing in trying to read this world by the inappropriate scale of our small bodies and minuscule lifetimes?
To quote Howard T. Odum (1924-2002), the eminent American ecologist, on a similar theme: "To see these patterns which are bigger than ourselves, let us take a special view through the macroscope." Statistical explanations, and the weird and diffuse notions of causality that come with them, seem especially well suited to express in a comprehensible form what we see through this "macroscope" (and often what we see through microscopes). And increasingly, our understanding of many important phenomena, be they social network dynamics, terrorism and war, sustainability, macroeconomics, ecosystems, the world of microbes and viruses or cures for complex diseases like cancer, depend on us seeing clearly through some kind of macroscope to understand the statistical behavior of a population of potentially interacting elements.
Seeing clearly, however, depends on finding new and better ways to build our intuition about the general principles that take inherent randomness or contingency at the individual level and produce complex patterns and regularities at the macroscopic or population level. That is, to help us understand the many counter-intuitive statistical mechanisms that shape our complex world, we need better ways of connecting statistics with stories.
27 October 2010: This piece is also being featured on Nature's Soapbox Science blog.
-----
[1] Actually, even defining what we mean by "explain" is a devilishly tricky problem. Invariably, different fields of scientific research have (slightly) different definitions of what "explain" means. In some cases, a statistical explanation is sufficient, in others it must be deterministic, while in still others, even if it is derived using statistical tools, it must be rephrased in a narrative format in order to provide "intuition". I'm particularly intrigued by the difference between the way people in machine learning define a good model and the way people in the natural sciences define it. The difference appears, to my eye, to be different emphases on the importance of intuitiveness or "interpretability"; it's currently deemphasized in machine learning while the opposite is true in the natural sciences. Fortunately, a growing number of machine learners are interested in building interpretable models, and I expect great things for science to come out of this trend.
In some areas of quantitative science, "story telling" is a grave insult, leveled whenever a scientist veers too far from statistical modes of explanation ("science") toward narrative modes ("just so stories"). While sometimes a justified complaint, I think completely deemphasizing narratives can undermine scientific progress. Human intuition is currently our only way to generate truly novel ideas, hypotheses, models and principles. Until we can teach machines to generate truly novel scientific hypotheses from leaps of intuition, narratives, supported by appropriate quantitative evidence, will remain a crucial part of science.
[2] Another fascinating aspect of the interaction between these two modes of explanation is that one seems to be increasingly invading the other: narratives, at least in the media and other kinds of popular discourse, increasing ape the strong explanatory language of science. For instance, I wonder when Time Magazine started using formulaic titles for its issues like "How X happens and why it matters" and "How X affects Y", which dominate its covers today. There are a few individual writers who are amazingly good at this form of narrative, with Malcolm Gladwell being the one that leaps most readily to my mind. His writing is fundamentally in a narrative style, stories about individuals or groups or specific examples, but the language he uses is largely scientific, speaking in terms of general principles and notions of causality. I can also think of scientists who import narrative discourse into their scientific writing to great effect. Doing so well can make scientific writing less boring and less opaque, but if it becomes more important than the science itself, it can lead to "pathological science".
[3] Which is perhaps why the common belief that "everything happens for a reason" persists so strongly in popular culture.
[4] It cannot, of course, be the entire explanation. For instance, the notion among Creationists that natural selection is equivalent to "randomness" is completely false; randomness is a crucial component of way natural selection constructs complex structures (without the randomness, natural selection could not work) but the selection itself (what lives versus what dies) is highly non-random and that is what makes it such a powerful process.
What makes statistical explanations interesting is that many of the details are irrelevant, i.e., generated by randomness, but the general structure, the broad brush-strokes of the phenomena are crucially highly non-random. The chief difficulty of this mode of investigation is in correctly separating these two parts of some phenomena, and many arguments in the scientific literature can be understood as a disagreement about the particular separation being proposed. Some arguments, however, are more fundamental, being about the very notion that some phenomena are partly random rather than completely deterministic.
[5] Another source of tension on this question comes from our ambiguous understanding of the relationship between our perception and experience of free will and the observation of strong statistical regularities among groups or populations of individuals. This too is a very old question. It tormented Rev. Thomas Malthus (1766-1834), the great English demographer, in his efforts to understand how demographic statistics like birth rates could be so regular despite the highly contingent nature of any particular individual's life. Malthus's struggles later inspired Ludwig Boltzmann (1844-1906), the famous Austrian physicist, to use a statistical approach to model the behavior of gas particles in a box. (Boltzmann had previously been using a deterministic approach to model every particle individually, but found it too complicated.) This contributed to the birth of statistical physics, one of the three major branches of modern physics and arguably the branch most relevant to understanding the statistical behavior of populations of humans or genes.
posted October 27, 2010 07:15 AM in Scientifically Speaking | permalink | Comments (0)
August 13, 2010
Philip Zimbardo on our relationship with time
In this short film, a cartoonist illustrates a portion of a lecture by Philip Zimbardo (yes, that one) on his understanding of different peoples' relationship and perception of time. Mostly, it sounds pretty reasonable, especially if you ignore some of his categorical reasoning and think instead about the general idea of delayed rewards and how different preferences for delay (none, short, long) can lead to social conflict. Toward the end, however, I was rather disappointed at his generational bashing of young people, which he drapes in scientific language to make it sound reasonable. As someone who spent thousands of hours playing video games when I was younger, I have a hard time looking back and feeling any regret.
posted August 13, 2010 08:12 PM in Scientifically Speaking | permalink | Comments (3)
July 16, 2010
Confirmation bias in science
There's a decent meditation by Chris Lee on the problems of confirmation bias in science over at Nobel Intent, ArsTechnica's science corner. In its simplest form, confirmation bias is a particularly nasty mistake to make for anyone claiming to be a scientist. Lee gives a few particularly egregious (and famous) examples, and then describes one of his own experiences in science as an example of how self-corrective science works. I particularly liked the analogy he uses toward the end of the piece, where he argues that modern science is like a contact sport. Certainly, that's very much what the peer review and post-publication citation process can feel like.
Sometimes, however, it can take a long time for the good ideas to emerge out of the rough and tumble, particularly if the science involves complicated statistical analyses or experiments, if good data is hard to come by (or if the original data is unavailable), if there are strong social forces incentivizing the persistence of bad ideas (or at least, if there's little reward for scientists who want to sort out the good from the bad, for instance, if the only journals that will publish the corrections are obscure ones), or if the language of the field is particularly vague and ill-defined. [1]
Here's one of Lee's closing thoughts, which I think characterizes how science works when it is working well. The presence of this kind of culture is probably a good indicator of a healthy scientific community.
This is the difference between doing science from the inside and observing it from the outside. [Scientists] attack each other's ideas mercilessly, and those attacks are not ignored. Sometimes, it turns out that the objection was the result of a misunderstanding, and once the misunderstanding is cleared up, the objection goes away. Objections that are relevant result in ideas being discarded or modified. And the key to this is that the existence of confirmation bias is both acknowledged and actively fought against.
-----
[1] Does it even need to be said?
posted July 16, 2010 04:55 PM in Scientifically Speaking | permalink | Comments (0)
June 11, 2010
The Future of Terrorism
Attention conservation notice: This post mainly concerns an upcoming Public Lecture I'm giving in Santa Fe NM, as part of the Santa Fe Institute's annual lecture series.
Wednesday, June 16, 2010, 7:30 PM at the James A. Little Theater
Nearly 200 people died in the Oklahoma City bombing of 1995, over 200 died in the 2002 nightclub fire in Bali, and at least 2700 died in the 9/11 attacks on the World Trade Center Towers. Such devastating events captivate and terrify us mainly because they seem random and senseless. This kind of unfocused fear is precisely terrorism's purpose. But, like natural disasters, terrorism is not inexplicable: it follows patterns, it can be understood, and in some ways it can be forecasted. Clauset explores what a scientific approach can teach us about the future of modern terrorism by studying its patterns and trends over the past 50 years. He reveals surprising regularities that can help us understand the likelihood of future attacks, the differences between secular and religious terrorism, how terrorist groups live and die, and whether terrorism overall is getting worse.
Naturally, this will be my particular take on the topic, driven in part by my own research on patterns and trends in terrorism. There are many other perspectives, however. For instance, from the US Department of Homeland Security (from 2007), the US Department of Justice (from 2009) and the French Institute for International Relations (from 2006). Perhaps the main difference between these and mine is in my focus on taking a data- and model-driven approach to understanding the topic, and on emphasizing terrorism worldwide rather than individual conflicts or groups.
Update 13 July 2010: The video of my lecture is now online. The running time is about 80 minutes; the talk lasted about 55 and I spent the rest of the time taking questions from the audience.
posted June 11, 2010 08:49 PM in Terrorism | permalink | Comments (0)
April 20, 2010
People v. The scale-free networks hypothesis
The other night while skimming the nightly arxiv mailing, I was momentarily confused as to why a paper on cell phone networks [1] was in the q-bio mailing. Then I realized that these cellular networks were about actual cells: the kind that squirm and wiggle and master our every innovation in biochemical warfare. Turns out, I should have paid more attention.
This paper, by de Lomana, Beg, de Fabritiis and Villà-Freixa, is about the way Nature organizes biological networks inside cells, such as protein-protein interaction networks, transcriptional regularity networks, metabolic networks, etc. The authors apply new statistical methods [2] to more rigorously test an old and oft-repeated systems biology hypothesis: that biological networks universally exhibit a "scale free" structure, as shown by their degree distributions following a power-law form. The implication is that there's a class of "universal" evolutionary mechanisms [3] that build and maintain the structure of these networks, which is why they exhibit this common organizational pattern. Many scientists are not fans of this idea, and one of my favorite critiques is a 2005 paper by Tanaka titled "Scale-rich Metabolic Networks" [4].
Long-time readers will guess what's coming next. Power-law distributions, and the question of whether some empirical data can reasonably be claimed to follow one, and thus also whether we are licensed to infer certain kinds of causal mechanisms versus others, is a topic very close to my heart. A few years ago, I even made a mild attempt to lay direct siege to the scale free networks fortress by reanalyzing some recently published protein-interaction network data [5]. I'm happy to say that de Lomana et al. have done a much better and more comprehensive job than I did. In their paper, they used applied sound methods to a wide variety of biochemical data sets and surprisingly, or perhaps unsurprisingly, they found that none of these systems exhibit plausible power laws. In their words:
Our results demonstrate that the large-scale topology of the molecular interaction networks and the global mRNA and protein expression distributions examined here do not strictly follow power-law distributions. Moreover, none of the three heavy-tailed models tested had a universal agreement with the empirical data even when using the highest quality data sets available. Distributions are evidently heavy-tailed and for this type of data [maximum likelihood] analyses prove superior to graphical methods for assessing different tested distributions.
de Lomana et al. go on to discuss how these conclusions might be wrong, due to various uncertainties relating to the empirical data. I particularly liked this latter piece because it shows that they've thought carefully about the hypotheses, the uncertainties in their data, and how these interact. (Sadly for science, this kind of self-criticism is increasingly unfashionable in much of this literature.)
In their conclusions, de Lomana et al. keep the interpretation fairly narrow. I think more can be said. Here's me, two years ago, writing about one particular study that argued in support of the scale-free hypothesis:
...there must be a lot of non-scale-free structure in the network. This structure may have evolutionary or functional significance, since it's behavior is qualitatively different from the large-degree proteins. Unfortunately, the authors missed the opportunity to identify or discuss this because they were sloppy in their analysis. The moral here is that doing the statistics right can shed new light on the interactome's structure, and can actually generate new questions for future work... if we're ever to build scientific theories here, then we sure had better get the details right.
Tip to Brian Karrer and Cosma Shalizi.
Update 2 Sept. 2010: Jordi tells me that the paper has finally appeared as A.L.G. De Lomana, Q.K. Beg, G. De Fabritiis and J. Villà-Freixa. "Statistical Analysis of Global Connectivity and Activity Distributions in Cellular Networks." Journal of Computational Biology, 17(7): 869-878 (2010).
-----
[1] Statistical Analysis of Global Connectivity and Activity Distributions in Cellular Networks by Adrián López García de Lomana, Qasim K. Beg, G. de Fabritiis, Jordi Villà-Freixa. Journal of Computational Biology (Forthcoming).
Various molecular interaction networks have been claimed to follow power-law decay for their global connectivity distribution. It has been proposed that there may be underlying generative models that explain this heavy-tailed behavior by self-reinforcement processes such as classical or hierarchical scale-free network models. Here we analyze a comprehensive data set of protein-protein and transcriptional regulatory interaction networks in yeast, an E. coli metabolic network, and gene activity profiles for different metabolic states in both organisms. We show that in all cases the networks have a heavy-tailed distribution, but most of them present significant differences from a power-law model according to a stringent statistical test. Those few data sets that have a statistically significant fit with a power-law model follow other distributions equally well. Thus, while our analysis supports that both global connectivity interaction networks and activity distributions are heavy-tailed, they are not generally described by any specific distribution model, leaving space for further inferences on generative models.
[2] Disclaimer: I helped develop these methods.
[3] Specifically preferential-attachment-style mechanisms, such as duplication-mutation. Problematically, the language used in this area has led to enormous confusion. The name "scale free" has been attached both to a particular mechanism (preferential attachment) that generates a particular pattern (a power-law distribution) and to the pattern itself. So, when someone says "X is scale free", they could mean either that X was generated by preferential attachment or that X follows a power-law distribution. The problem, of course, is that there are many mechanisms that generate power-law distributions, so just because a preferential attachment mechanism implies a power-law distribution does not mean that if we observe a power-law distribution that we can correctly infer preferential attachment. If we do, we're almost surely committing a Type I error (also called an error of excessive credulity). Personally, when it comes to scientific theories, I would prefer to make errors of excessive skepticism, but I'm not sure many of my colleagues in network science share that preference.
[4] R. Tanaka, "Scale-Rich Metabolic Networks." Physical Review Letters 94, 168101 (2005).
[5] You may not know that I took that blog entry and turned it into a comment that I posted on the arxiv, or that I subsequently submitted the comment to Science. The comment was not published, but not because my criticism wasn't correct. Here's a short explanation of why it wasn't published.
posted April 20, 2010 02:20 PM in Scientifically Speaking | permalink | Comments (1)
January 12, 2010
The future of terrorism
Here's one more thing. SFI invited me to give a public lecture as part of their 2010 lecture series. These talks are open to, and intended for, the public. They're done once a month, in Santa Fe NM over most of the year. This year, the schedule is pretty impressive. For instance, on March 16, Daniel Dennett will be giving a talk about the evolution of religion.
My own lecture, which I hope will be good, will be on June 16th:
One hundred sixty-eight people died in the Oklahoma City bombing of 1995, 202 people died in the 2002 nightclub fire in Bali, and at least 2749 people died in the 9/11 attacks on the World Trade Center Towers. Such devastating events captivate and terrify us mainly because they seem random and senseless. This kind of unfocused fear is precisely terrorism's purpose. But, like natural disasters, terrorism is not inexplicable: it follows patterns, it can be understood, and in some ways it can be forecasted. Clauset explores what a scientific approach can teach us about the future of modern terrorism by studying its patterns and trends over the past 50 years. He reveals surprising regularities that can help us understand the likelihood of future attacks, the differences between secular and religious terrorism, how terrorist groups live and die, and whether terrorism overall is getting worse.
Also, if you're interested in my work on terrorism, there's now a video online of a talk I gave on their group dynamics last summer in Zurich.
posted January 12, 2010 10:53 AM in Self Referential | permalink | Comments (2)
November 17, 2009
How big is a whale?
One thing I've been working on recently is a project about whale evolution [1]. Yes, whales, those massive and inscrutable aquatic mammals that are apparently the key to saving the world [2]. They've also been called the poster child of macroevolution, which is why I'm interested in them, due to their being so incredibly different from their closest living cousins, who still have four legs and nostrils on the front of their face.
Part of this project requires understanding something about how whale size (mass) and shape (length) are related. This is because in some cases, it's possible to get a notion of how long a whale is (for example, a long dead one buried in Miocene sediments), but it's generally very hard to estimate how heavy it is. [3]
This goes back to an old question in animal morphology, which is whether size and shape are related geometrically or elastically. That is, if I were to double the mass of an animal, would it change its body shape in all directions at once (geometric) or mainly in one direction (elastic)? For some species, like snakes and cloven-hoofed animals (like cows), change is mostly elastic; they mainly get longer (snakes) or wider (bovids, and, some would argue, humans) as they get bigger.
About a decade ago, Marina Silva [4], building on earlier work [5], tackled this question quantitatively for about 30% of all mammal species and, unsurprisingly I think, showed that mammals tend grow geometrically as they change size. In short, yes, mammals are generally spheroids, and L = (const.) x M^(1/3). This model is supposed to be even better for whales: because they're basically neutrally buoyant in water, gravity plays very little role in constraining their shape, and thus there's less reason for them to deviate from the geometric model [6].
Collecting data from primary sources on the length and mass of living whale species, I decided to reproduce Silva's analysis [7]. In this case, I'm using about 2.5 times as much data as Silva had (n=31 species versus n=77 species), so presumably my results are more accurate. Here's a plot of log mass versus log length, which shows a pretty nice allometric scaling relationship between mass (in grams) and length (in meters):
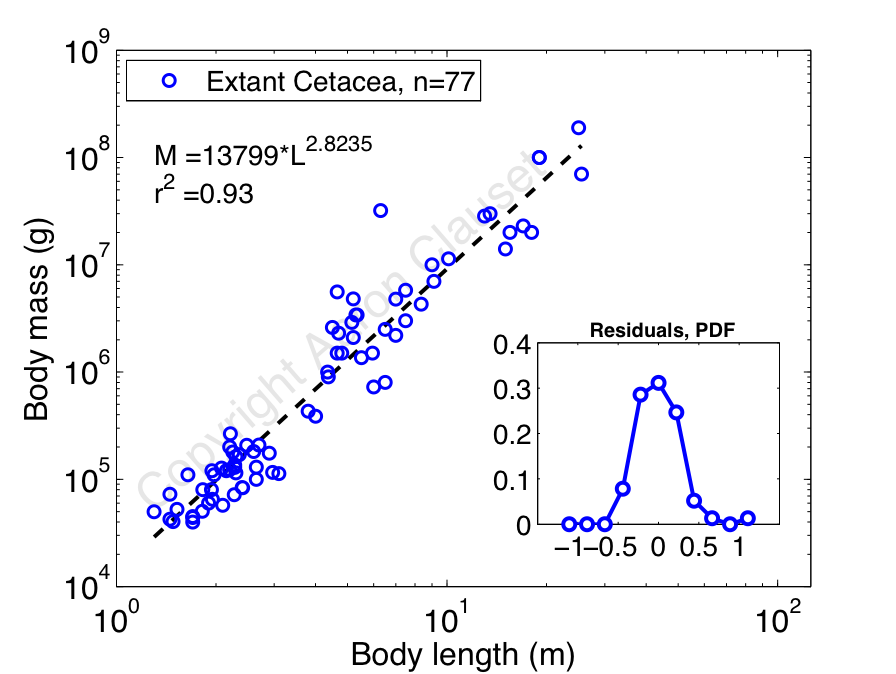
Aside from the fact that mass and length relate very closely, the most interesting thing here is that the estimated scaling exponent is less than 3. If we take the geometric model at face value, then we'd expect the mass of a whole whale to simply be its volume times its density, or

where k_1 and k_2 scale the lengths of the two minor axes (its widths, front-to-back and left-to-right) relative to the major axis (its length L, nose-to-tail), and the trailing constant is the density of whale flesh (here, assumed to be the density of water) [8].
If the constants k_1 and k_2 are the same for all whales (the simplest geometric model), then we'd expect a cubic relation: M = (const.) x L^3. But, our measured exponent is less than 3. So, this implies that k_1 and k_2 cannot be constants, and must instead increase slightly with greater length L. Thus, as a whale gets longer, it gets wider less quickly than we expect from simple geometric scaling. But, that being said, we can't completely rule out the hypothesis that the scatter around the regression line is obscuring a beautifully simple cubic relation, since the 95% confidence intervals around the scaling exponent do actually include 3, but just barely: (2.64, 3.01).
So, the evidence is definitely in the direction of a geometric relationship between a whale's mass and length. That is, to a large extent, a blue whale, which can be 80 feet long (25m), is just a really(!) big bottlenose dolphin, which are usually only 9 feet long (2.9m). That being said, the support for the most simplistic model, i.e., strict geometric scaling with constant k_1 and k_2, is marginal. Instead, something slightly more complicated happens, with a whale's circumference growing more slowly than we'd expect. This kind of behavior could be caused by a mild pressure toward more hydrodynamic forms over the simple geometric forms, since the drag on a longer body should be slightly lower than the drag on a wider body.
Figuring out if that's really the case, though, is beyond me (since I don't know anything about hydrodynamics and drag) and the scope of the project. Instead, it's enough to be able to make a relatively accurate estimation of body mass M from an estimate of body length L. Plus, it's fun to know that big whales are mostly just scaled up versions of little ones.
More about why exactly I need estimates of body mass for will have to wait for another day.
Update 17 Nov. 2009: Changed the 95% CI to 3 significant digits; tip to Cosma.
Update 29 Nov. 2009: Carl Zimmer, one of my favorite science writers, has a nice little post about the way fin whales eat. (Fin whales are almost as large as blue whales, so presumably the mechanics are much the same for blue whales.) It's a fascinating piece, involving the physics of parachutes.
-----
[0] The pictures are, left-to-right, top-to-bottom: blue whale, bottlenose dolphin, humpback whale, sperm whale, beluga, narwhal, Amazon river dolphin, and killer whale.
[1] Actually, I mean Cetaceans, but to keep things simple, I'll refer to whales, dolphins, and porpoises as "whales".
[2] Thankfully, the project doesn't involve networks of whales... If that sounds exciting, try this: D. Lusseau et al. "The bottlenose dolphin community of Doubtful Sound features a large proportion of long-lasting associations. Can geographic isolation explain this unique trait?" Behavioral Ecology and Sociobiology 54(4): 396-405 (2003).
[3] For a terrestrial mammal, it's possible to estimate body size from the shape of its teeth. Basically, mammalian teeth (unlike reptilian teeth) are highly differentiated and certain aspects of their shape correlate strongly with body mass. So, if you happen to find the first molar of a long dead terrestrial mammal, there's a biologist somewhere out there who can tell you both how much it weighed and what it probably ate, even if the tooth is the only thing you have. Much of what we know about mammals from the Jurassic and Triassic, when dinosaurs were dominant, is derived from fossilized teeth rather than full skeletons.
[4] Silva, "Allometric scaling of body length: Elastic or geometric similarity in mammalian design." J. Mammology 79, 20-32 (1998).
[5] Economos, "Elastic and/or geometric similarity in mammalian design?" J. Theoretical Biology 103, 167-172 (1983).
[6] Of course, fully aquatic species may face other biomechanical constraints, due to the drag that water exerts on whales as they move.
[7] Actually, I did the analysis first and then stumbled across her paper, discovering that I'd been scooped more than a decade ago. Still, it's nice to know that this has been looked at before, and that similar conclusions were arrived at.
[8] Blubber has a slight positive buoyancy, and bone has a negative buoyancy, so this value should be pretty close to the true average density of a whale.
posted November 17, 2009 04:33 PM in Evolution | permalink | Comments (2)
November 03, 2009
The trouble with community detection
I'm a little (a month!) late in posting it, but here's a new paper, largely by my summer student Ben Good, about the trouble with community detection algorithms.
The short story is that the popular quality function called "modularity" (invented by Mark Newman and Michelle Girvan) admits serious degeneracies that make it somewhat impractical to use in situations where the network is large or has a non-trivial number of communities (a.k.a. modules). At the end of the paper, we briefly survey some ways to potentially mitigate this problem in practical contexts.
The performance of modularity maximization in practical contexts
Benjamin H. Good, Yves-Alexandre de Montjoye, Aaron Clauset, arxiv:0910.0165 (2009).
Although widely used in practice, the behavior and accuracy of the popular module identification technique called modularity maximization is not well understood. Here, we present a broad and systematic characterization of its performance in practical situations. First, we generalize and clarify the recently identified resolution limit phenomenon. Second, we show that the modularity function Q exhibits extreme degeneracies: that is, the modularity landscape admits an exponential number of distinct high-scoring solutions and does not typically exhibit a clear global maximum. Third, we derive the limiting behavior of the maximum modularity Q_max for infinitely modular networks, showing that it depends strongly on the size of the network and the number of module-like subgraphs it contains. Finally, using three real-world examples of metabolic networks, we show that the degenerate solutions can fundamentally disagree on the composition of even the largest modules. Together, these results significantly extend and clarify our understanding of this popular method. In particular, they explain why so many heuristics perform well in practice at finding high-scoring partitions, why these heuristics can disagree on the composition of the identified modules, and how the estimated value of Q_max should be interpreted. Further, they imply that the output of any modularity maximization procedure should be interpreted cautiously in scientific contexts. We conclude by discussing avenues for mitigating these behaviors, such as combining information from many degenerate solutions or using generative models.
posted November 3, 2009 08:55 AM in Networks | permalink | Comments (0)
June 27, 2009
A "Baloney" Detection Ket
I like this.
(tip to onegoodmove)
posted June 27, 2009 07:12 PM in Scientifically Speaking | permalink | Comments (0)
January 28, 2009
On coincidences
This reminds me of a frustrating conversation I once had with a gentleman on an airplane. He was convinced by the obnoxiously popular idea that everything happens for a reason, and believed that there was no such thing as a coincidence. Unsurprisingly, he was extremely delighted when he discovered, after we'd been chatting for 10 minutes or so inside the terminal, that our seats on the plane were directly across the aisle from each other.
I also like the fact that this video (around 4:20) quotes and discusses The Infinite Monkey Theorem and the results of an experiment conducted in England to test what actually happens when Macaque monkeys are given typewriters. I like this fact because a print out of the corresponding Wikipedia page (which the narrator in the video has clearly read) has been posted on my office door for over a year now, with the results of the experiment highlighted. Coincidentally, my current office mate studies Macaque monkeys, although sadly she was not involved in the experiment.
posted January 28, 2009 12:43 AM in Scientifically Speaking | permalink | Comments (1)
October 15, 2008
Power laws in the mist
Flipping through last week's Science yesterday, I was pleasantly surprised to see another entry [1] in the ongoing effort to construct a map of all protein interactions in the model organism yeast (S. cerevisiae). The idea here is that since sequencing DNA is now relatively easy, and we have a pretty good idea of which genes code for proteins, it would be useful to know which of these proteins actually interact with each other. As it's often said, DNA gives us a "parts list" of an organism, but doesn't tell us much about how those parts work together to make life happen [2].
The construction of this "interactome" is a tricky task, requiring us to first build all the proteins, and then to test each pair for whether they interact. The first step is easy with modern methods. The second step, however, is hard: it requires us to test 100-1000 million possible interactions, which would take even a very diligent graduate lab many lifetimes to finish. Instead of that kind of brute-force approach, scientists rely on clever molecular techniques to test for many interactions at once. There have been several high profile efforts along these lines [3], and, many would argue, a lot of real progress.
With an interactome map in hand, the hope is that we can ask biologically meaningful questions about the patterns of interactions, i.e., we can do network analysis on it, and shed light on fundamental questions about how cells work as a whole. We know now that there are some rather serious problems with molecular methods used to infer the interaction network, and these cause more cautious scientists to wonder how much real biology our current maps actually capture [4]. On the other hand, each new interactome is probably better than the last one because molecular techniques do improve, and we know more about how to deal with their problems.
All this stuff aside, even if we take the inferred data at face value, there continue to be problems with its interpretation. For instance, a popular question to ask of interactome data is whether the degree distribution of the network is "scale free." If it does follow a power-law distribution, then this is viewed as being interesting, and perhaps support for some of the several theories about the large-scale organization of such networks.
Answering this kind of question requires care, however, and long-time readers will already know where I'm going with this train of thought. In the text, Yu et al. say
As found previously for other macromolecular networks, the "connectivity" or degree distribution of all three data sets is best approximated by a power-law."
To the right is their data [5] for the Y2H-union data set (one of the three claimed to follow power laws), replotted as a complementary cumulative distribution function (CDF) [6], which cleans up some of the visual noise in the original plot. On top of their data, I've plotted the power-law distribution they quote in their paper (alpha=2.4), which shows just how horrible their fit actually is. We can quantitatively measure how good the fit is by calculating a p-value for the fit (p=0.00 +/- 0.03) [7], which shows that the data definitely do not follow the claimed power law. So much for being scale free? Maybe.
The authors' first mistake was to use linear regression to fit a power law to their data, which can dramatically overweight the large but rare events and, more often than not, finds power-law behavior when none really exists. A better method for choosing alpha is maximum likelihood. If we do this naively, i.e., assuming that all of our data are drawn from a power law, we get the plot on the left.
The fit here is better for more of the data (which is concentrated among the small values of x), but pretty terrible for larger values. And the p-value is still too small for this power-law to be a plausible model of the data. So, the "scale free" claim still seems problematic.
But, we can be more sophisticated by assuming that only the larger values in the data are actually distributed like a power law. This nuance is reasonable since being "scale free" shouldn't depend on what happens for small values of x. Using appropriate tools [8] to choose the place where the power-law behavior starts, we get the power law on the right. Now, we have a more nuanced picture: the 200-odd largest values in the data set (about 10% of the proteins) really are plausibly distributed (p=0.95 +\- 0.03) like a power law, while small values are distributed in some other, non-power-law, way.
So, although the patterns of interactions for large-degree proteins in the Y2H-union data do seem to follow a power law, it is not the power law that the authors claim it is. And, since the truly plausible power-law behavior only holds for top 10% of the proteins, it means there must be a lot of non-scale-free structure in the network. This structure may have evolutionary or functional significance, since it's behavior is qualitatively different from the large-degree proteins. Unfortunately, the authors missed the opportunity to identify or discuss this because they were sloppy in their analysis. The moral here is that doing the statistics right can shed new light on the interactome's structure, and can actually generate new questions for future work.
So far, I've only shown the re-analysis of one of their three data sets, but repeating the same steps with their other two data sets (Combined-AP/MS and LC-multiple) yields similarly worrying results: the Combined-AP/MS data cannot be considered power-law distributed by any stretch of the imagination, and the LC-multiple data are a marginal case. These conclusions certainly mean something about the structure of the different interactomes, but the authors again miss an opportunity to discuss it. The table below summarizes the re-analysis results for the three data sets; the power laws quoted by Yu et al. are given under the heading "regression", while the correct fits are under the second heading:

Notably, the quoted power laws are completely incompatible with the maximum likelihood ones, illustrating that even when there is power-law behavior to be found (e.g., in the Y2H-union data) regression misleads us. Worse, when there is no power-law behavior to be found, regression can give us spuriously high confidence (e.g., the R^2 value for the Combined-AP/MS data). Since pictures are also fun, here are the remaining two plots of the maximum likelihood power laws, showing pretty clearly why the Combined-AP/MS can be rejected as a power law.
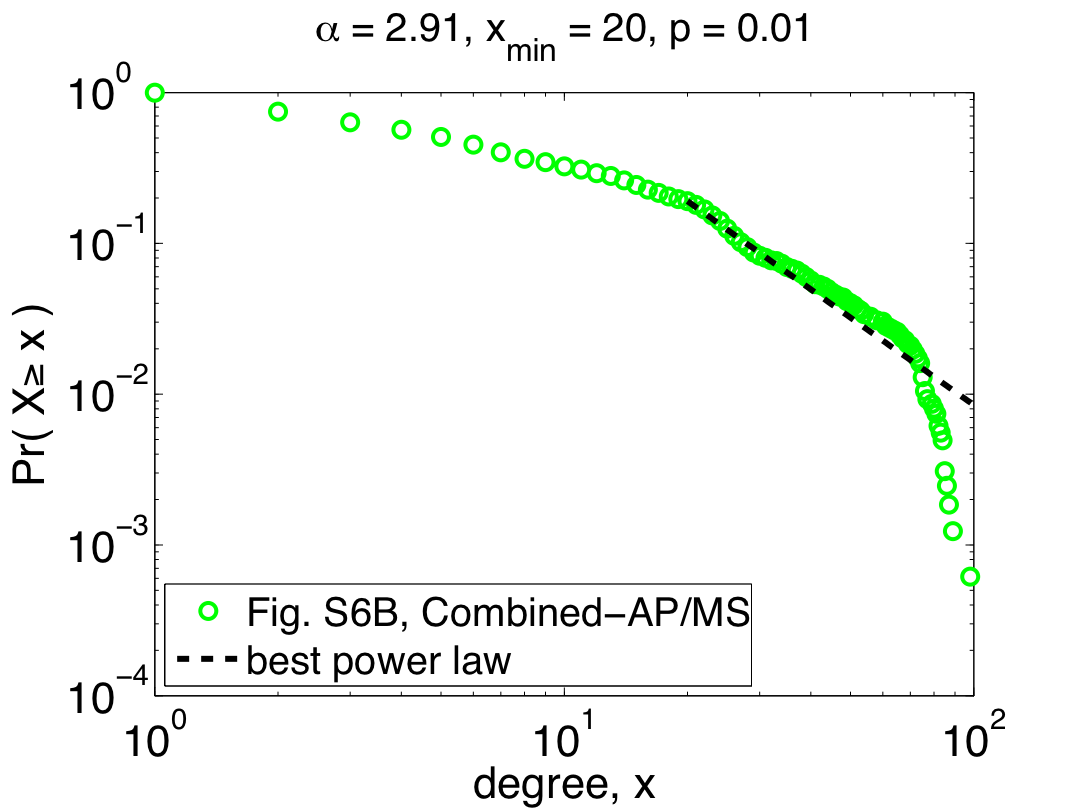
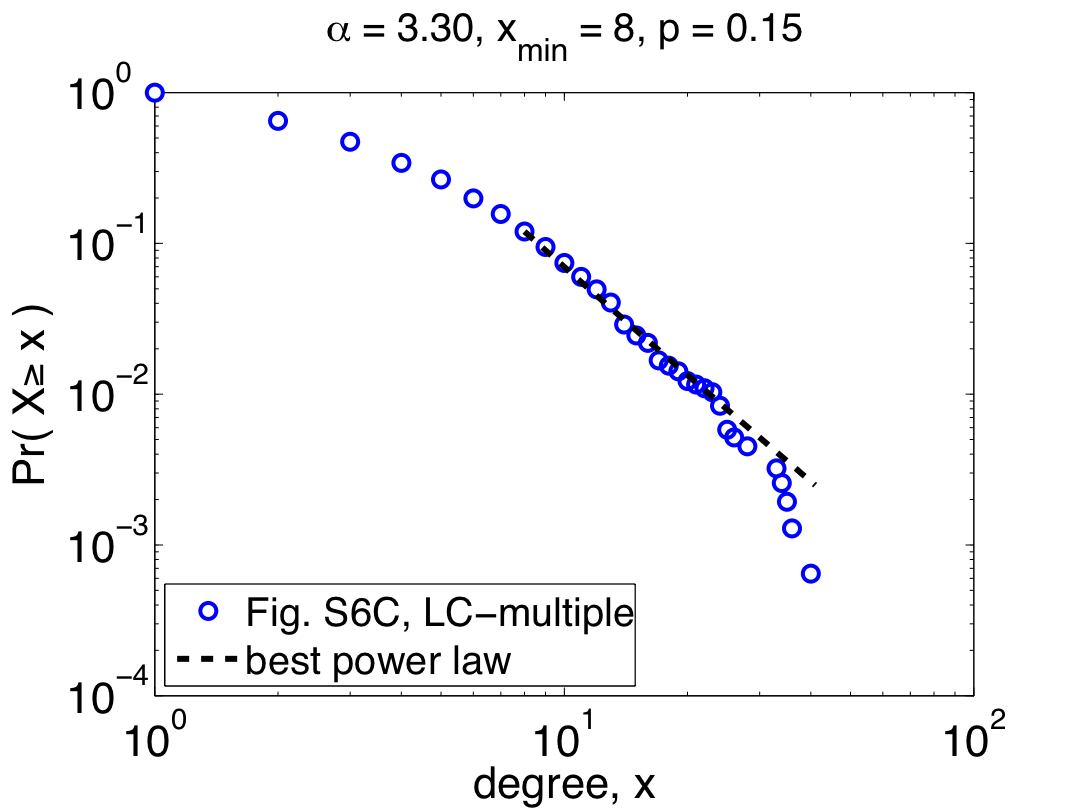
But, not content to merely claim power-law behavior, the authors go on to claim that the power law was the best model [9]. Digging around in the appendices, you find out that they compared their power law with one alternative, a power law with an exponential cutoff (also fitted using regression). Unfortunately, regressions are also the wrong tool for this kind of question, and so this claim is also likely to be wrong [10]. In fact, adding an exponential cutoff is almost always a better model of data than a regular power law. If you felt like wearing your cynical hat today, you might conclude that the authors had some non-scientific motivation to conclude that these networks were "scale free".
There are many aspects of the Yu et al. study that seem entirely reasonable, and majority of the paper is about the experimental work to actually construct their version of the yeast interactome. In this sense, the paper does push the field forward in a meaningful way, and it's mildly unfair of me to critique only the power-law analysis in the paper -- a small part of a very large project.
On the other hand, the goal of constructing interactomes is to ultimately understand what mechanisms create the observed patterns of interactions, and if we're ever to build scientific theories here, then we sure had better get the details right. Anyway, I'm hopeful that the community will eventually stop doing ridiculous things with power-law distributed data, but until reviewers start demanding it, I think we'll see a lot more sloppy science and missed opportunities, and our scientific understanding of the interactome's structure and its implications for cellular function will suffer.
Update 17 October 2008: Nathan Eagle suggested that a bit more detail on why maximum likelihood is preferable to regression in this case would be useful. So, here it is:
Whenever you're fitting a model to data, there are some properties you want your fitting procedure to have in order to trust it's results. The most important of these is consistency. Consider a game in which I play the role of Nature and generate some power-law distributed data for you. You then play the role of Scientist and try to figure out which power law I used to generate the data (i.e., what values of alpha and xmin I chose before I generated the data). A consistent procedure is one whose answers get closer to the truth the more data I show you.
Maximum likelihood estimators (MLEs) are guaranteed to be consistent (they also have normally-distributed errors, which lets you quote a "standard error" for how precise your answer is), which means you can trust them not to mislead you. In fact, regression techniques like least squares are MLEs for a situation where two measurable properties (e.g., pressure and volume) are related, modulo normal measurement errors. But, these assumptions fail if the data is instead a distribution, which means regression methods are not consistent for detecting power-law distributions. In other words, using regression to detect a power-law distribution applies the wrong model to the problem, and it should be no surprise that it produces wildly inaccurate guesses about power-law behavior. More problematically, though, it doesn't give any warning that it's misbehaving, which can lead to confident claims of power-law distributions when none actually exist (as in the Combined-AP/MS case above). If you're still thirsty for more details, you can find them in Appendix A of [8] below.
Update 6 January 2009: There's now a version of these comments on the arxiv.
-----
[1] Yu et al., "High Quality Binary Protein Interaction Map of the Yeast Interactome Network." Science 322, 104 (2008).
[2] Although scientists in this area often talk about how important the interactome is for understanding how life works, it's only part of the puzzle. Arguably, a much more important problem is understanding how genes interact and regulate each other.
[3] For yeast, these are basically Fromont-Racine, Rain and Legrain, Nature Genetics 16, 277 (1997), Uetz et al., Nature 402, 623 (2000), and Ito et al., PNAS 98, 4569 (2001).
[4] For instance, worryingly high false-positive rates, ridiculously high false-negative rates (some of these are driven by the particular kind of molecular trick used, which can miss whole classes of proteins, e.g., nucleus-bound ones) and a large amount of irreproducibility by alternative techniques or other groups. This last point leads me to believe that all of the results so far should not yet be trusted. Interested readers could refer to Bader, Chaudhuri, Rothberg and Chant, "Gaining confidence in high-throughput protein interaction networks." Nature Biotechnology, 22 78 (2004).
[5] Admittedly, it's not exactly their data, but rather a very close approximation to it, derived from Fig. S6A in their supporting online material using DataThief and a lot of patience.
[6] The complementary CDF is defined as Pr( X >= x ). If the data are distributed according to a power law, then this function will be straight on log-log axes. The reverse, however, is not true. That is, being straight on log-log axes is not a sufficient condition for being power-law distributed. There are many kinds of data that look straight on log-log axes but which are not power-law distributed.
[7] The conventional use of p-values is to determine whether two things are "significantly different" from each other. If p<0.1, then the conclusion is that they are different. Here, we're testing the reverse: if p>0.1, then we conclude that the two things are plausibly the same.
[8] Clauset, Shalizi and Newman, "Power-law distributions in empirical data." arXiv:0706.1062 (2007).
Implementations of these methods are available for free online.
[9] Actually, the authors say "best approximated by", which is a disingenuous hedge. What does it mean to "approximate" here, and how bad must the approximation be to be considered unreasonable? If the goal is poetry, then maybe approximations like the one they use are okay, but if the goal is science, we should strive for more precise and more meaningful statements.
[10] If they'd wanted to do it right, then they would have used standard, trustworthy tools for comparing their two models, such as a likelihood ratio test.
[11] A colleague of mine asked me why I didn't write this up as an official "Comment" for Science. My response was basically that I didn't think it would make a bit of difference if I did, and it's probably more useful to do it informally here, anyway. My intention is not to trash the Yu et al. paper, which as I mentioned above has a lot of genuinely good bits in it, but rather to point out that the common practices (i.e., regressions) for analyzing power-law distributions in empirical data are terrible, and that better, more reliable methods both exist and are easy to use. Plus, I haven't blogged in a while, and grumping about power laws is as much a favorite past time of mine as publishing bad power laws apparently is for some people.
posted October 15, 2008 03:18 PM in Scientifically Speaking | permalink | Comments (2)
September 11, 2008
We're still here
Despite some mildly ridiculous fears that science (in the form of the Large Hadron Collider at [1] CERN in Gevena Switzerland) would cause the world would end yesterday we're still here. Whew. It must have been God's Will. To celebrate, here's a mildly ridiculous rap song about the LHC experiment (which, quite pleasantly, gets the physics right).
(Tip to Tanya for the video.)
Update 12 September 2008: The New York Times has run a well written piece by Brian Greene on the LHC and it's significance.
-----
[1] For more information about what CERN is, check out this 3 minute video on YouTube about it.
posted September 11, 2008 02:05 PM in Humor | permalink | Comments (1)
June 30, 2008
More familiar than we thought
The nearly 10,000 living species of birds are amazingly diverse, and yet we often think of them as being fundamentally different from the more familiar 4000-odd mammalian species. For instance, bird brains are organized very differently from mammals -- birds lack the neocortex that we humans exhibit so prominently, among other things. The tacit presumption derived from this structural difference has long been that birds should not exhibit some of the neurological behaviors that mammals exhibit. And yet, evidence continues to emerge demonstrating that birds are at least functionally very much like mammals, exhibiting tools use, cultural knowledge , long-term planning behavior, and creativity among other things.
A recent study in the Proceedings of the National Academy of Science (USA) adds another trait: sleeping [1,2], at least among song birds. By hooking up some zebra finches to the machinery usually used to measure the brain activity of sleeping mammals, Philip Low and his colleagues discovered that song-bird brains exhibit the same kind of sleeping-brain activity (slow waves, REM, etc.) normally seen in mammals. The authors avoid the simplistic explanation that the cause of this similarity is due to a shared ancestry, i.e., mammalian-style sleep evolved in the common ancestor of birds and mammals, which would be about 340 million years ago (with the origin of the Amniote class of animals). This hypothesis would imply (1) that all birds should sleep this way (but the current evidence suggests that it's only song-birds that do so), and (2) that other amniotes like lizards would have mammalian-like sleep patterns (which they apparently do not).
So, the similarity must therefore be an example of convergent evolution, i.e., birds and mammals evolved this kind of sleep behavior independently. The authors suggest that this convergence is because there are functionally equivalent regions of mammal and bird brains (a familiar idea for long-time readers of this blog) [3] and that these necessitate the same kind of sleep behavior. That is, song birds and mammals sleep the same way for the same reason. But, without understanding what mammalian-like sleep behavior is actually for, this could be mere speculation, even though it seems like it's on the right track. Given the other similarities of complex behavior seen in birds and mammals, it's possible that this kind of sleep behavior is fundamental to complex learning behaviors, although there could be other explanations too (e.g., see [3] below). At the very least, this similarity of behavior in evolutionarily very distant species gives us a new handle into understanding why we, and other species, sleep the way we do.
Update 30 June 2008: The New York Times also has an article in its science section about this phenomenon.
-----
[1] "Mammalian-like features of sleep structure in zebra finches." P. S. Low, S. S. Shank, T. J. Sejnowski and D. Margoliash. PNAS 105, 9081-9086 (2008).
A suite of complex electroencephalographic patterns of sleep occurs in mammals. In sleeping zebra finches, we observed slow wave sleep (SWS), rapid eye movement (REM) sleep, an intermediate sleep (IS) stage commonly occurring in, but not limited to, transitions between other stages, and high amplitude transients reminiscent of K-complexes. SWS density decreased whereas REM density increased throughout the night, with late-night characterized by substantially more REM than SWS, and relatively long bouts of REM. Birds share many features of sleep in common with mammals, but this collective suite of characteristics had not been known in any one species outside of mammals. We hypothesize that shared, ancestral characteristics of sleep in amniotes evolved under selective pressures common to songbirds and mammals, resulting in convergent characteristics of sleep.
[2] New Scientist has a popular science piece about the PNAS article.
[3] Mammals and birds have another important convergent similarity: they are both warm-blooded, but their common ancestor was cold-blooded. Thus, warm-bloodedness had to evolve independently for birds and for mammals, a phenomenon known as polyphyly. One interesting hypothesis is that warm-bloodedness and mammalian-like sleep patterns are linked somehow; if so, then presumably sleeping has something fundamental to do with metabolism, rather than learning as is more popularly thought. Of course, the fact that the similarity in sleeping seems to be constrained to song-birds rather than all birds poses some problems for the metabolism idea.
posted June 30, 2008 08:52 AM in Obsession with birds | permalink | Comments (2)
March 28, 2008
Is there a Physics of Society, redux
As I mentioned before, it's unlikely that I'll end up posting anything in depth about my thoughts about the Physics of Society workshop I ran back in January. On the other hand, I've been sitting on a couple of things related to a physics of society, so here they are.
Andrew Gelman (Statistics and Political Science at Columbia U.) has a nice critique about the trouble with social sciences that he's put under the pithy heading of "Thou shalt not sit with statisticians nor commit a social science". I admit that I'm deeply sympathetic to these criticisms, at least partially because in spite of a lot of effort, and a lot of writing, the social sciences don't appear to have produced much. Of course, there are lots of plausible explanations for this, including the usual refrain that social sciences are much harder than the natural sciences because humans are wily creatures, culture changes over time but has a huge influence on human behavior, and even 10^9 humans is nothing compared to the 10^20s of particles statistical physicists often consider. Another explanation that was mentioned at my workshop by Carter Butts is that relative to the natural sciences, the social sciences are drastically under-funded and under-staffed. One of my personal suspicions, however, is that social science has been hindered by a lack of good data by which to actually test the theories social scientists kick around. This kind of empirical vacuum can encourage researchers to develop all sorts of bad habits, and physicists interesting in social science topics (e.g., opinion dynamics) are by no means immunized against these by nature of the physics training.
This summer, Dirk Helbing and colleagues are running a workshop on the future of quantitative sociology; held in Zurich August 18-23, which looks quite interesting. (Frank Schweitzer is another of the organizers, and on the first night of my workshop, Frank told me about a similar meeting on sociophysics that he helped organize back in 2002.) Dirk is an exception among physicists working on sociological questions, as he actually conducts controlled experiments on human traffic behavior in his laboratory. These have produced some very nice results, and developed some nice connections with turbulent flows. But, there are a host of other sociological questions that have, for the most part, remained wholly inaccessible to controlled experimentation. Matt Salganik's presentation about his experimental work using an online environment got me very excited about the possibility that computer technology can help solve some of the tricky problems with social influence, framing effects, etc. that usually make experiments in this area inconclusive. Another interesting possibility is behavioral economics (which ETH Zurich is strong in). That is, perhaps by adapting techniques from these experiments, we can better understand, for instance, the roles that imitation and homophily play in the way humans modify their behavior in social settings.
Naturally, the interest in controlled experiments or in physics-style modeling of social phenomena is not new, and sociologists have been arguing over how best to study social behavior for more than 100 years. The recent interest by physicists in social phenomena may, in part, be explainable by the massive amounts of electronically collected data now available. Sociologists seem to have noticed too, to some degree. For instance, a lengthy article by Emirbayer from 1997 in the American Journal of Sociology criticizes sociology's tendency to focus on static or inherent properties of people rather than on the dynamic or process-based emphasis that appeals more to physicists. At the workshop, John M. Roberts gave a nice presentation of the historical interactions between physics and sociology, but pointed out that usually sociolgists' interest in dynamic or process-based models didn't last more than a few years each time it cropped up, possibly because sociologists often relied on metaphorical models (e.g., thinking of the social equivalents of "heat" or "leverage") that ultimately didn't help them make any real predictions. From my point of view, if this revival of interest in dynamic and quantitative models of social behavior is to turn into real scientific progress, then I think the key is going to be better testing of models with data. It's easy (and fun!) to do math, but it's not science until there's a meaningful comparison with real data.
posted March 28, 2008 08:40 AM in Scientifically Speaking | permalink | Comments (5)
October 25, 2007
Untangling the knots
Attention conservation notice: this is a posting about a seminar at SFI.
The State of String Theory, with David Gross
Friday, 26 October, 2007 at 12:15 PM in the Noyce Conference Room (Santa Fe Institute, Santa Fe NM)
David Gross won the 2004 Nobel Prize in Physics for his work on asymptotic freedom, and is currently the Frederick W. Gluck Chair in Theoretical Physics at (and director of) the Kavli Institute for Theoretical Physics, UC - Santa Barbara. So, in short, this should be interesting...
posted October 25, 2007 02:35 AM in Scientifically Speaking | permalink | Comments (4)
September 12, 2007
Is terrorism getting worse? A look at the data. (part 2)
Cosma and Matt both wanted to see how the trend fares when we normalize by the increase in world popluation, i.e., has terrorism worsened per capita. Pulling data from the US Census's world population database, we can do this. The basic facts are that the world's population has increased at a near constant rate over the past 40 years, going from about 3.5 billion in 1968 to about 6.6 billion in 2007. In contrast, the total number of deaths per year from terrorism (according to MIPT) has gone from 115 (on average) over the first 10 years (1968 - 1977), to about 3900 (on average) over the last 10 years (1998 - 2007). Clearly, population increase alone (about a factor of 2) cannot explain the increase in total deaths per year (about a factor of 34).
However, this view gives a slightly misleading picture because the MIPT changed the way it tracked terrorism in 1998 to include domestic attacks worldwide. Previously, it only tracked transnational terrorism (target and attackers from different countries), so part of the the apparent large increase in deaths from terrorism in the past 10 years is due to simply including a greater range of events in the database. Looking at the average severity of an event circumvents this problem to some degree, so long as we assume there's no fundamental difference in the severity of domestic and transnational terrorism (fortunately, the distributions pre-1998 and post-1998 are quite similar, so this may be a reasonable assumption).
The misleading per capita figure is immediately below. One way to get at the question, however, is to throw out the past 10 years of data (domestic+transnational) and focus only on the first 30 years of data (transnational only). Here, the total number of deaths increased from the 115 (on average) in the first decade to 368 in the third decade (1988-1997), while the population increased from 3.5 billion in 1968 to 5.8 billion in 1997. The implication being that total deaths from transnational terrorism have increased more quickly than we would expect based on population increases, even if we account for the slight increase in lethality of attacks over this period. Thus, we can argue that the frequency of attacks has significantly increased in time.
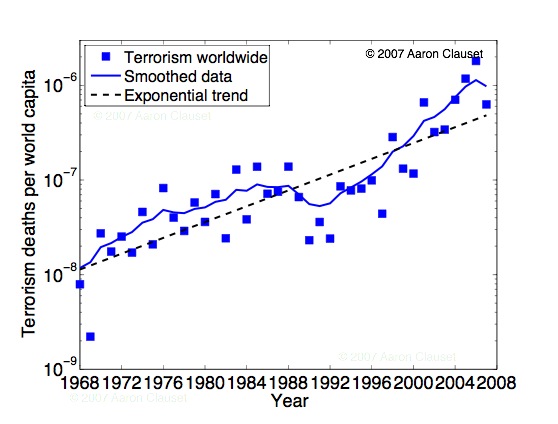
The more clear per capita-event figure is the next one. What's remarkable is that the per capita-event severity is extremely stable over the past 40 years, at about 1 death per billion (dpb) per event. This suggests that, if there really has been a large increase in the total deaths (per capita) from terrorism each year (as we argued above), then it must be mainly attributable to an increase in the number of lethal attacks each year, rather than attacks themselves becoming worse.
So, is terrorism getting worse? The answer is typically no, but that terrorism is becoming a more popular mode of behavior. From a policy point of view, this would seem a highly problematic trend.
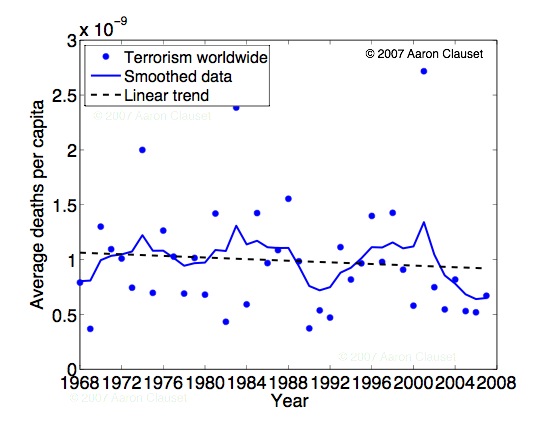
A. Clauset, M. Young and K. S. Gledistch, "On the Frequency of Severe Terrorist Attacks." Journal of Conflict Resolution 51(1): 58 - 88 (2007).
posted September 12, 2007 08:12 AM in Political Wonk | permalink | Comments (2)
September 11, 2007
Is terrorism getting worse? A look at the data.
Data taken from the MIPT database and includes all events that list at least one death (10,936 events; 32.3% as of May 17, 2007). Scatter points are the average number of deaths for lethal attacks in a given year. Linear trend has a slope of roughly 1 additional death per 20 years, on average. (Obviously, a more informative characterization would be the distribution of deaths, which would give some sense of the variability about the average.) Smoothing was done using an exponential kernel, and black triangles indicate the years (1978, 1991 and 2006) of the local minima of the smoothed function. Other smoothing schemes give similar results, and the auto-correlation function on the scatter data indicates that the average severity of lethal attacks oscillates with a roughly 13 year periodicity. If this trend holds, note that 2006 was a low-point for lethal terrorism.
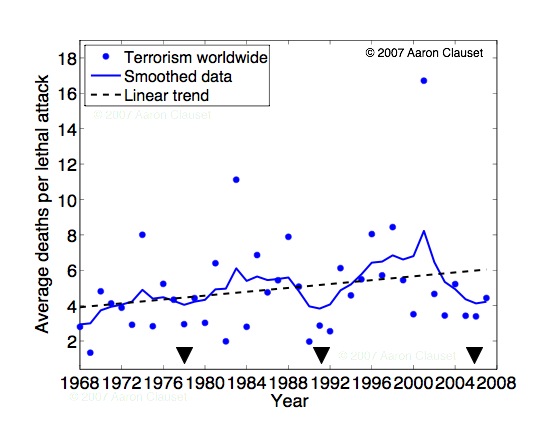
A. Clauset, M. Young and K. S. Gledistch, "On the Frequency of Severe Terrorist Attacks." Journal of Conflict Resolution 51(1): 58 - 88 (2007).
posted September 11, 2007 10:33 AM in Political Wonk | permalink | Comments (3)
August 21, 2007
Sleight of mind
There's a nice article in the NYTimes right now that ostensibly discusses the science of magic, or rather, the science of consciousness and how magicians can engineer false assumptions through their understanding of it. Some of the usual suspects make appearances, including Teller (of Penn and Teller), Daniel Dennett (that rascally Tufts philosopher who has been in the news much of late over his support of atheism and criticism of religion) and Irene Pepperberg, whose African parrot Alex has graced this blog before (here and here). Interestingly, the article points out a potentially distant forerunner of Alex named Clever Hans, a horse who learned not arithmetic, but his trainer's unconscious suggestions about what the right answers were (which sounds like pretty intelligent behavior to me, honestly). Another of the usual suspects is the wonderful video that, with the proper instruction to viewers, conceals a person in a gorilla suit walking across the screen.
The article is a pleasant and short read, but what surprised me the most is that philosophers are, apparently, still arguing over whether consciousness is a purely physical phenomenon or does it have some additional immaterial component, called "qualia". Dennett, naturally, has the best line about this.
One evening out on the Strip, I spotted Daniel Dennett, the Tufts University philosopher, hurrying along the sidewalk across from the Mirage, which has its own tropical rain forest and volcano. The marquees were flashing and the air-conditioners roaring — Las Vegas stomping its carbon footprint with jackboots in the Nevada sand. I asked him if he was enjoying the qualia. “You really know how to hurt a guy,” he replied.
For years Dr. Dennett has argued that qualia, in the airy way they have been defined in philosophy, are illusory. In his book “Consciousness Explained,” he posed a thought experiment involving a wine-tasting machine. Pour a sample into the funnel and an array of electronic sensors would analyze the chemical content, refer to a database and finally type out its conclusion: “a flamboyant and velvety Pinot, though lacking in stamina.”
If the hardware and software could be made sophisticated enough, there would be no functional difference, Dr. Dennett suggested, between a human oenophile and the machine. So where inside the circuitry are the ineffable qualia?
This argument is just a slightly different version of the well-worn Chinese room thought experiment proposed by John Searle. Searle's goal was to undermine the idea that the wine-tasting machine was actually equivalent to an oenophile (so-called "strong" artificial intelligence), but I think his argument actually shows that the whole notion of "intelligence" is highly problematic. In other words, one could argue that the wine-tasting machine as a whole (just like a human being as a whole) is "intelligent", but the distinction between intelligence and non-intelligences becomes less and less clear as one considers poorer and poorer versions of the machine, e.g., if we start mucking around with its internal program, so that it makes mistakes with some regularity. The root of this debate, which I think has been well-understood by critics of artificial intelligence for many years, is that humans are inherently egotistical beings, and we like feeling that we are special in some way that other beings (e.g., a horse or a parrot) are not. So, when pressed to define intelligence scientifically, we continue to move the goal posts to make sure that humans are always a little more special than everything else, animal or machine.
In the end, I have to side with Alan Turing, who basically said that intelligence is as intelligences does. I'm perfectly happy to dole out the term "intelligence" to all manner of things or creatures to various degrees. In fact, I'm pretty sure that we'll eventually (assuming that we don't kill ourselves off as a species, in the meantime) construct an artificial intelligence that is, for all intents and purposes, more intelligent than a human, if only because it won't have the enumerable quirks and idiosyncrasies (e.g., optical illusions and humans' difficulty in predicting what will make us the happiest) in human intelligence that are there because we are evolved beings rather than designed beings.
posted August 21, 2007 09:52 AM in Thinking Aloud | permalink | Comments (22)
August 04, 2007
Milgram's other experiment
In my line of work, Stanley Milgram is best remembered for his small-world experiment. But in other circles, he's better known for his work on human obedience. This seminal study was done back when scientific brilliance was unfettered by pesky rules about ethics, but thanks to the wonders of YouTube, you can share in his joyous discovery of how presumably good, or maybe at least "normal" (whatever that means), people can be made to do thing they know to be terrible when instructed by a superior. (In fact, it is partially because of this experiment that science now has an internal review mechanism to prevent such psychologically traumatic experiments from being conducted.) Here is Milgram writing in his 1974 article "The Perils of Obedience" (reproduced here) on the results of his study:
The legal and philosophic aspects of obedience are of enormous importance, but they say very little about how most people behave in concrete situations. I set up a simple experiment at Yale University to test how much pain an ordinary citizen would inflict on another person simply because he was ordered to by an experimental scientist. Stark authority was pitted against the subjects' [participants'] strongest moral imperatives against hurting others, and, with the subjects' [participants'] ears ringing with the screams of the victims, authority won more often than not. The extreme willingness of adults to go to almost any lengths on the command of an authority constitutes the chief finding of the study and the fact most urgently demanding explanation.
posted August 4, 2007 04:04 PM in Scientifically Speaking | permalink | Comments (2)
June 28, 2007
Hacking microbiology
Two science news articles (here and here) about J. Craig Venter's efforts to hack the genome (or perhaps, more broadly, hacking microbiology) reminded me of a few other articles about his goals. The two articles I ran across today concern a rather cool experiment where scientists took the genome of one bacterium species (M. mycoides) and transplanted it into a closely related one (M. capricolum). The actual procedure by which they made the genome transfer seems rather inelegant, but the end result is that the donor genome replaced the recepient genome and was operating well enough that the recepient looked like the donor. (Science article is here.) As a proof of concept, this experiment is a nice demonstration that the cellular machinery of the the recepient species is similar enough to that of the donor that it can run the other's genomic program. But, whether or not this technique can be applied to other species is an open question. For instance, judging by the difficulties that research on cloning has encountered with simply transferring a nucleus of a cell into an unfertilized egg of the same species, it seems reasonable to expect that such whole-genome transfers won't be reprogramming arbitrary cells any time in the foreseeable future.
The other things I've been meaning to blog about are stories I ran across earlier this month, also relating to Dr. Venter's efforts to pioneer research on (and patents for) genomic manipulation. For instance, earlier this month Venter's group filed a patent on an "artificial organism" (patent application is here; coverage is here and here). Although the bacterium (derived from another cousin of the two mentioned above, called M. genitalium) is called an artificial organism (dubbed M. laboratorium), I think that gives Venter's group too much credit. Their artificial organism is really just a hobbled version of its parent species, where they removed many of the original genes that were not, apparently, always necessary for a bacterium's survival. From the way the science journalism reads though, you get the impression that Venter et al. have created a bacterium from scratch. I don't think we have either the technology or the scientific understanding of how life works to be able to do that yet, nor do I expect to see it for a long time. But, the idea of engineering bacteria to exhibit different traits (maybe useful traits, such as being able to metabolize some of the crap modern civilizations produce) is already a reality and I'm sure we'll see more work along these lines.
Finally, Venter gave a TED talk in 2005 about his trip to sample the DNA of the ocean at several spots around the world. This talk is actually more about the science (or more pointedly, about how little we know about the diversity of life, as expressed through genes) and less about his commercial interests. It appears that some of the research results from this trip have already appeared on PLoS Biology.
I think many people love to hate Venter, but you do have to give him credit for having enormous ambition, and for, in part, spurring the genomics revolution currently gripping microbiology. Perhaps like many scientists, I'm suspicious of his commercial interests and find the idea of patenting anything about a living organism to be a little absurd, but I also think we're fast approaching the day when we putting bacteria to work doing things that we currently do via complex (and often dirty) industrial processes will be an everyday thing.
posted June 28, 2007 04:13 PM in Things that go squish | permalink | Comments (1)
June 08, 2007
Power laws and all that jazz
With apologies to Tolkien:
Three Power Laws for the Physicists, mathematics in thrall,
Four for the biologists, species and all,
Eighteen behavioral, our will carved in stone,
One for the Dark Lord on his dark throne.
In the Land of Science where Power Laws lie,
One Paper to rule them all, One Paper to find them,
One Paper to bring them all and in their moments bind them,
In the Land of Science, where Power Laws lie.
From an interest that grew directly out of my work chracterizing the frequency of severe terrorist attacks, I'm happy to say that the review article I've been working on with Cosma Shalizi and Mark Newman -- on accurately characterizing power-law distributions in empirical data -- is finally finished. The paper covers all aspects of the process, from fitting the distribution to testing the hypothesis that the data is distributed according to a power law, and to make it easy for folks in the community to use the methods we recommend, we've also made our code available.
So, rejoice, rejoice all ye people of Science! Go forth, fit and validate your power laws!
For those still reading, I have a few thoughts about this paper now that it's been released into the wild. First, I naturally hope that people read the paper and find it interesting and useful. I also hope that we as a community start asking ourselves what exactly we mean when we say that such-and-such a quantity is "power-law distributed," and whether our meaning would be better served at times by using less precise terms such as "heavy-tailed" or simply "heterogeneous." For instance, we might simply mean that visually it looks roughly straight on a log-log plot. To which I might reply (a) power-law distributions are not the only thing that can do this, (b) we haven't said what we mean by roughly straight, and (c) we haven't been clear about why we might prefer a priori such a form over alternatives.
The paper goes into the first two points in some detail, so I'll put those aside. The latter point, though, seems like one that's gone un-addressed in the literature for some time now. In some cases, there are probably legitimate reasons to prefer an explanation that assumes large events (and especially those larger than we've observed so far) are distributed according to a power law -- for example, cases where we have some convincing theoretical explanations that match the microscopic details of the system, are reasonably well motivated, and whose predictions have held up under some additional tests. But I don't think most places where power-law distributions have been "observed" have this degree of support for the power-law hypothesis. (In fact, most simply fit a power-law model and assume that it's correct!) We also rarely ask why a system necessarily needs to exhibit a power-law distribution in the first place. That is, would the system behave fundamentally differently, perhaps from a functional perspective, if it instead exhibited a log-normal distribution in the upper tail?
Update 15 June: Cosma also blogs about the paper, making many excellent points about the methods we describe for dealing with data, as well as making several very constructive points about the general affair of power-law research. Well worth the time to read.
posted June 8, 2007 10:00 AM in Complex Systems | permalink | Comments (3)
May 27, 2007
DS07
This week, I'm in Snowbird, UT for SIAM's conference on Applications of Dynamical Systems (DS07). I'm here for a mini-symposium on complex networks organized by Mason Porter and Peter Mucha. I'll be blogging about these (and maybe other) network sessions as time allows (I realize that I still haven't blogged about NetSci last week - that will be coming soon...).
posted May 27, 2007 11:38 PM in Scientifically Speaking | permalink | Comments (1)
May 21, 2007
NetSci 2007
This week, I'm in New York City for the International Conference on Network Science, being held at the New York Hall of Science Museum in Queens. I may not be able to blog each day about the events, but I'll be posting my thoughts and comments as things progress. Stay tuned. In the meantime, here's the conference talk schedule.
posted May 21, 2007 11:41 AM in Scientifically Speaking | permalink | Comments (0)
IPAM - Random and Dynamic Graphs and Networks (Days 4 & 5)
Two weeks ago, I was in Los Angeles for the Institute for Pure and Applied Mathematics' (IPAM, at UCLA) workshop on Random and Dynamic Graphs and Networks; this is the fourth and fifth entry.
Rather than my usual format of summarizing the things that got me thinking during the last few days, I'm going to go with a more free-form approach.
Thursday began with Jennifer Chayes (MSR) discussing some analytical work on adapting convergence-in-distribution proof techniques to ensembles of graphs. She introduced the cut-norm graph distance metric (useful on dense graphs; says that they have some results for sparse graphs, but that it's more difficult for those). The idea of graph distance seems to pop up in many different areas (including several I've been thinking of) and is closely related to the GRAPH ISOMORPHISM problem (which is not known to be NP-complete, but nor is it known to be in P). For many reasons, it would be really useful to be able to calculate in polynomial time the minimum edge-edit distance between two graphs; this would open up a lot of useful techniques based on transforming one graph into another.
Friday began with a talk by Jeannette Janssen (Dalhousie University) on a geometric preferential attachment model, which is basically a geometric random graph but where nodes have a sphere of attraction (for new edges) that has volume proportional to the node's in-degree. She showed some very nice mathematical results on this model. I wonder if this idea could be generalized to arbitrary manifolds (with a distance metric on them) and attachment kernels. That is, imagine that our complex network has actually imbedded on some complicated manifold and the attachment is based on some function of the distance on that manifold between the two nodes. The trick would be then to infer both the structure of the manifold and the attachment function from real data. Of course, without some constraints on both features, it would be easy to construct an arbitrary pair (manifold and kernel) that would give you exactly the network you observed. Is it sufficient to get meaningful results that both should be relatively smooth (continuous, differentiable, etc.)?
Jeannette's talk was followed by Filippo Menczer's talk on mining traffic data from the Internet2/Abilene network. The data set was based on daily dumps of end-to-end communications (packet headers with client and server IDs anonymized) and looked at a variety of behaviors of this traffic. He used this data to construct interaction graphs betwen clients and servers, clients and applications (e.g., "web"), and a few other things. The analysis seems relatively preliminary in the sense that there are a lot of data issues that are lurking in the background (things like aggregated traffic from proxies, aliasing and masking effects, etc.) that make it difficult to translate conclusions about the traffic into conclusions about real individual users. But, fascinating stuff, and I'm looking forward to seeing what else comes out of that data.
The last full talk I saw was by Raissa D'Souza on competition-induced preferential attachment, and a little bit at the end on dynamic geometric graphs and packet routing on them. I've seen the technical content of the preferential attachment talk before, but it was good to have the reminder that power-law distributions are not necessarily the only game in town for heavy-tailed distributions, and that even though the traditional preferential attachment mechanism may not be a good model of the way real growing networks change, it may be that another mechanism that better models the real world can look like preferential attachment. This ties back to Sidney Redner's comment a couple of days before about the citation network: why does the network look like one grown by preferential attachment, when we know that's not how individual authors choose citations?
posted May 21, 2007 11:38 AM in Scientifically Speaking | permalink | Comments (4)
May 09, 2007
IPAM - Random and Dynamic Graphs and Networks (Day 3)
This week, I'm in Los Angeles for the Institute for Pure and Applied Mathematics' (IPAM, at UCLA) workshop on Random and Dynamic Graphs and Networks; this is the third of five entries based on my thoughts from each day. As usual, these topics are a highly subjective slice of the workshop's subject matter...
The impact of mobility networks on the worldwide spread of epidemics
I had the pleasure of introducing Alessandro Vespignani (Indiana University) for the first talk of the day on epidemics in networks, and his work in modeling the effect that particles (people) moving around on the airport network have on models of the spread of disease. I've seen most of this stuff before from previous versions of Alex's talk, but there were several nice additions. The one that struck the audience the most was a visualization of all of the individual flights over the space of a couple of days in the eastern United States; the animation was made by Aaron Koblin for a different project, but was still quite effective in conveying the richness of the air traffic data that Alex has been using to do epidemic modeling and forecasting.
On the structure of growing networks
Sidney Redner gave the pre-lunch talk about his work on the preferential attachment growing-network model. Using the master equation approach, Sid explored an extremely wide variety of properties of the PA model, such as the different regimes of degree distribution behavior for sub-, exact, and different kinds of super- linear attachment rates, the first-mover advantage in the network, the importance of initial degree in determining final degree, along with several variations on the initial model. The power of the master equation approach was clearly evident, I should really learn more about.
He also discussed his work analyzing 100 years of citation data from the Physical Review journal (about 350,000 papers and 3.5 million citations; in 1890, the average number of references in a paper was 1, while in 1990, the average number had increased to 10), particularly with respect to his trying to understand the evidence for linear preferential attachment as a model of citation patterns. Quite surprisingly, he showed that for the first 100 or so citations, papers in PR have nearly linear attachment rates. One point Sid made several times in his talk is that almost all of the results for PA models are highly sensitive to variations in the precise details of the attachment mechanism, and that it's easy to get something quite different (so, no power laws) without trying very hard.
Finally, a question he ended with is why does linear PA seem to be a pretty good model for how citations acrue to papers, even though real citation patterns are clearly not dictated by the PA model?
Panel discussion
The last talk-slot of the day was replaced by a panel discussion, put together by Walter Willinger and chaired by Mark Newman. Instead of the usual situation where the senior people of a field sit on the panel, this panel was composed of junior people (with the expectation that the senior people in the audience would talk anyway). I was asked to sit on the panel, along with Ben Olding (Harvard), Lea Popovic (Cornell), Leah Shaw (Naval Research Lab), and Lilit Yeghiazarian (UCLA). We each made a brief statement about what we liked about the workshop so far, and what kinds of open questions we would be most interested in seeing the community study.
For my on part, I mentioned many of the questions and themes that I've blogged about the past two days. In addition, I pointed out that function is more than just structure, being typically structure plus dynamics, and that our models currently do little to address the dynamics part of this equation. (For instance, can dynamical generative models of particular kinds of structure tell us more about why networks exhibit those structures specifically, and not some other variety?) Lea and Leah also emphasized dynamics as being a huge open area in terms of both modeling and mechanisms, with Lea pointing out that it's not yet clear what are the right kinds of dynamical processes that we should be studying with networks. (I made a quick list of processes that seem important, but only came up with two main caterogies, branching-contact-epidemic-percolation processes and search-navigation-routing processes. Sid later suggested that consensus-voting style processes, akin to the Ising model, might be another, although there are probably others that we haven't thought up yet.) Ben emphasized the issues of sampling, for instance, sampling subgraphs of our model, e.g., the observable WWW or even just the portion we can crawl in an afternoon, and dealing with sampling effects (i.e., uncertainty) in our models.
The audience had a lot to say on these and other topics, and particularly so on the topics of what statisticians can contribute to the field (and also why there are so few statisticians working in this area; some suggestions that many statisticians are only interested in proving asymptotic results for methods, and those that do deal with data are working on bio-informatics-style applications), and on the cultural difference between the mathematicians who want to prove nice things about toy models (folks like Christian Borgs, Microsoft Research) as a way of understanding the general propeties of networks and of their origin, and the empiricists (like Walter Willinger) who want accurate models of real-world systems that they can use to understand their system better. Mark pointed out that there's a third way in modeling, which relies on using an appropriately defined null model as a probe to explore the structure of your network, i.e., a null model that reproduces some of the structure you see in your data, but is otherwise maximally random, can be used to detect the kind of structure the model doesn't explain (so-called "modeling errors", in contrast to "measurement errors"), and thus be used in the standard framework of error modeling that science has used successfully in the past to understand complex systems.
All-in-all, I think the panel discussion was a big success, and the conversation certainly could have gone on well past the one-hour limit that Mark imposed.
posted May 9, 2007 11:38 PM in Scientifically Speaking | permalink | Comments (0)
May 08, 2007
IPAM - Random and Dynamic Graphs and Networks (Day 2)
This week, I'm in Los Angeles for the Institute for Pure and Applied Mathematics' (IPAM, at UCLA) workshop on Random and Dynamic Graphs and Networks; this is the second of five entries based on my thoughts from each day. As usual, these topics are a highly subjective slice of the workshop's subject matter...
Biomimetic searching strategies
Massimo Vergassola (Institut Pasteur) started the day with an interesting talk that had nothing to do with networks. Massimo discussed the basic problem of locating a source of smelly molecules in the macroscopic world where air currents cause pockets of the smell to be sparsely scattered across a landscape, thus spoiling the chemotaxis (gradient ascent) strategy used by bacteria, and a clever solution for it (called "infotaxis") based on trading off exploration and exploitation via an adaptive entropy minimization strategy.
Diversity of graphs with highly variable connectivity
Following lunch, David Alderson (Naval Postgraduate School) described his work with Lun Li (Caltech) on understanding just how different networks with a given degree distribution can be from each other. The take-home message of Dave's talk is, essentially, that the degree distribution is a pretty weak constraint on other patterns of connectivity, and is not a sufficient statistical characterization of the global structure of the network with respect to many (most?) of the other (say, topological and functional) aspects we might care about. Although he focused primarily on degree assortativity, the same kind of analysis could in principle be done for other network measures (clustering coefficient, distribution, diameter, vertex-vertex distance distribution, etc.), none of which are wholly independent of the degree distribution, or of each other! (I've rarely seen the interdependence of these measures discussed (mentioned?) in the literature, even though they are often treated as such.)
In addition to describing his numerical experiments, Dave sounded a few cautionary notes about the assumptions that are often made in the complex networks literature (particularly by theoreticians using random-graph models) on the significance of the degree distribution. For instance, the configration model with a power-law degree sequence (and similarly, graphs constructed via preferential attachment) yields networks that look almost nothing like any real-world graph that we know, except for making vaguely similar degree distributions, and yet they are often invoked as reasonable models of real-world systems. In my mind, it's not enough to simply fix-up our existing random-graph models to instead define an ensemble with a specific degree distribution, and a specific clustering coefficient, and a diameter, or whatever our favorite measures are. In some sense all of these statistical measures just give a stylized picture of the network, and will always be misleading with respect to other important structural features of real-world networks. For the purposes of proving mathematical theorems, I think these simplistic toy models are actually very necessary -- since their strong assumptions make analytic work significantly easier -- so long as we also willfully acknowledge that they are a horrible model of the real world. For the purposes of saying something concrete about real networks, we need more articulate models, and, probably, models that are domain specific. That is, I'd like a model of the Internet that respects the idiosyncracies of this distributed engineered and evolving system; a model of metabolic networks that respects the strangeness of biochemistry; and a model of social networks that understands the structure of individual human interactions. More accurately, we probably need models that understand the function that these networks fulfill, and respect the dynamics of the network in time.
Greedy search in social networks
David Liben-Nowell (Carleton College) then closed the day with a great talk on local search in social networks. The content of this talk largely mirrored that of Ravi Kumar's talk at GA Tech back in January, which covered an empirical study of the distribution of the (geographic) distance covered by friendship links in the LiveJournal network (from 2003, when it had about 500,000 users located in the lower 48 states). This work combined some nice data analysis with attempts to validate some of the theoretical ideas due to Kleinberg for locally navigable networks, and a nice generalization of those ideas to networks with non-uniform population distributions.
An interesting point that David made early in his talk was that homophily is not sufficient to explain the presense of either the short paths that Milgrams' original 6-degrees-of-seperation study demonstrated, or even the existence of a connected social graph! That is, without a smoothly varying notion of "likeness", then homophily would lead us to expect disconnected components in the social network. If both likeness and the population density in the likeness space varied smoothly, then a homophilic social web would cover the space, but the average path length would be long, O(n). In order to get the "small world" that we actually observe, we need some amount of non-homophilic connections, or perhaps multiple kinds of "likeness", or maybe some diversity in the preference functions that individuals use to link to each other. Also, it's still not clear what mechanism would lead to the kind of link-length distribution predicted by Kleinberg's model of optimally navigable networks - an answer to this question would, presumably, tell us something about why modern societies organize themselves the way they do.
posted May 8, 2007 10:52 PM in Scientifically Speaking | permalink | Comments (0)
May 07, 2007
IPAM - Random and Dynamic Graphs and Networks (Day 1)
This week, I'm in Los Angeles for the Institute for Pure and Applied Mathematics' (IPAM, at UCLA) workshop on random and dynamic graphs and networks. This workshop is the third of four in their Random Shapes long program. The workshop has the usual format, with research talks throughout the day, punctuated by short breaks for interacting with your neighbors and colleagues. I'll be trying to do the same for this event as I did for the DIMACS workshop I attended back in January, which is to blog each day about interesting ideas and topics. As usual, this is a highly subjective slice of the workshop's subject matter.
Detecting and understanding the large-scale structure of networks
Mark Newman (U. Michigan) kicked off the morning by discussing his work on clustering algorithms for networks. As he pointed out, in the olden days of network analysis (c. 30 years ago), you could write down all the nodes and edges in a graph and understand its structure visually. These days, our graphs are too big for this, and we're stuck using statistical probes to understand how these things are shaped. And yet, many papers include figures of networks as incoherent balls of nodes and edges (Mark mentioned that Marc Vidal calls these figures "ridiculograms").
I've seen the technical content of Mark's talk before, but he always does an excellent job of making it seem fresh. In this talk, there was a brief exchange with the audience regarding the NP-completeness of the MAXIMUM MODULARITY problem, which made me wonder what exactly are the kind of structures that would make an instance of the MM problem so hard. Clearly, polynomial time algorithms that approximate the maximum modularity Q exist because we have many heuristics that work well on (most) real-world graphs. But, if I was an adversary and wanted to design a network with particularly difficult structure to partition, what kind would I want to include? (Other than reducing another NPC problem using gadgets!)
Walter Willinger raised a point here (and again in a few later talks) about the sensitivity of most network analysis methods to topological uncertainty. That is, just about all the techniques we have available to us assume that the edges as given are completely correct (no missing or spurious edges). Given the classic result due to Watts and Strogatz (1998) of the impact that a few random links added to a lattice have on the diameter of the graph, it's clear that in some cases, topological errors can have a huge impact on our conclusions about the network. So, developing good ways to handle uncertainty and errors while analyzing the structure of a network is a rather large, gaping hole in the field. Presumably, progress in this area will require having good error models of our uncertainty, which, necessary, depend on the measurement techniques used to produce the data. In the case of traceroutes on the Internet, this kind of inverse problem seems quite tricky, but perhaps not impossible.
Probability and Spatial Networks
David Aldous (Berkeley) gave the second talk and discussed some of his work on spatial random graphs, and, in particular, on the optimal design and flow through random graphs. As an example, David gave us a simple puzzle to consider:
Given a square of area N with N nodes distributed uniformly at random throughout. Now, subdivided this area into L^2 subsquares, and choose one node in each square to be a "hub." Then, connect each of the remaining nodes in a square to the hub, and connect the hubs together in a complete graph. The question is, what is the size L that minimizes the total (Euclidean) length of the edges in this network?
He then talked a little about other efficient ways to connect up uniformly scattered points in an area. In particular, Steiner trees are the standard way to do this, and have a cost O(N). The downside for this efficiency is that the tree-distance between physically proximate points on the plane is something polynomial in N (David suggested that he didn't have a rigorous proof for this, but it seems quite reasonable). As it turns out, you can dramatically lower this cost by adding just a few random lines across the plane -- the effect is analagous to the one in the Watts-Strogatz model. Naturally, I was thinking about the structure of real road networks here, and it would seem that the effect of highways in the real world is much the same as David's random lines. That is, it only takes a few of these things to dramatically reduce the topological distance between arbitrary points. Of course, road networks have other things to worry about, such as congestion, that David's highways don't!
posted May 7, 2007 11:49 PM in Scientifically Speaking | permalink | Comments (1)
March 30, 2007
The difference
Via xkcd: "How could you choose avoiding a little pain over understanding a magic lightning machine?" So true. So true...
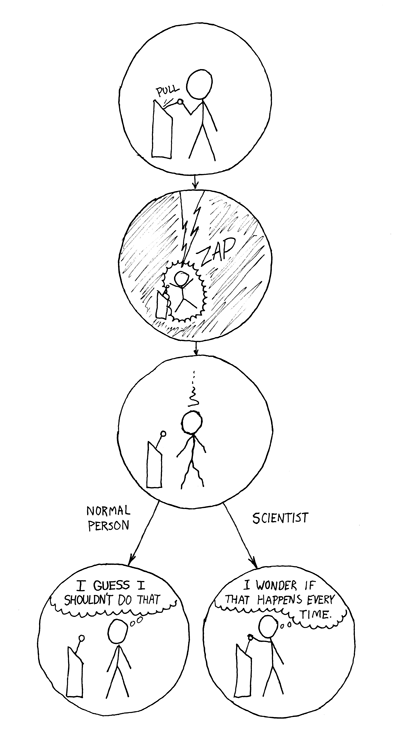
posted March 30, 2007 10:16 AM in Humor | permalink | Comments (3)
March 25, 2007
The kaleidoscope in our eyes
Long-time readers of this blog will remember that last summer I received a deluge of email from people taking the "reverse" colorblind test on my webpage. This happened because someone dugg the test, and a Dutch magazine featured it in their 'Net News' section. For those of you who haven't been wasting your time on this blog for quite that long, here's a brief history of the test:
In April of 2001, a close friend of mine, who is red-green colorblind, and I were discussing the differences in our subjective visual experiences. We realized that, in some situations, he could perceive subtle variations in luminosity that I could not. This got us thinking about whether we could design a "reverse" colorblindness test - one that he could pass because he is color blind, and one that I would fail because I am not. Our idea was that we could distract non-colorblind people with bright colors to keep them from noticing "hidden" information in subtle but systematic variations in luminosity.
Color blind is the name we give to people who are only dichromatic, rather than the trichromatic experience that 'normal' people have. This difference is most commonly caused by a genetic mutation that prevents the colorblind retina from producing more than two kinds of photosensitive pigment. As it turns out, most mammals are dichromatic, in roughly the same way that colorblind people are - that is, they have a short-wave pigment (around 400 nm) and a medium-wave pigment (around 500 nm), giving them one channel of color contrast. Humans, and some of our closest primate cousins, are unusual for being trichromatic. So, how did our ancestors shift from being di- to tri-chromatic? For many years, scientists have believed that the gene responsible for our sensitivity in the green part of the spectrum (530 nm) was accidentally duplicated and then diverged slightly, producing a second gene yielding sensitivity to slightly longer wavelengths (560 nm; this is the red-part of the spectrum. Amazingly, the red-pigment differs from the green by only three amino acids, which is somewhere between 3 and 6 mutations).
But, there's a problem with this theory. There's no reason a priori to expect that a mammal with dichromatic vision, who suddenly acquired sensitivity to a third kind of color, would be able to process this information to perceive that color as distinct from the other two. Rather, it might be the case that the animal just perceives this new range of color as being one of the existing color sensations, so, in the case of picking up a red-sensitive pigment, the animal might perceive reds as greens.
As it turns out, though, the mammalian retina and brain are extremely flexible, and in an experiment recently reported in Science, Jeremy Nathans, a neuroscientist at Johns Hopkins, and his colleagues show that a mouse (normally dichromatic, with one pigment being slightly sensitive to ultraviolet, and one being very close to our medium-wave, or green sensitivity) engineered to have the gene for human-style long-wave or red-color sensitivity can in fact perceive red as a distinct color from green. That is, the normally dichromatic retina and brain of the mouse have all the functionality necessary to behave in a trichromatic way. (The always-fascinating-to-read Carl Zimmer, and Nature News have their own takes on this story.)
So, given that a dichromatic retina and brain can perceive three colors if given a third pigment, and a trichromatic retina and brain fail gracefully if one pigment is removed, what is all that extra stuff (in particular, midget cells whose role is apparently to distinguish red and green) in the trichromatic retina and brain for? Presumably, enhanced dichromatic vision is not quite as good as natural trichromatic vision, and those extra neural circuits optimize something. Too bad these transgenic mice can't tell us about the new kaleidoscope in their eyes.
But, not all animals are dichromatic. Birds, reptiles and teleost fish are, in fact, tetrachromatic. Thus, after mammals branched off from these other species millions of years ago, they lost two of these pigments (or, opsins), perhaps during their nocturnal phase, where color vision is less functional. This variation suggests that, indeed, the reverse colorblind test is based on a reasonable hypothesis - trichromatic vision is not as sensitive to variation in luminosity as dichromatic vision is. But why might a deficient trichromatic system (retina + brain) would be more sensitive to luminal variation than a non-deficient one? Since a souped-up dichromatic system - the mouse experiment above - has most of the functionality of a true trichromatic system, perhaps it's not all that surprising that a deficient trichromatic system has most of the functionality of a true dichromatic system.
A general explanation for both phenomena would be that the learning algorithms of the brain and retina organize to extract the maximal amount of information from the light coming into the eye. If this happens to be from two kinds of color contrast, it optimizes toward taking more information from luminal variation. It seems like a small detail to show scientifically that a deficient trichromatic system is more sensitive to luminal variation than a true trichromatic system, but this would be an important step to understanding the learning algorithm that the brain uses to organize itself, developmentally, in response to visual stimulation. Is this information maximization principle the basis of how the brain is able to adapt to such different kinds of inputs?
G. H. Jacobs, G. A. Williams, H. Cahill and J. Nathans, "Emergence of Novel Color Vision in Mice Engineered to Express a Human Cone Photopigment", Science 315 1723 - 1725 (2007).
P. W. Lucas, et al, "Evolution and Function of Routine Trichromatic Vision in Primates", Evolution 57 (11), 2636 - 2643 (2003).
posted March 25, 2007 10:51 AM in Evolution | permalink | Comments (3)
March 21, 2007
Structuring science; or: quantifying navel-gazing and self-worth
Like most humans, scientists are prone to be fascinated by themselves, particularly in large groups, or when they need to brag to each other about their relative importance. I want to hit both topics, but let me start with the latter.
In many ways, the current academic publishing system aggravates some of the worst behavior scientists can exhibit (with respect to doing good science). For instance, the payoff for getting an article published in one of a few specific high-profile journals [1] is such that - dare I say it - weak-minded scientists may overlook questionable scientific practices, make overly optimistic interpretations the significance of their results, or otherwise mislead the reviewers about the quality of the science [2,3].
While my sympathies lie strongly with my academic friends who want to burn the whole academic publishing system to the ground in a fit of revolutionary vigor, I'm reluctant to actually do so. That is, I suppose that, at this point, I'm willing to make this faustian bargain for the time I save by getting a quick and dirty idea of a person's academic work by looking at the set of journals in which they're published [4]. If only I were a more virtuous person [5] - I would then read each paper thoroughly, at first ignoring the author list, in order to gauge its quality and significance, and, when it was outside of my field, I would recuse myself from the decision entirely or seek out a knowledgeable expert.
Which brings me to one of the main points of this post - impact factors - and how horrible they are [6]. Impact factors are supposed to give a rough measure of the scientific community's appraisal of the quality of a journal, and, by extension, the articles that appear in that journal. Basically, it's the the average number of citations received by an article (as tracked by the official arbiter of impact, ISI/Thompson), divided by the number of articles published in that journal, or something. So, obviously review journals have a huge impact because they publish only a few papers that are inevitably cited by everyone. The problem with this proxy for significance is that it assumes that all fields have similar citation patterns and that all fields are roughly the same size, neither of which is even remotely true. These assumptions explain why a middling paper in a medical journal seems to have a larger impact than a very good paper in, say, physics - the number of people publishing in medicine is about an order of magnitude greater than the number of physicists, and the number of references per paper is larger, too. When these bibliometrics are used in hiring and funding decisions, or even decisions about how to do, how to present, where to submit, and how hard to fight for your research, suddenly these quirks start driving the behavior of science itself, rather than the other way around.
Enter eigenfactor (from Carl Bergstrom's lab), a way of estimating journal impact that uses the same ideas that Google (or, more rightly, PageRank, or, even more rightly, A. A. Markov) uses to give accurate search results. The formulation of a journal's significance in terms of Markov chains is significantly better than impact factors, and is significantly more objective than the ranking we store in our heads. For instance, this method captures our intuitive notion about the significance of review articles - that is, they may garner many citations by defining the standard practices and results of an area, but they consequently cite very many articles to so, and thus their significance is moderated. But, this obsession with bibliometrics as a proxy for scientific quality is still dangerous, as some papers are highly cited because they are not good science! (tips to Cosma Shalizi, and to Suresh)
The other point I wanted to mention in this post is the idea of mapping the structure of science. Mapping networks is a topic I have a little experience with, and I feel comfortable saying that your mapping tools have a strong impact on the kind, and quality, of conclusions you make about that structure. But, if all we're interested in is making pretty pictures, have at it! Seed Magazine has a nice story about a map of science constructed from citation patterns of roughly 800,000 articles [7] (that's a small piece of it to the left; pretty). Unsurprisingly, you can see that certain fields dominate this landscape, and I'm sure that some of that dominance is due to the same problems with impact factors that I mentioned above, such as using absolute activity and other first-order measures of impact, rather than integrating over the entire structure. Of course, there's some usefulness in looking at even dumb measures, so long as you remember what they leave out. (tip to Slashdot)
-----
[1] I suppose the best examples of this kind of venue are the so-called vanity journals like Nature and Science.
[2] The ideal, of course, is a very staid approach to research, in which the researcher never considers the twin dicta of tenure: publish-or-perish and get-grants-or-perish, or the social payoff (I'm specifically thinking of prestige and attention from the media) for certain kinds of results. Oh that life were so perfect, and science so insulated from the messiness of human vice! Instead, we get bouts of irrational exuberance like polywater and other forms of pathological science. Fortunately, there can be a scientific payoff for invalidating such high-profile claims, and this (eventual) self-correcting tendency is both one of the saving graces of scientific culture, and one of the things that distinguishes it from non-sciences.
[3] There have been a couple of high-profile cases of this kind of behavior in the scientific press over the past few years. The whole cloning fiasco over Hwang Woo-Suk's work is probably the best known of these (for instance, here).
[4] I suppose you could get a similar idea by looking at the institutions listed on the c.v., but this is probably even less accurate than the list of journals.
[5] To be fair, pre-print systems like arXiv are extremely useful (and novel) in that force us to be more virtuous about evaluating the quality of individual papers. Without the journal tag to (mis)lead, we're left having to evaluate papers by other criteria, such as the abstract, or - gasp! - the paper itself. (I imagine the author list is also frequently used.) I actually quite like this way of learning about papers, because I fully believe that our vague ranking of academic journals is so firmly ingrained in our brains, that it strongly biases our opinions of papers we read. "Oh, this appeared in Nature, it must be good!" Bollocks.
[6] The h-index, or Hirsch number is problematic for similar reasons. A less problematic version would be to integrate over the whole network of citations, rather than simply looking at first-order citation patterns. If we would only truly embrace the arbitrariness of these measures, we would all measure our worth by our Erdos numbers, or some other demigod-like being.
[7] The people who created the map are essentially giving away large versions of it to anyone willing to pay shipping and handling.
posted March 21, 2007 11:07 AM in Scientifically Speaking | permalink | Comments (0)
January 31, 2007
My kingdom for a good null-model
The past few days, I've been digging into the literature on extreme value theory, which is a rather nice branch of probability theory that shows how the distribution of the largest (or, smallest) observed value varies. This exercise has been mostly driven by a desire to understand how it connects to my own research on power-law distributions (I'm reluctant to admit that I'm actually working on a lengthy review article on the topic, partially in an attempt to clear up what seems to be substantial confusion over both their significance and how to go about measuring them in real data). But, that's a topic for a future post. What I really want to mention is an excellent example of good statistical reasoning in experimental high energy physics (hep), as related by Prof. John Conway over at CosmicVariance. Conway is working on the CDF experiment (at Fermilab), and his story kicks off with the appropriate quip "Was it real?" The central question Conway faces is whether or not a deviation / fluctuation in his measurements is significant. If it is, then it's evidence for the existence of a particular particle called the Higgs boson - a long sought-after component of the Standard Model of particle physics. If not, then it's back to searching for the Higgs. What I liked most about Conway's post is the way the claims of significance - the bump is real - are carefully vetted against both theroetical expectations of random fluctuations and a desire to not over-hype the potential for discovery.
In the world of networks (and power laws), the question of "Is it real?" is one that I wish was asked more often. When looking at complex network structure, we often want to know whether a pattern or the value of some statistical measure could have been caused by chance. Crucially, though, our ability to answer this question depends on our model of chance itself - this point is identical to the one that Conway faces, however, for hep experiments, the error models are substantially more precise than what we have for complex networks. Historically, network theorists have used either the Erdos-Renyi random graph or the configuration model (see cond-mat/0202208) as the model of chance. Unfortunately, neither of these look anything like the real-world, and thus probably provide terrible over-estimates of the significance of any particular network pattern. As a modest proposal, I suggest that hierarchical random graphs (HRGs) seem to serve as a more robust null-model, since they can capture a wide range of the heterogeneity that we observe in the real-world, e.g., community structure, skewed degree distribution, high clustering coefficient, etc. The real problem, of course, is that a good null-model depends heavily on what kind of question is being asked. In the hep experiment, we know enough about what the results would look like without the Higgs that, if it does exist, then we'd see large (i.e., statistically large) fluctuations at a specific location in the distribution.
Looking forward, the general problem of coming up with good null-models of network structure, against which we can reasonably benchmark our measurements and their deviations from our theoretical expectations, is hugely important, and I'm sure it will become increasingly so as we delve more deeply into the behavior of dynamical processes that run on top of a network (e.g., metabolism or signaling). For instance, what would a reasonable random-graph model of a signaling network look like? And, how can we know if the behavior of a real-world signaling network is within statistical fluctuations of its normal behavior? How can we tell whether two metabolic networks are significantly different from each other, or whether their topology is identical up to a small amount of noise? Put another way, how can we tell when a metabolic network has made a significant shift in its behavior or struture as a result of natural selection? One could even phrase the question of "What is a species?" as a question of whether the difference between two organisms is within statistical fluctuations of a cannonical member of the species.
posted January 31, 2007 12:25 AM in Scientifically Speaking | permalink | Comments (0)
January 27, 2007
Fish are the new birds?
Given my apparent fascination with bird brains, and their evident ability to functionally imitate mammalian brains, imagine my surprise to discover that fish (specifically the males of a species of cichlid called A. burtoni) employ similar logical inference techniques to birds and mammals. The experimental setup allowed a bystander cichlid to observe fights between five others, through which a social hierarchy of A > B > C > D > E was constructed. In subsequent pairings between the bystander and the members of the hierarchy, the bystander preferred pairing with the losers in the hierarchy, i.e., near E and D. The idea is that the bystander is hedging his bet on where he stands in the hierarchy by preferring to fight losers over winners.
One interesting implication of this study is that logical inference - in this case something called "transitive inference", which allows the user to use chains of relationships to infer additional relationships that shortcut the chain, e.g., A > B and B > C implies A > C - maybe have evolved extremely early in the history of life; alternatively, it could be that the ability to do logical inference is something that brains can acquire relatively quickly when natural selection favors it slightly. In the case of the cichlids, it may be that the development of transitive inference evolved in tandem with their becoming highly territorial.
I wonder what other cerebral capabilities fish and birds have in common...
L. Grosenick, T. S. Clement and R. D. Fernald, "Fish can infer social rank by observation alone." Nature 445, 429 (2007).
posted January 27, 2007 12:43 PM in Obsession with birds | permalink | Comments (2)
January 25, 2007
DIMACS - Complex networks and their applications (Day 3)
The third day of the workshop focused on applications to biochemical networks (no food webs), with a lot of that focus being on the difficulties of taking fuzzy biological data (e.g., gene expression data) and converting it into an accurate and meaningful form for further analysis or for hypothesis testing. Only a few of the talks were theoretical, but this perhaps reflects the current distribution of focus in biology today. After the workshop was done, I wondered just how much information crossed between the various disciplines represented at the workshop - certainly, I came away from it with a few new ideas, and a few new insights from the good talks I attended. And I think that's the sign of a successful workshop.
Complex Networks in Biology
Chris Wiggins (Columbia) delivered a great survey of interesting connections between machine learning and biochemical networks. It's probably fair to say that biologists are interested in constructing an understanding of cellular-level systems that compares favorably to an electrical engineer's understanding of circuits (Pointer: Can a Biologist Fix a Radio?). But, this is hard because living stuff is messy, inconsistent in funny ways, and has a tendency to change while you're studying it. So, it's harder to get a clean view of what's going on under the hood than it was with particle physics. This, of course, is where machine learning is going to save us - ML offers powerful and principled ways to sift through (torture) all this data.
The most interesting part of his talk, I think, was his presentation of NetBoost, a mechanism discriminator that can tell you which (among a specific suite of existing candidates) is the most likely to have generated your observed network data [1]. For instance, was it preferential attachment (PA) or duplication-mutation-complementation (DMC) that produced a given protein-interaction network (conclusion: the latter is better supported). The method basically works by constructing a decision tree that looks at the subgraph decomposition of a network and scores it's belief that each of the various mechanisms produced it [2]. With the ongoing proliferation of network mechanisms (theorists really don't have enough to do these days), this kind of approach serves as an excellent way to test a new mechanism against the data it's supposed to be emulating.
One point Chris made that resonated strongly with me - and which Cris and Mark made yesterday - is the problem with what you might call "soft validation" [3]. Typically, a study will cluster or do some other kind of analysis with the data, and then tell a biological story about why these results make sense. On the other hand, forcing the clustering to make testable predictions would be a stronger kind of validation.
Network Inference and Analysis for Systems Biology
Just before lunch, Joel Bader (Johns Hopkins) gave a brief talk about his work on building a good view of the protein-protein interaction network (PPIN). The main problems with this widely studied data are the high error rate, both for false positives (interactions that we think exist, but don't) and false negatives (interactions that we think don't exist, but do). To drive home just how bad the data is, he pointed out that two independent studies of the human PPIN showed just 1% overlap in the sets of "observed" interactions.
He's done a tremendous amount of work on trying to improve the accuracy of our understanding of PPINs, but here he described a recent approach that fits degree-based generative models [4] to the data using our old friend expectation-maximization (EM) [5]. His results suggest that we're seeing about 30-40% of the real edges, but that our false positive rate is about 10-15%. This is a depressing signal-to-noise ratio (roughly 1%), because the number of real interactions is O(n), while our false positive rate is O(n^2). Clearly, the biological methods used to infer the interactions need to be improved before we have a clear idea of what this network looks like, but it also suggests that a lot of the previous results on this network are almost surely wrong. Another question is whether it's possible to incorporate these kinds of uncertainties into our analyses of the network structure.
Activating Interaction Networks and the Dynamics of Biological Networks
Meredith Betterton (UC-Boulder) presented some interesting work on signaling and regulatory networks. One of the more surprising tidbits she used in her motivation is the following. In yeast, the mRNA transcription undergoes a consistent 40-minute genome-wide oscillation, but when exposed to an antidepressant (in this case, phenelzine), the period doubles [6]. (The fact that gene expression oscillates like this poses another serious problem for the results of gene expression analysis that doesn't account for such oscillations.)
The point Meredith wanted to drive home, though, was we shouldn't just think of biochemical networks as static objects - they also represent the form that the cellular dynamics must follow. Using a simple dynamical model of activation and inhibition, she showed that the structure (who points to who, and whether an edge inhibits or activates its target) of a real-world circadian rhythm network and a real-world membrane-based signal cascade basically behave exactly as you would expect - one oscillates and the other doesn't. But, then she showed that it only takes a relatively small number of flips (activation to inhibition, or vice versa) to dramatically change the steady-state behavior of these cellular circuits. In a sense, this suggests that these circuits are highly adaptable, given a little pressure.
There are several interesting questions that came to mind while she was presenting. For instance, if we believe there are modules within the signaling pathways that accomplish a specific function, how can we identify them? Do sparsely connected dense subgraphs (assortative community structure) map onto these functional modules? What are the good models for understanding these dynamics, systems of differential equations, discrete time and matrix multiplication, or something more akin to a cellular version of Ohm's Law? [7]
-----
[1] M. Middendorf, E. Ziv and C. Wiggins, "Inferring Network Mechanisms: The Drosophila melanogaster Protein Interaction Network." PNAS USA 102 (9), 3192 (2005).
[2] Technically, it's using these subgraphs as generic features and then crunching the feature vectors from examples of each mechanism through a generalized decision tree in order to learn how to discriminate among them. Boosting is used within this process in order to reduce the error rates. The advantage of this approach to model selection and validation, as Chris pointed out, is that it doesn't assume a priori which features (e.g., degree distribution, clustering coefficient, distance distribution, whatever) are interesting, but rather chooses the ones that can actually discriminate between things we believe are different.
[3] Chris called it "biological validation," but the same thing happens in sociology and Internet modeling, too.
[4] I admit that I'm a little skeptical of degree-based models of these networks, since they seem to assume that we're getting the degree distribution roughly right. That assumption is only reasonable if our sampling of the interactions attached to a particular vertex is unbiased, which I'm not sure about.
[5] After some digging, I couldn't find the reference for this work. I did find this one, however, which illustrates a different technique for a related problem. I. Iossifov et al., "Probabilistic inference of molecular networks from noisy data sources." 20 (8), 1205 (2004).
[6] C. M. Li and R. R. Klevecz, "A rapid genome-scale response of the transcriptional oscillator to perturbation reveals a period-doubling path to phenotypic change." PNAS USA 103 (44), 16254 (2006).
[7] Maribeth Oscamou pointed out to me during the talk that any attempt to construct such rules have to account for processes like the biochemical degradation of the signals. That is, unlike electric circuits, there's no strict conservation of the "charge" carrier.
posted January 25, 2007 01:20 PM in Scientifically Speaking | permalink | Comments (0)
January 24, 2007
DIMACS - Complex networks and their applications (Day 2)
There were several interesting talks today, or rather, I should say that there were several talks today that made me think about things beyond just what the presenters said. Here's a brief recap of the ones that made me think the most, and some commentary about what I thought about. There were other good talks today, too. For instance, I particularly enjoyed Frank McSherry's talk on doing PageRank on his laptop. There was also one talk on power laws and scale-free graphs that stimulated a lot of audience, ah, interaction - it seems that there's a lot of confusion both over what a scale-free graph is (admittedly the term has no consistent definition in the literature, although there have been some recent attempts to clarify it in a principled manner), and how to best show that some data exhibit power-law behavior. Tomorrow's talks will be more about networks in various biological contexts.
Complex Structures in Complex Networks
Mark Newman's (U. Michigan) plenary talk mainly focused on the importance of having good techniques to extract information from networks, and being able to do so without making a lot of assumptions about what the technique is supposed to look for. That is, rather than assume that some particular kind of structure exists and then look for it in our data, why not let the data tell you what kind of interesting structure it has to offer? [1] The tricky thing about this approach to network analysis, though, is working out a method that is flexible enough to find many different kinds of structure, and to present only that which is unusually strong. (Point to ponder: what should we mean by "unusually strong"?) This point was a common theme in a couple of the talks today. The first example that Mark gave of a technique that has this nice property was a beautiful application of spectral graph theory to the task of find a partition of the vertices that give an extremal value of modularity. If we ask for the maximum modularity, this heuristic method [2], using the positive eigenvalues of the resulting solution, gives us a partition with very high modularity. But, using the negative eigenvalues gives a partition that minimizes the modularity. I think we normally think of modules meaning assortative structures, i.e., sparsely connected dense subgraphs. But, some networks exhibit modules that are approximately bipartite, i.e., they are disassortative, being densely connected sparse subgraphs. Mark's method naturally allows you to look for either. The second method he presented was a powerful probabilistic model of node clustering that can be appropriately parameterized (fitted to data) via expectation-maximization (EM). This method can be used to accomplish much the same results as the previous spectral method, except that it can look for both assortative and disassortative structure simultaneously in the same network.
Hierarchical Structure and the Prediction of Missing Links
In an afternoon talk, Cris Moore (U. New Mexico) presented a new and powerful model of network structure, the hierarchical random graph (HRG) [5]. (Disclaimer: this is joint work with myself and Mark Newman.) A lot of people in the complex networks literature have talked about hierarchy, and, presumably, when they do so, they mean something roughly along the lines of the HRG that Cris presented. That is, they mean that nodes with a common ancestor low in the hierarchical structure are more likely to be connected to each other, and that different cuts across it should produce partitions that look like communities. The HRG model Cris presented makes these notions explicit, but also naturally captures the kind of assortative hierarchical structure and the disassortative structure that Mark's methods find. (Test to do: use HRG to generate mixture of assortative and disassortative structure, then use Mark's second method to find it.) There are several other attractive qualities of the HRG, too. For instance, using a Monte Carlo Markov chain, you can find the hierarchical decomposition of a single real-world network, and then use the HRG to generate a whole ensemble of networks that are statistically similar to the original graph [6]. And, because the MCMC samples the entire posterior distribution of models-given-the-data, you can look not only at models that give the best fit to the data, but you can look at the large number of models that give an almost-best fit. Averaging properties over this ensemble can give you more robust estimates of unusual topological patterns, and Cris showed how it can also be used to predict missing edges. That is, suppose I hide some edges and then ask the model to predict which ones I hid. If it can do well at this task, then we've shown that the model is capturing real correlations in the topology of the real graph - it has the kind of explanatory power that comes from making correct predictions. These kinds of predictions could be extremely useful for laboratory or field scientists who manually collect network data (e.g., protein interaction networks or food webs) [7]. Okay, enough about my own work!
The Optimization Origins of Preferential Attachment
Although I've seen Raissa D'Souza (UC Davis) talk about competition-induced preferential attachment [8] before, it's such an elegant generalization of PA that I enjoyed it a second time today. Raissa began by pointing out that most power laws in the real-world can't extend to infinity - in most systems, there are finite limits to the size that things can be (the energy released in an earthquake or the number of edges a vertex can have), and these finite effects will typically manifest themselves as exponential cutoffs in the far upper tail of the distribution, which takes the probability of these super-large events to zero. She used this discussion as a springboard to introduce a relatively simple model of resource constraints and competition among vertices in a growing network that produces a power-law degree distribution with such an exponential cutoff. The thing I like most about this model is that it provides a way for (tempered) PA to emerge from microscopic and inherently local interactions (normally, to get pure PA to work, you need global information about the system). The next step, of course, is to find some way to measure evidence for this mechanism in real-world networks [9]. I also wonder how brittle the power-law result is, i.e., if you tweak the dynamics a little, does the power-law behavior disappear?
Web Search and Online Communities
Andrew Tomkins (of Yahoo! Reserch) is a data guy, and his plenary talk drove home the point that Web 2.0 applications (i.e., things that revolve around user-generated content) are creating a huge amount of data, and offering unparalleled challenges for combining, analyzing, and visualizing this data in meaningful ways. He used Flickr (a recent Y! acquisition) as a compelling example by showing an interactive (with fast-rewind and fast-forward features) visual stream of the trends in user-generated tags for user-posted images, annotated with notable examples of those images. He talked a little about the trickiness of the algorithms necessary to make such an application, but what struck me most was his plea for help and ideas in how to combine information drawn from social networks with user behavior with blog content, etc. to make more meaningful and more useful applications - there's all this data, and they only have a few ideas about how to combine it. The more I learn about Y! Research, the more impressed I am with both the quality of their scientists (they recently hired Duncan Watts), and the quality of their data. Web 2.0 stuff like this gives me the late-1990s shivers all over again. (Tomkins mentioned that in Korea, unlike in the US, PageRank-based search has been overtaken by an engine called Naver, which is driven by users building good sets of responses to common search queries.)
-----
[1] To be more concrete, and perhaps in lieu of having a better way of approaching the problem, much of the past work on network analysis has taken the following approach. First, think of some structure that you think might be interesting (e.g., the density of triangles or the division into sparsely connected dense subgraphs), design a measure that captures that structure, and then measure it in your data (it turns out to be non-trivial to do this in an algorithm independent way). Of course, the big problem with this approach is that you'll never know whether there is other structure that's just as important as, or maybe more important than, the kind you looked for, and that you just weren't clever enough to think to look for it.
[2] Heuristic because Mark's method is a polynomial time algorithm, while the problem of modularity maximization was recently (finally...) shown to be NP-complete. The proof is simple, and, in retrospect, obvious - just as most such proofs inevitably end up being. See U. Brandes et al. "Maximizing Modularity is hard." Preprint (2006).
[3] M. E. J. Newman, "Finding community structure in networks using the eigenvectors of matrices." PRE 74, 036104 (2006).
[4] M. E. J. Newman and E. A. Leicht, "Mixture models and exploratory data analysis in networks." Submitted to PNAS USA (2006).
[5] A. Clauset, C. Moore and M. E. J. Newman, "Structural Inference of Hierarchies in Networks." In Proc. of the 23rd ICML, Workshop on "Statistical Network Analysis", Springer LNCS (Pittsburgh, June 2006).
[6] This capability seems genuinely novel. Given that there are an astronomical number of ways to rearrange the edges on a graph, it's kind of amazing that the hierarchical decomposition gives you a way to do such a rearrangement, but one which preserves the statistical regularities in the original graph. We've demonstrated this for the degree distribution, the clustering coefficient, and the distribution of pair-wise distances. Because of the details of the model, it sometimes gets the clustering coefficient a little wrong, but I wonder just how powerful / how general this capability is.
[7] More generally though, I think the idea of testing a network model by asking how well it can predict things about real-world problems is an important step forward for the field; previously, "validation" consisted of showing only a qualitative (or worse, a subjective) agreement between some statistical measure of the model's behavior (e.g., degree distribution is right-skewed) and the same statistical measure on a real-world network. By being more quantitative - by being more stringent - we can say stronger things about the correctness of our mechanisms and models.
[8] R. M. D'Souza, C. Borgs, J. T. Chayes, N. Berger, and R. Kleinberg, "Emergence of Tempered Preferential Attachment From Optimization", To appear in PNAS USA, (2007).
[9] I think the best candidate here would be the BGP graph, since there is clearly competition there, although I suspect that the BGP graph structure is a lot more rich than the simple power-law-centric analysis has suggested. This is primarily due to the fact that almost all previous analyses have ignored the fact that the BGP graph exists as an expression of the interaction of business interests with the affordances of the Border Gateway Protocol itself. So, its topological structure is meaningless without accounting for the way it's used, and this means accounting for complexities of the customer-provider and peer-to-peer relationships on the edges (to say nothing of the sampling issues involved in getting an accurate BGP map).
posted January 24, 2007 02:18 AM in Scientifically Speaking | permalink | Comments (1)
January 23, 2007
DIMACS - Complex networks and their applications (Day 1)
Today and tomorrow, I'm at the DIMACS workshop on complex networks and their applications, held at Georgia Tech's College of Computing. Over the course of the workshop, I'll be blogging about the talks I see and whatever ideas they stimulate (sadly, I missed most of the first day because of travel).
The most interesting talk I saw Monday afternoon was by Ravi Kumar (Yahoo! Research), who took location data of users on LiveJournal, and asked Do we see the same kind of routable structure - i.e., an inverses-square law relationship in the distance between people and the likelihood that they have a LJ connection - that Kleinberg showed was optimal for distributed / local search? Surprisingly, they were able to show that in the US, once you correct for the fact that there can be many people at a single "location" in geographic space (approximated to the city level), you do indeed observe exactly the kind of power-law that Kleinberg predicted [1]. Truly, this was a kind of stunning confirmation of Kleinberg's theory. So now, the logical question would be, What mechanism might produce this kind of structure in geographic space? Although you could probably get away with assuming a priori the population distribution, what linking dynamics would construct the observed topological pattern? My first project in graduate school asked exactly this question for the pure Kleinberg model, and I wonder if it could be adapted to the geographic version that Kumar et al. consider.
[1] D. Liben-Nowell, et al. "Geographic Routing in Social Networks." PNAS USA 102, 33 11623-1162 (2005).
posted January 23, 2007 06:24 AM in Scientifically Speaking | permalink | Comments (0)
January 02, 2007
One brain, two brains, Red brain, blue brains.
Brains, brains, brains.
How do they do that thing that they do?
One of my first posts here, almost two years ago, was a musing on the structure and function of brains, and how, although bird brains and primate brains are structured quite differently, they seem to perform many of the same "high cognitive" tasks that we associate with intelligence. Carrion crows that use tools, and magpies with a sense of permanence (my niece just recently learned this fact, and is infinitely amused by it). From my musing in early 2005:
So how is it that birds, without a neocortex, can be so intelligent? Apparently, they have evolved an set of neurological clusters that are functionally equivalent to the mammal's neocortex, and this allows them to learn and predict complex phenomena. The equivalence is an important point in support of the belief that intelligence is independent of the substrate on which it is based; here, we mean specifically the types of supporting structures, but this independence is a founding principle of the dream of artificial intelligence (which is itself a bit of a misnomer). If there is more than one way that brains can create intelligent behavior, it is reasonable to wonder if there is more than one kind of substance from which to build those intelligent structures, e.g., transitors and other silicon parts.
Parrots, those inimitable imitators, are linguistic acrobats, but are they actually intelligent? There is, apparently, evidence that they are. Starting in 1977, Irene Pepperberg (Dept. Psychology, Brandeis University) began training an African Grey parrot named Alex in the English language [1]. Amazingly, Alex has apparently mastered a vocabulary of about a hundred words, understands concepts like color and size, can convey his desires, and can count. (Pepperton has a short promotional video (3MB) that demonstrates some of these abilities, although her work has been criticized as nothing but glorified operant conditioning by Noam Chomsky. Of course, one could probably also argue that what humans do is actually nothing more than the same.)
How long will it be, I wonder, before they stick Alex in an MRI machine to see what his brain is doing? Can we tell a difference, neurologically, between operant conditioning and true understanding? Can an inter-species comparative neuroscience resolve questions about how the brain does what it does? For instance, do Alex's cortical clusters specialize in tasks in the same way that regions of the mammalian brain are specialized? I wonder, too, what the genetics of such a comparative neuroscience would say - are there genes and genetic regularoty structures that are conserved between both (intelligent) bird and (intelligent) mammal species? Many, many interesting questions here...
[1] Sadly, I must admit, what brought Alex to my attention, was not his amazingly human-like linguistic abilities. Rather, it was an article in the BBC about another African Grey named N'kisi, who has been used to try to demonstrate telepathy in animals. N'kisi, trained by an artist Aimée Morgana, has a larger vocabulary than Alex, and also seems to have a (wry) sense of humor.
In the BBC article, there's a cryptic reference to an experiment that apparently demonstrates N'kisi's talent with language. But, a little digging reveals that this experiment was actually intended to show that N'kisi has a telepathic connection with Morgana. And this is what got the BBC to do an article about the intelligence of parrots, even though the article makes no overt mention of the pseudo-scientific nature of the experiment.
posted January 2, 2007 09:48 AM in Obsession with birds | permalink | Comments (0)
October 31, 2006
Future of computing
The New York Times has a short piece covering a recent symposium run by the National Academies on the future of computing. And, naturally enough, the intertwining of social networks and technological networks (e.g., YouTube and MySpace) was a prominent topic. Jon Kleinberg represented our community at the symposium. From the article:
But with the rise of the Internet, social networks and technology networks are becoming inextricably linked, so that behavior in social networks can be tracked on a scale never before possible.
“We’re really witnessing a revolution in measurement,” Dr. Kleinberg said.
The new social-and-technology networks that can be studied include e-mail patterns, buying recommendations on commercial Web sites like Amazon, messages and postings on community sites like MySpace and Facebook, and the diffusion of news, opinions, fads, urban myths, products and services over the Internet. Why do some online communities thrive, while others decline and perish? What forces or characteristics determine success? Can they be captured in a computing algorithm?
Isn't it nice to see your research topics in print in a major news paper? To me, the two exciting things about this area are the sheer number of interesting questions to study, and the potential for their answers to qualitatively improve our lives. (Although, being more of a theoretician than an engineer, I suppose I leave the latter for other people.) For the Web in particular, new ideas like YouTube and del.icio.us have made it possible to study social behavior in ways never before possible. Physicists and computer scientists deserve some credit for recognizing that these sources of data offer a fundamentally new perspective on questions that sociologists have been kicking around for several decades now.
As is true perhaps with any relatively new field, there still a lot of debate and jostling about how to do good science here. But mostly, that just adds to the excitement. There's a lot of opportunity to say important and interesting things about these systems, and, to develop new and useful applications on top of them.
Update Nov 2: In a separate piece, the NYTimes discusses Tim Berners-Lee's efforts to found a "Web science" field that combines aspects of Computer Science with Sociology and Business. Sounds familiar, no? Here's the final thought from the article:
Ben Shneiderman, a professor at the University of Maryland, said Web science was a promising idea. “Computer science is at a turning point, and it has to go beyond algorithms and understand the social dynamics of issues like trust, responsibility, empathy and privacy in this vast networked space,” Professor Shneiderman said. “The technologists and companies that understand those issues will be far more likely to succeed in expanding their markets and enlarging their audiences.”
(Tip to C. Moore)
posted October 31, 2006 05:07 PM in Computer Science | permalink | Comments (0)
October 11, 2006
Hierarchy in networks
After several months of silence on it, I've finally posted a new paper (actually written more than 5 months ago!) on the arxiv about the hierarchical decomposition of network structure. I presented it at the 23rd International Conference on Machine Learning (ICML) Workshop on Social Network Analysis in June.
Aaron Clauset, Cristopher Moore, M. E. J. Newman, "Structural Inference of Hierarchies in Networks", to appear in Lecture Notes in Computer Science (Springer-Verlag). physics/0610051
One property of networks that has received comparatively little attention is hierarchy, i.e., the property of having vertices that cluster together in groups, which then join to form groups of groups, and so forth, up through all levels of organization in the network. Here, we give a precise definition of hierarchical structure, give a generic model for generating arbitrary hierarchical structure in a random graph, and describe a statistically principled way to learn the set of hierarchical features that most plausibly explain a particular real-world network. By applying this approach to two example networks, we demonstrate its advantages for the interpretation of network data, the annotation of graphs with edge, vertex and community properties, and the generation of generic null models for further hypothesis testing.
posted October 11, 2006 07:44 PM in Self Referential | permalink | Comments (0)
October 01, 2006
Visualizing biology
Aftering seeing it blogged on Cosmic Variance and Shtetl-Optimzed, I wasn't surprised when my friend Josh Adelman wrote to share it, too, with "it" being this wonderful bit of bio-visualization:
A brief popular explanation of the video, which gives substantial background on Harvard's sponsorship of XVIVO, the company that did the work, is here. The video above is apparently a short version of a longer one that will be used in Harvard's undergraduate education; the short version will play at this year's Siggraph 2006 Electronic Theater.
Anyway, what prompted me to blog about this video is that, being a biophysicist, Josh added some valuable additional insight into what's being shown here, and the new(-ish) trend of trying to popularize this stuff through these beautiful animations.
For instance, that determined looking "walker" in the video is actually a kinesin molecule walking along a microtubule, which was originally worked out by the Vale Lab at UCSF, which have their own (more technical) animation of how the walker actually works. Truly, an amazingly little protein.
Another of the more visually stunning bits of the film is the self-assembling behavior of the microtubules themselves. This work was done by the Nogales Lab at Berkeley, and they too have some cool animations that explain how microtubules dynamically assemble and disassemble.
DNA replication hardly makes an appearance in the video above, but the Walter & Eliza Hall Institute produced several visually stunning shorts that show how this process works (the sound-effects are cheesy, but it's clear the budget was well spent on other things).
posted October 1, 2006 03:43 PM in Scientifically Speaking | permalink | Comments (0)
August 22, 2006
Jon Kleinberg wins the Nevanlinna Prize
Jon Kleinberg, a computer science professor at Cornell, has done it again, this time winning the Nevanlinna Prize for major contributions to the mathematical aspects of computer science (given out every four years; created in 1982; Robert Tarjan was the first recipient). What's most gratifying about his work is that he manages to ask (and answer!) questions that directly effect the way our complex world works. For instance, his work on the small world phenonema (and its more practical side: decentralized search) was the inspiration of my first graduate school research project.
Congratulations Jon!
(tip to Dragomir Radev via SOCNET)
posted August 22, 2006 10:47 AM in Scientifically Speaking | permalink | Comments (2)
July 31, 2006
Criticizing global warming
Dr. Peter Doran, an antarctic climate resesarcher at UIC, was the author of one of two studies that the polemicists like to use to dispute global warming. Although he's tried to correct the out-of-control spinning on the topic that certain deniers are wont to do, he's been largely unsuccessful. Politics and news, as always, trump both accuracy and honesty. In a recent article for the Amherst Times (apparently pulled mostly from his review of An Inconvenient Truth, which he gives "two frozen thumbs up"), he discusses this problem, and the facts. From the original:
...back to our Antarctic climate story, we indeed stated that a majority -- 58 percent -- of the continent cooled between 1966 and 2000, but let’s not forget the remainder was warming. One region, the Antarctic Peninsula, warmed at orders of magnitude more than the global average. Our paper did not predict the future and did not make any comment on climate anywhere else on Earth except to say, in our very first sentence, that the Earth’s average air temperature increased by 0.06 degrees Celsius per decade in the 20th century.
New models created since our paper was published have suggested a link between the lack of significant warming in Antarctica to the human-induced ozone hole over the continent. Besides providing a protective layer over the Earth, ozone is a greenhouse gas. The models now suggest that as the ozone hole heals, thanks to world-wide bans on harmful CFCs, aerosols, and other airborne particles, Antarctica should begin to fall in line and warm up with the rest of the planet. These models are conspicuously missing from climate skeptic literature. Also missing is the fact that there has been some debate in the science community over our results. We continue to stand by the results for the period analyzed, but an unbiased coverage would acknowledge the differences of opinion.
Tip to onegoodmove.
posted July 31, 2006 04:18 PM in Global Warming | permalink | Comments (0)
July 26, 2006
Models, errors and the methods of science.
A recent posting on the arxiv prompts me to write down some recent musings about the differences between science and non-science.
On the Nature of Science by B.K. Jennings
A 21st century view of the nature of science is presented. It attempts to show how a consistent description of science and scientific progress can be given. Science advances through a sequence of models with progressively greater predictive power. The philosophical and metaphysical implications of the models change in unpredictable ways as the predictive power increases. The view of science arrived at is one based on instrumentalism. Philosophical realism can only be recovered by a subtle use of Occam's razor. Error control is seen to be essential to scientific progress. The nature of the difference between science and religion is explored.
Which can be summarized even more succinctly by George Box, famously saying "all models are wrong but some models are useful" with the addendum that this recognition is what makes science different from religion (or other non-scientific endeavors), and that the sorting out the useful from the useless is what drives science forward.
In addition to being a relatively succinct introduction to the basic terrain of modern philosophy of science, Jennings also describes two common critiques of science. The first is the God of the Gaps idea: basically, science explains how nature works and everything left unexplained is the domain of God. Obviously, the problem is that those gaps have a pesky tendency to disappear over time, taking that bit of God with them. For Jennings, this idea is just a special case of the more general "Proof by Lack of Imagination" critique, which is summarized as "I cannot imagine how this can happen naturally, therefore it does not, or God must have done it." As with the God of the Gaps idea, more imaginative people tend to come along (or have come along before) who can imagine how it could happen naturally (e.g., continental drift). Among physicists who like this idea, things like the precise value of fundamental constants are grist for the mill, but can we really presume that we'll never be able to explain them naturally?
Evolution is, as usual, one of the best examples of this kind of attack. For instance, almost all of the arguments currently put forth by creationists are just a rehashing of arguments made in the mid-to-late 1800s by religious scientists and officials. Indeed, Darwin's biggest critic was the politically powerful naturalist Sir Richard Owen, who objected to evolution because he preferred the idea that God used archetypical forms to derive species. The proof, of course, was in the overwhelming weight of evidence in favor of evolution, and, in the end, with Darwin being much more clever than Owen.
Being the bread and butter of science, this may seem quite droll. But I think non-scientists have a strong degree of cognitive dissonance when faced with such evidential claims. That is, what distinguishes scientists from non is our conviction that knowledge about the nature of the world is purely evidential, produced only by careful observations, models and the control of our errors. For the non-scientist, this works well enough for the knowledge required to see to the basics of life (eating, moving, etc.), but conflicts with (and often loses out to) the knowledge given to us by social authorities. In the West before Galileo, the authorities were the Church or Aristotle - today, Aristotle has been replaced by talk radio, television and cranks pretending to be scientists. I suspect that it's this conflicting relationship with knowledge that might explain several problems with the lay public's relationship with science. Let me connect this with my current reading material, to make the point more clear.
Deborah Mayo's excellent (and I fear vastly under-read) Error and the Growth of Experimental Knowledge, is a dense and extremely thorough exposition of a modern philosophy of science, based on the evidential model I described above. As she reinterprets Kuhn's analysis of Popper, she implicitly points to an explanation for why science so often classes with non-science, and why these clashes often leave scientists shaking their heads in confusion. Quoting Kuhn discussing why astrology is not a science, she says
The practitioners of astrology, Kuhn notes, "like practitioners of philosophy and some social sciences [AC: I argue also many humanities]... belonged to a variety of different schools ... [between which] the debates ordinarily revolved about the implausibility of the particular theory employed by one or another school. Failures of individual predictions played very little role." Practitioners were happy to criticize the basic commitments of competing astrological schools, Kuhn tells us; rival schools were constantly having their basic presuppositions challenged. What they lacked was that very special kind of criticism that allows genuine learning - the kind where a failed prediction can be pinned on a specific hypothesis. Their criticism was not constructive: a failure did not genuinely indicate a specific improvement, adjustment or falsification.
That is, criticism that does not focus on the evidential basis of theories is what non-sciences engage in. In Kuhn's language, this is called "critical discourse" and is what distinguishes non-science from science. In a sense, critical discourse is a form of logical jousting, in which you can only disparage the assumptions of your opponent (thus undercutting their entire theory) while championing your own. Marshaling anecdotal evidence in support of your assumptions is to pseudo-science, I think, what stereotyping is to racism.
Since critical discourse is the norm outside of science, is it any wonder that when non-scientists, attempting to resolve the cognitive dissonance between authoritative knowledge and evidential knowledge, resort to the only form of criticism they understand? This leads me to be extremely depressed about the current state of science education in this country, and about the possibility of politicians ever learning from their mistakes.
posted July 26, 2006 11:26 PM in Scientifically Speaking | permalink | Comments (1)
July 17, 2006
Uncertainty about probability
In the past few days, I've been reading about different interpretations of probability, i.e., the frequentist and bayesian approaches (for a primer, try here). This has, of course, led me back to my roots in physics since both quantum physics (QM) and statistical mechanics both rely on probabilities to describe the behavior of nature. Amusingly, I must not have been paying much attention while I was taking QM at Haverford, e.g., Neils Bohr once said "If quantum mechanics hasn't profoundly shocked you, you haven't understood it yet." and back then I was neither shocked nor confused by things like the uncertainty principle, quantum indeterminacy or Bell's Theorem. Today, however, it's a different story entirely.
John Baez has a nice summary and selection of news-group posts that discuss the idea of frequentism versus bayesianism in the context of theoretical physics. This, in turn, led me to another physicist's perspective on the matter. The late Ed Jaynes has an entire book on probability from a physics perspective, but I most enjoyed his discussion of the physics of a "random experiment", in which he notes that quantum physics differs sharply in its use of probabilities from macroscopic sciences like biology. I'll just quote Jaynes on this point, since he describes it so eloquently:
In biology or medicine, if we note that an effect E (for example, muscle contraction) does not occur unless a condition C (nerve impulse) is present, it seems natural to infer that C is a necessary causative agent of E... But suppose that condition C does not always lead to effect E; what further inferences should a scientist draw? At this point the reasoning formats of biology and quantum theory diverge sharply.
... Consider, for example, the photoelectric effect (we shine a light on a metal surface and find that electrons are ejected from it). The experimental fact is that the electrons do not appear unless light is present. So light must be a causative factor. But light does not always produce ejected electrons... Why then do we not draw the obvious inference, that in addition to the light there must be a second causative factor...?
... What is done in quantum theory is just the opposite; when no cause is apparent, one simple postulates that no cause exists; ergo, the laws of physics are indeterministic and can be expressed only in probability form.
... In classical statistical mechanics, probability distributions represent our ignorance of the true microscopic coordinates - ignorance that was avoidable in principle but unavoidable in practice, but which did not prevent us from predicting reproducible phenomena, just because those phenomena are independent of the microscopic details.
In current quantum theory, probabilities express the ignorance due to our failure to search for the real causes of physical phenomena. This may be unavoidable in practice, but in our present state of knowledge we do not know whether it is unavoidable in principle.
Jaynes goes on to describe how current quantum physics may simply be in a rough patch where our experimental methods are simply too inadequate to appropriately isolate the physical causes of the apparent indeterministic behavior of our physical systems. But, I don't quite understand how this idea could square with the refutations of such a hidden variable theory after Bell's Theorem basically laid local realism to rest. It seems to me that Jaynes and Baez, in fact, evoke similar interpretations of all probabilities, i.e., that they only represent our (human) model of our (human) ignorance, which can be about either the initial conditions of the system in question, the causative rules that cause it to evolve in certain ways, or both.
It would be unfair to those statistical physicists who work in the field of complex networks to say that they share the same assumptions of no-causal-factor that their quantum physics colleagues may accept. In statistical physics, as Jaynes points out, the reliance on statistical methodology is forced on statistical physicists by our measurement limitations. Similarly, in complex networks, it's impractical to know the entire developmental history of the Internet, the evolutionary history of every species in a foodweb, etc. But unlike statistical physics, in which experiments are highly repeatable, every complex network has a high degree of uniqueness, and are thus more like biological and climatological systems where there is only one instance to study. To make matters even worse, complex networks are also quite small, typically having between 10^2 and 10^6 parts; in contrast, most systems that concern statistical physics have 10^22 or more parts. In these, it's probably not terribly wrong to use a frequentist perspective and assume that their relative frequencies behave like probabilities. But when you only have a few thousand or million parts, such claims seems less tenable since it's hard to argue that you're close to asymptotic behavior in this case. Bayesianism, being more capable of dealing with data-poor situations in which many alternative hypotheses are plausible, seems to offer the right way to deal with such problems. But, perhaps owing to the history of the field, few people in network science seem to use it.
For my own part, I find myself being slowly seduced by their siren call of mathematical rigor and the notion of principled approaches to these complicated problems. Yet, there are three things about the bayesian approach that make me a little uncomfortable. First, given that with enough data, it doesn't matter what your original assumption about the likelihood of any outcome is (i.e., your "prior"), shouldn't bayesian and frequentist arguments lead to the same inferences in a limiting, or simply very large, set of identical experiments? If this is right, then it seems more reasonable that statistical physicists have been using frequentist approaches for years with great success. Second, in the case where we are far from the limiting set of experiments, doesn't being able to choose an arbitrary prior amount to a kind of scientific relativism? Perhaps this is wrong because the manner in which you update your prior, given new evidence, is what distinguishes it from certain crackpot theories.
Finally, choosing an initial prior seems highly arbitrary, since one can always recurse a level and ask what prior on priors you might take. Here, I like the ideas of a uniform prior, i.e., I think everything is equally plausible, and of using the principle of maximum entropy (MaxEnt; also called the principle of indifference, by Laplace). Entropy is a nice way to connect this approach with certain biases in physics, and may say something very deep about the behavior of our incomplete description of nature at the quantum level. But, it's not entirely clear to me (or, apparently, others: see here and here) how to use maximum entropy in the context of previous knowledge constraining our estimates of the future. Indeed, one of the main things I still don't understand is how, if we model the absorption of knowledge as a sequential process, to update our understanding of the world in a rigorous way while guaranteeing that the order we see the data doesn't matter.
Update July 17: Cosma points out that Jaynes's Bayesian formulation of statistical mechanics leads to unphysical implications like a backwards arrow of time. Although it's comforting to know that statistical mechanics cannot be reduced to mere Bayesian crank-turning, it doesn't resolve my confusion about just what it means that the quantum state of matter is best expressed probabilistically! His article also reminds me that there are good empirical reasons to use a frequentist approach, reasons based on Mayo's arguments and which should be familiar to any scientist who has actually worked with data in the lab. Interested readers should refer to Cosma's review of Mayo's Error, in which he summarizes her critique of Bayesianism.
posted July 17, 2006 03:30 PM in Scientifically Speaking | permalink | Comments (0)
July 06, 2006
An ontological question about complex systems
Although I've been reading Nature News for several years now (as part of my daily trawl for treasure in the murky waters of science), I first came to recognize one of their regular writers Philip Ball when he wrote about my work on terrorism with Maxwell Young. His essay, now hidden behind Nature's silly subscription-only barrier, sounded an appropriately cautionary note about using statistical patterns of human behavior to predict the future, and was even titled "Don't panic, it might never happen."
The idea that there might be statistical laws that govern human behavior can be traced, as Ball does in his essay, back to the English philosopher Thomas Hobbes (1588-1679) in The Leviathan and to the French positivist philosopher Auguste Comte (1798-1857; known as the father of sociology, and who also apparently coined the term "altruism"), who were inspired by the work of physicists in mechanizing the behavior of nature to try to do the same with human societies.
It seems, however, that somewhere between then and now, much of sociology has lost interest in such laws. A good friend of mine in graduate school for sociology (who shall remain nameless to protect her from the politics of academia) says that her field is obsessed with the idea that context, or nurture, drives all significant human behavior, and that it rejects the idea that overarching patterns or laws of society might exist. These, apparently, are the domain of biology, and thus Not Sociology. I'm kind of stunned that any field that takes itself seriously would so thoroughly cling to the nearly medieval notion of the tabula rasa (1) in the face of unrelenting scientific evidence to the contrary. But, if this territory has been abandoned by sociologists (2), it has recently, and enthusiastically, been claimed by physicists (who may or may not recognize the similarity of their work to a certain idea in science fiction).
Ball's background is originally in chemistry and statistical physics, and having spent many years as an editor at Nature, he apparently now has a broad perspective on modern science. But, what makes his writing so enjoyable is the way he places scientific advances in their proper historical context, showing both where the inspiration may have come from, and how other scientists were developing similar or alternative ideas concurrently. These strengths are certainly evident in his article about the statistical regularity of terrorism, but he puts them to greater use in several books and, in particular, one on physicists' efforts to create something he calls sociophysics. As it turns out, however, this connection between physics and sociology is not a new one, and the original inspiration for statistical physics (one of the three revolutionary ideas in modern physics; the other two are quantum mechanics and relativity) is owed to social scientists.
In the mid 1800s, James Clerk Maxwell, one of the fathers of statistical physics, read Henry Thomas Buckle's lengthy History of Civilization. Buckle was a historian by trade, and a champion of the idea that society's machinations are bound by fundamental laws. Maxwell, struggling with the question of how to describe the various motions of particles in a gas, was struck by Buckle's descriptions of the statistical nature of studies of society. Such studies sought not to describe each individual and their choices exactly, but instead represent the patterns of behavior statistically, and often pointed to surprising regularities, e.g., the near-stable birth or suicide rates in a particular region. As a result, Maxwell abandoned the popular approach of describing gas particles only using Newtonian mechanics, i.e., an attempt to describe every particle's position and motion exactly, in favor for a statistical approach that focused on the distribution of velocities.
It was the profound success of these statistical descriptions that helped cement this approach as one of the most valuable tools available to physicists, and brought about some pretty profound shifts in our understanding of gasses, materials and even astrophysics. So, it seems fitting that statistical physicists are now returning to their roots by considering statistical laws of human behavior. Alas, I doubt that most such physicists appreciate this fact.
These efforts, which Ball surveys in "Critical Mass" (Farrar, Straus and Giroux, 2004) via a series of well-written case studies, have dramatically altered our understanding of phenomena as varied as traffic patterns (which have liquid, gaseous, solid and meta-stable states along with the corresponding phase transitions), voting patterns in parliamentary elections (which display nice heavy-tailed statistics), the evolution of pedestrian traffic trails across a university quad, economics and the statistics of businesses and markets, and a very shallow discussion of social networks. Although his exposition is certainly aimed at the layman, he does not shy away from technical language when appropriate. Pleasantly, he even reproduces figures from the original papers when it serves his explanations. Given that these phenomena were drawn from a burgeoning field of interdisciplinary research, it's easy to forgive him for omitting some of my favorite topics, treating others only shallowly, and mercifully leaving out the hobby horses of cellular automata, genetic algorithms and artificial life.
Now, after seeing that list of topics, you might think that "Critical Mass" was a book about complex systems, and you might be right. But, you might be wrong, too, which is the problem when there's no strict definition of a term. So, let's assume he has, and see what this offers in terms of clarifying the corresponding ontological question. For one thing, Ball's choices suggest that perhaps we do not need other ill-defined properties like emergence, self-organization or robustness (3) to define a complex system. Instead, perhaps when we say we are studying a "complex system," we simply mean that it has a highly heterogeneous composition that we seek to explain using statistical mechanisms. To me, the former means that I, because of my limited mental capacity to grasp complicated equations, relationships or a tremendously large configuration space, pretty much have to use a statistical characterization that omits most of the detailed structure of the system; also, I say heterogeneous because homogeneous systems are much easier to explain using traditional statistical mechanics. The latter means that I'm not merely interested in describing the system, which can certainly be done using traditional statistics, but rather in explaining the rules and laws that govern the formation, persistence and evolution of that structure. For me, this definition is attractive both for its operational and utilitarian aspects, but also because it doesn't require me to wave my hands, use obfuscating jargon or otherwise change the subject.
In general, it's the desire to establish laws that reflects complex systems' roots in physics, and it is this that distinguishes it from traditional statistics and machine learning. In those areas, the focus seems to me to be more on predictive power ("Huzzah! My error rate is lower than yours.") and less on mechanisms. My machine learning friends tell me that people are getting more interested in the "interpretability" of their models, but I'm not sure this is the same thing as building models that reflect the true mechanical nature of the underlying system... of course, one fundamental difference between much of statistical learning and what I've described above is that for many systems, there's no underlying mechanism! We shouldn't expect problems like keeping the spam out of my inbox to exhibit nice mechanistic behavior, and there are a tremendous number of such problems out there today. Fortunately, I'm happy to leave those to people who care more about error rates than mechanisms, and I hope they're happy to leave studying the (complex) natural world, mechanisms and all, to me.
Updates, July 7
(1) The notion of the tabula rasa is not antithetical to the idea that there are patterns in social behavior, but patterns per se are not the same as the kind of societal laws that the founders of sociology were apparently interested in, i.e., sociology apparently believes these patterns to be wholly the results of culture and not driven by things that every human shares like our evolutionary history as a species. I suppose there's a middle ground here, in which society has created the appearance of laws, which the sociophysicists then discover and mistake for absolutes. Actually, I'm sure that much of what physicists have done recently can be placed into this category.
(2) It may be the case that it is merely the portion of sociology that my friend is most familiar with that expresses this odd conviction, and that there are subfields that retain the idea that true mechanistic laws do operate in social systems. For all I know, social network analysis people may be of this sort; it would be nice to have an insider's perspective on this.
(3) Like the notions of criticality and universality, these terms actually do have precise, technical definitions in their proper contexts, but they've recently been co-opted in imprecisely ways and are now, unfortunately and in my opinion, basically meaningless in most of the complex systems literature.
posted July 6, 2006 07:09 PM in Reviews | permalink | Comments (0)
April 15, 2006
Ken Miller on Intelligent Design
Ken Miller, a cell biologist at Brown University, gave a (roughly hour long) talk at Case Western University in January on his involvement in the Dover PA trial on the teaching of Intelligent Design in American public schools. The talk is accessible, intelligent and interesting. Dr. Miller is an excellent speaker and if you can hang-in there until the Q&A at the end, he'll make an somewhat scary connection between the former dominance of the Islamic world (the Caliphate) in scientific progress and its subsequent trend toward non-rational theocratic thinking, and the position of the United States (and perhaps all of Western civilization) relative to its own future.
posted April 15, 2006 05:30 PM in Evolution | permalink | Comments (0)
March 01, 2006
The scenic view
In my formal training in physics and computer science, I never did get much exposure to statistics and probability theory, yet I have found myself consistently using them in my research (partially on account of the fact that I deal with real data quite often). What little formal exposure I did receive was always in some specific context and never focused on probability as a topic itself (e.g., statistical mechanics, which could hardly be called a good introduction to probability theory). Generally, my training played-out in the crisp and clean neighborhoods of logical reasoning, algebra and calculus, with the occasional day-trip to the ghetto of probability. David Mumford, a Professor of Mathematics at Brown University, opines about ongoing spread of that ghetto throughout the rest science and mathematics, i.e., how probability theory deserves a respect at least equal to that of abstract algebra, in a piece from 1999 on The Dawning of the Age of Stochasticity. From the abstract,
For over two millennia, Aristotle's logic has rules over the thinking of western intellectuals. All precise theories, all scientific models, even models of the process of thinking itself, have in principle conformed to the straight-jacket of logic. But from its shady beginnings devising gambling strategies and counting corpses in medieval London, probability theory and statistical inference now emerge as better foundations for scientific models ... [and] even the foundations of mathematics itself.
It may sound it, but I doubt that Mumford is actually overstating his case here, especially given the deep connection between probability theory, quantum mechanics (c.f. the recent counter-intuitive result on quantum interrogation) and complexity theory.
A neighborhood I'm more familiar with is that of special functions; things like the Gamma distribution, the Riemann Zeta function (a personal favorite), and the Airy functions. Sadly, these familiar friends show up very rarely in the neighborhood of traditional computer science, but instead hang out in the district of mathematical modeling. Robert Batterman, a Professor of Philosophy at Ohio State University, writes about why exactly these functions are so interesting in On the Specialness of Special Functions (The Nonrandom Effusions of the Divine Mathematician).
From the point of view presented here, the shared mathematical features that serve to unify the special functions - the universal form of their asymptotic expansions - depends upon certain features of the world.
(Emphasis his.) That is, the physical world itself, by presenting a patterned appearance, must be governed by a self-consistent set of rules that create that pattern. In mathematical modeling, these rules are best represented by asymptotic analysis and, you guessed it, special functions, that reveal the universal structure of reality in their asymptotic behavior. Certainly this approach to modeling has been hugely successful, and remains so in current research (including my own).
My current digs, however, are located in the small nexus that butts up against these neighborhoods and those in computer science. Scott Aaronson, who occupies an equivalent juncture between computer science and physics, has written several highly readable and extremely interesting pieces on the commonalities he sees in his respective locale. I've found them to be a particularly valuable way to see beyond the unfortunately shallow exploration of computational complexity that is given in most graduate-level introductory classes.
In NP-complete Problems and Physical Reality Aaronson looks out of his East-facing window toward physics for hints about ways to solve NP-complete problems by using physical processes (e.g., simulated annealing). That is, can physical reality efficiently solve instances of "hard" problems? Although he concludes that the evidence is not promising, he points to a fundamental connection between physics and computer science.
Then turning to look out his West-facing window towards computer science, he asks Is P Versus NP Formally Indepenent?, where he considers formal logic systems and the implications of Godel's Incompleteness Theorem for the likelihood of resolving the P versus NP question. It's stealing his thunder a little, but the most quotable line comes from his conclusion:
So I'll state, as one of the few definite conclusions of this survey, that P \not= NP is either true or false. It's one or the other. But we may not be able to prove which way it goes, and we may not be able to prove that we can't prove it.
There's a little nagging question that some researchers are only just beginning to explore, which is, are certain laws of physics formally independent? I'm not even entirely sure what that means, but it's an interesting kind of question to ponder on a lazy Sunday afternoon.
There's something else embedded in these topics, though. Almost all of the current work on complexity theory is logic-oriented, essentially because it was born of the logic and formal mathematics of the first half of the 20th century. But, if we believe Mumford's claim that statistical inference (and in particular Bayesian inference) will invade all of science, I wonder what insights it can give us about solving hard problems, and perhaps why they're hard to begin with.
I'm aware of only anecdotal evidence of such benefits, in the form of the Survey Propagation Algorithm and its success at solving hard k-SAT formulas. The insights from the physicists' non-rigorous results has even helped improve our rigorous understanding of why problems like random k-SAT undergo a phase transition from mostly easy to mostly hard. (The intuition is, in short, that as the density of constraints increases, the space of valid solutions fragments into many disconnected regions.) Perhaps there's more being done here than I know of, but it seems that a theory of inferential algorithms as they apply to complexity theory (I'm not even sure what that means, precisely; perhaps it doesn't differ significantly from PPT algorithms) might teach us something fundamental about computation.
posted March 1, 2006 02:32 PM in Interdisciplinarity | permalink | Comments (0)
February 21, 2006
Pirates off the Coast of Paradise
At the beginning of graduate school, few people have a clear idea of what area of research they ultimately want to get into. Many come in with vague or ill-informed notions of their likes and dislikes, most of which are due to the idiosyncrasies of their undergraduate major's curriculum, and perhaps scraps of advice from busy professors. For Computer Science, it seems that most undergraduate curricula emphasize the physical computer, i.e., the programming, the operating system and basic algorithm analysis, over the science, let alone the underlying theory that makes computing itself understandable. For instance, as a teaching assistant for an algorithms course during my first semester in grad school, I was disabused of any preconceptions when many students had trouble designing, carrying-out, and writing-up a simple numerical experiment to measure the running time of an algorithm as a function of its input size, and I distinctly remember seeing several minds explode (and, not in the Eureka! sense) during a sketch of Cantor's diagonalization argument. When you consider these anecdotes along with the flat or declining numbers of students enrolling in computer science, we have a grim picture of both the value that society attributes to Computer Science and the future of the discipline.
The naive inference here would be that students are (rightly) shying away from a field that serves little purpose to society, or to them, beyond providing programming talent for other fields (e.g., the various biological or medical sciences, or IT departments, which have a bottomless appetite for people who can manage information with a computer). And, with programming jobs being outsourced to India and China, one might wonder if the future holds anything but an increasing Dilbert-ization of Computer Science.
This brings us to a recent talk delivered by Prof. Bernard Chazelle (CS, Princeton) at the AAAS Annual Meeting about the relevance of the Theory of Computer Science (TCS for short). Chazelle's talk was covered briefly by PhysOrg, although his separate and longer essay really does a better job of making the point,
Moore's Law has fueled computer science's sizzle and sparkle, but it may have obscured its uncanny resemblance to pre-Einstein physics: healthy and plump and ripe for a revolution. Computing promises to be the most disruptive scientific paradigm since quantum mechanics. Unfortunately, it is the proverbial riddle wrapped in a mystery inside an enigma. The stakes are high, for our inability to “get” what computing is all about may well play iceberg to the Titanic of modern science.
He means that behind the glitz and glam of iPods, Internet porn, and unmanned autonomous vehicles armed with GPS-guided missles, TCS has been drawing fundamental connections, through the paradigm of abstract computation, between previously disparate areas throughout science. Suresh Venkatasubramanian (see also Jeff Erickson and Lance Fortnow) phrases it in the form of something like a Buddhist koan,
Theoretical computer science would exist even if there were no computers.
Scott Aaronson, in his inimitable style, puts it more directly and draws an important connection with physics,
The first lesson is that computational complexity theory is really, really, really not about computers. Computers play the same role in complexity that clocks, trains, and elevators play in relativity. They're a great way to illustrate the point, they were probably essential for discovering the point, but they're not the point. The best definition of complexity theory I can think of is that it's quantitative theology: the mathematical study of hypothetical superintelligent beings such as gods.
Actually, that last bit may be overstating things a little, but the idea is fair. Just as theoretical physics describes the physical limits of reality, theoretical computer science describes both the limits of what can be computed and how. But, what is physically possible is tightly related to what is computationally possible; physics is a certain kind of computation. For instance, a guiding principle of physics is that of energy minimization, which is a specific kind of search problem, and search problems are the hallmark of CS.
The Theory of Computer Science is, quite to the contrary of the impression with which I was left after my several TCS courses in graduate school, much more than proving that certain problems are "hard" (NP-complete) or "easy" (in P), or that we can sometimes get "close" to the best much more easily than we can find the best itself (approximation algorithms), or especially that working in TCS requires learning a host of seemingly unrelated tricks, hacks and gimmicks. Were it only these, TCS would be interesting in the same way that Sudoku puzzles are interesting - mildly diverting for some time, but eventually you get tired of doing the same thing over and over.
Fortunately, TCS is much more than these things. It is the thin filament that connects the mathematics of every natural science, touching at once game theory, information theory, learning theory, search and optimization, number theory, and many more. Results in TCS, and in complexity theory specifically, have deep and profound implications for what the future will look like. (E.g., do we live in a world where no secret can actually be kept hidden from a nosey third party?) A few TCS-related topics that John Baez, a mathematical physicist at UC Riverside who's become a promoter of TCS, pointed to recently include "cryptographic hash functions, pseudo-random number generators, and the amazing theorem of Razborov and Rudich which says roughly that if P is not equal to NP, then this fact is hard to prove." (If you know what P and NP mean, then this last one probably doesn't seem that surprising, but that means you're thinking about it in the wrong direction!) In fact, the question of P versus NP may even have something to say about the kind of self-consistency we can expect in the laws of physics, and whether we can ever hope to find a Grand Unified Theory. (For those of you hoping for worm-hole-based FTL travel in the future, P vs. NP now concerns you, too.)
Alas my enthusiasm for these implications and connections is stunted by a developing cynicism, not because of a failure to deliver on its founding promises (as, for instance, was the problem that ultimately toppled artificial intelligence), but rather because of its inability to convince not just the funding agencies like NSF that it matters, but its inability to convince the rest of Computer Science that it matters. That is, TCS is a vitally important, but a needlessly remote, field of CS, and is valued by the rest of CS for reasons analogous to those for which CS is valued by other disciplines: its ability to get things done, i.e., actual algorithms. This problem is aggravated by the fact that the mathematical training necessary to build toward a career in TCS is not a part of the standard CS curriculum (I mean at the undergraduate level, but the graduate one seems equally faulted). Instead, you acquire that knowledge by either working with the luminaries of the field (if you end up at the right school), or by essentially picking up the equivalent of a degree in higher mathematics (e.g., analysis, measure theory, abstract algebra, group theory, etc.). As Chazelle puts it in his pre-talk interview, "Computer science ... is messy and infuriatingly complex." I argue that this complexity is what makes CS, and particularly TCS, inaccessible and hard-to-appreciated. If Computer Science as a discipline wants to survive to see the "revolution" Chazelle forecasts, it needs to reevaluate how it trains its future members, what it means to have a science of computers, and even further, what it means to have a theory of computers (a point CS does abysmally on). No computer scientist likes to be told her particular area of study is glorified programming, but without significant internal and external restructuring, that is all Computer Science will be to the rest of the world.
posted February 21, 2006 12:06 AM in Scientifically Speaking | permalink | Comments (0)
February 13, 2006
Sampling science
Three things that recently caught my attention in my web-trawling:
The first is newly published work by a team of MIT neuroscientists who study rats and memory. They discovered that the neurons in rat brains have an instant-replay behavior that kicks-in during the idle periods between actions. Except, this is not the replay that many of us experience just after learning a new task, when we play the events over in our mind sequentially. No, rats think about things in reverse and at high-speed!
D. J. Foster and M. A. Wilson Nature, advance online publication 10.1038/04587 (2006)
The second is a recent posting on arxiv.org by three Argentinian mathematicians on the quality of fits to power-law distributions using the standard least-squares method. Given my own interest in power laws, and my own work on statistical methods for characterizing them, this paper confirms what many of us in the field have known in practice for some time: least-squares is a horrible way to measure the scaling exponent of a power law. From the abstract:
In this work we study the condition number of the least square matrix corresponding to scale free networks. We compute a theoretical lower bound of the condition number which proves that they are ill conditioned. Also, we analyze several matrices from networks generated with the linear preferential attachment model showing that it is very difficult to compute the power law exponent by the least square method due to the severe lost of accuracy expected from the corresponding condition numbers.
And finally, although touch-sensitive screens are (almost) everywhere today, they are merely single-touch interfaces. That is, you can only touch them in one place at a time. Enter multi-touch interfaces (direct link to the video), developed by a team at NYU. Reminscient of the interfaces seen in Minority Report and The Island, in which table-top or wall-sized displays interact with users via "gestures". I can't wait until I can get one of these things.
posted February 13, 2006 04:34 AM in Scientifically Speaking | permalink | Comments (1)
January 30, 2006
Selecting morality
I've been musing a little more about Dr. Paul Bloom's article on the human tendency to believe in the supernatural. (See here for my last entry on this.) The question that's most lodged in my mind right now is thus, What if the only way to have intelligence like ours, i.e., intelligence that is capable of both rational (science) and irrational (art) creativity, is to have these two competing modules, the one that attributes agency to everything and the one that coldly computes the physical outcome of events? If this is true, then the ultimate goal of creating "intelligent" devices may have undesired side-effects. If futurists like Jeff Hawkins are right that an understanding of the algorithms that run the brain are within our grasp, then we may see these effects within our lifetime. Not only will your computer be able to tell when you're unhappy with it, you may need to intuit when it's unhappy with you! (Perhaps because you ignored it for several days while you tended to your Zen rock garden, or perhaps you left it behind while you went to the beach.)
This is a somewhat entertaining line of thought, with lots of unpleasant implications for our productivity (imagine having to not only keep track of the social relationships of your human friends, but also of all the electronic devices in your house). But, Bloom's discussion raises another interesting question. If our social brain evolved to manage the burgeoning collection of inter-personal and power relationships in our increasingly social existence, and if our social brain is a key part of our ability to "think" and imagine and understand the world, then perhaps it is hard-wired with certain moralistic beliefs. A popular line of argument between theists and atheists is the question of, If one does not get one's sense of morality from God, what is to stop everyone from doing exactly as they please, regardless of its consequences? The obligatory examples of such immoral (amoral?) behavior are rape and murder - that is, if I don't have in me the fear of God and his eternal wrath, what's to stop me from running out in the street and killing the first person I see?
Perhaps surprisingly, as the philosopher Daniel Dennett (Tufts University) mentions in this half-interview, half-survey article from The Boston Globe, being religious doesn't seem to have any impact on a person's tendency to do clearly immoral things that will get you thrown in jail. In fact, many of those whom are most vocal about morality (e.g., Pat Robertson) are themselves cravenly immoral, by any measure of the word (a detailed list of Robertson's crimes; a brief but humorous summary of them (scroll to bottom; note picture)).
Richard Dawkins, the well-known British ethologist and atheist, recently aired a two-part documentary, of his creation, on the BBC's Channel 4 attempting to explore exactly this question. (Audio portion for both episodes available here and here, courtesy of onegoodmove.org.) He first posits that faith is the antithesis of rationality - a somewhat incendiary assertion on the face of it. However, consider that faith is, by definition, the belief in something for which there is no evidence or for which there is evidence against, while rationally held beliefs are those based on evidence and evidence alone. In my mind, such a distinction is rather important for those with any interest in metaphysics, theology or that nebulous term, spirituality. Dawkins' argument goes very much along the lines of Stephen Weinberg, Nobel Prize in physics, who once said "Religion is an insult to human dignity - without it you'd have good people doing good things and evil people doing evil things. But for good people to do evil things it takes religion." However, Dawkins' documentary points at a rather more fundamental question, Where does morality comes from if not from God, or the cultural institutions of a religion?
This question was recently, although perhaps indirectly, explored by Jessica Flack and her colleagues at the Santa Fe Institute; published in Nature last week (summary here). Generally, Flack et al. studied the importance of impartial policing, by authoritative members of a pigtailed macaque troupe, to the cohesion and general health of the troupe as a whole. Their discovery that all social behavior in the troupe suffers in the absence of these policemen shows that they serve the important role of regulating the self-interested behavior of individuals. That is, by arbitrating impartially among their fellows in conflicts, when there is no advantage or benefit to them for doing so, the policemen demonstrate an innate sense of a right and wrong that is greater than themselves.
There are two points to take home from this discussion. First, that humans are not so different from other social animals in that we need constant reminders of what is "moral" in order for society to function. But second, if "moral" behavior can come from the self-interested behavior of individuals in social groups, as is the case for the pigtailed macaque, then it needs no supernatural explanation. Morality can thus derive from nothing more than the natural implication of real consequences, to both ourselves and others, for certain kinds of behaviors, and the observation that those consequences are undesirable. At its heart, this is the same line of reasoning for religious systems of morality, except that the undesirable consequences are supernatural, e.g., burning in Hell, not getting to spend eternity with God, etc. But clearly, the pigtailed macaques can be moral without God and supernatural consequences, so why can't humans?
J. C. Flack, M. Girvan, F. B. M. de Waal and D. C. Krakauer, "Policing stabilizes construction of social niches in primates." Nature 439, 426 (2006).
Update, Feb. 6th: In the New York Times today, there is an article about how quickly a person's moral compass can shift when certain unpalatable acts are sure to be done (by that person) in the near future, e.g., being employed as a part of the State capital punishment team, but being (morally) opposed to the death penalty. This reminds me of the Milgram experiment (no, not that one), which showed that a person's moral compass could be broken simply by someone with authority pushing it. In the NYTimes article, Prof. Bandura (Psychology, Stanford) puts it thus:
It's in our ability to selectively engage and disengage our moral standards, and it helps explain how people can be barbarically cruel in one moment and compassionate the next.
(Emphasis mine.) With a person's morality being so flexible, it's no wonder that constant reminders (i.e., policing) are needed to keep us behaving in a way that preserves civil society. Or, to use the terms theists prefer, it is policing, and the implicit terrestrial threat embodied by it, that keeps us from running out in the street and doing profane acts without a care.
Update, Feb. 8th: Salon.com has an interview with Prof. Dennet of Tufts University, a strong advocate of clinging to rationality in the face of the dangerous idea that everything that is "religious" in its nature is, by definition, off-limits to rational inquiry. Given that certain segments of society are trying (and succeeding) to expand the range of things that fall into that domain, Dennet is an encouragingly clear-headed voice. Also, when asked how we will know right from wrong without a religious base of morals, he answers that we will do as we have always done, and make our own rules for our behavior.
posted January 30, 2006 02:43 AM in Thinking Aloud | permalink | Comments (3)
January 05, 2006
Is God an accident?
This is the question that Dr. Paul Bloom, professor of psychology at Yale, explores in a fascinating exposé in The Atlantic Monthly on the origins of religion, as evidence by a belief in supernatural beings through a neurological basis of our ability to attritute agency. He begins,
Despite the vast number of religions, nearly everyone in the world believes in the same things: the existence of a soul, an afterlife, miracles, and the divine creation of the universe. Recently psychologists doing research on the minds of infants have discovered two related facts that may account for this phenomenon. One: human beings come into the world with a predisposition to believe in supernatural phenomena. And two: this predisposition is an incidental by-product of cognitive functioning gone awry. Which leads to the question ...
The question being, of course, whether the nearly universal belief in these things is an accident of evolution optimizing brain-function for something else entirely.
Belief in the supernatural is an overly dramatic way to put the more prosaic idea that we see agency (willful acts, as in, free will) where none exists. That is, consider the extreme ease with which we anthropomorphize inanimate objects like the Moon ("O, swear not by the moon, the fickle moon, the inconstant moon, that monthly changes in her circle orb, Lest that thy love prove likewise variable." Shakespeare Romeo and Juliet 2:ii), complex objects like our computers (intentionally confounding us, colluding to ruin our job or romantic prospects, etc.), and living creatures whom we view as little more than robots ("smart bacteria"). Bloom's consideration of the question of why is this innate tendency apparently universal among humans is a fascinating exploration of both evolution, human behavior and our pathologies. At the heart of his story arc, he considers whether easy attribution of agency provides some other useful ability in terms of natural selection. In short, he concludes that yes, our brain is hardwired to see intention and agency where none exists because viewing the world through this lens made (makes) it easier for us to manage our social connections and responsibilities, and the social consequences of our actions. For instance, consider a newborn - Bloom desribes experiments that show that
when twelve-month-olds see one object chasing another, they seem to understand that it really is chasing, with the goal of catching; they expect the chaser to continue its pursuit along the most direct path, and are surprised when it does otherwise.
But more generally,
Understanding of the physical world and understanding of the social world can be seen as akin to two distinct computers in a baby's brain, running separate programs and performing separate tasks. The understandings develop at different rates: the social one emerges somewhat later than the physical one. They evolved at different points in our prehistory; our physical understanding is shared by many species, whereas our social understanding is a relatively recent adaptation, and in some regards might be uniquely human.
This doesn't directly resolve the problem of liberal attribution of agency, which is the foundation of a belief in supernatural beings and forces, but Bloom resolves this by pointing out that because these two modes of thinking evolved separately and apparently function independently, we essentially view people (whose agency is understood by our "social brain") as being fundamentally different from objects (whose behavior is understood by our "physics brain"). This distinction makes it possible for us to envision "soulless bodies and bodiless souls", e.g., zombies and ghosts. With this in mind, certain recurrent themes in popular culture become eminently unsurprising.
So it seems that we are all dualists by default, a position that our everyday experience of consciousness only reinforces. Says Bloom, "We don't feel that we are our bodies. Rather, we feel that we occupy them, we possess them, we own them." The problem of having two modes of thinking about the world is only exacerbated by the real world's complexity, i.e., is a dog's behavior best understood with the physics brain or the social brain?, is a computer's behavior best understood with... you get the idea. In fact, it seems that you could argue quite convincingly that much of modern human thought (e.g., Hobbes, Locke, Marx and Smith) has been an exploration of the tension between these modes; Hobbes in particular sought a physical explanation of social organization. This also points out, to some degree, why it is so difficult for humans to be rational beings, i.e., there is a fundamental irrationality in the way we view the world that is difficult to first be aware of, and then to manage.
Education, or more specifically a training in scientific principles, can be viewed as a conditioning regiment that encourages the active management of the social brain's tendency to attribute agency. For instance, I suspect that the best scientists use their social mode of thinking when analyzing the interaction of various forces and bodies to make the great leaps of intuition that yield true steps forward in scientific understanding. That is, the irrationality of the two modes of thinking can, if engaged properly, be harnessed to extend the domain of rationality. There is certainly a great many suggestive anecdotes for this idea, and it suggests that if we ever want computers to truly solve problems the way humans do (as opposed to simply engaging in statistical melee), they will need to learn how to be more irrational, but in a careful way. I certainly wouldn't want my laptop to suddenly become superstitious about say, being plugged into the Internet!
posted January 5, 2006 04:50 PM in Scientifically Speaking | permalink | Comments (0)
December 19, 2005
On modeling the human response time function; Part 3.
Much to my surprise, this morning I awoke to find several emails in my inbox apparently related to my commentary on the Barabasi paper in Nature. This morning, Anders Johansen pointed out to myself and Luis Amaral (I can only assume that he has already communicated this to Barabasi) that in 2004 he published an article entitled Probing human response times in Physica A about the very same topic using the very same data as that of Barabasi's paper. In it, he displays the now familiar heavy-tailed distribution of response times and fits a power law of the form P(t) ~ 1/(t+c) where c is a constant estimated from the data. Asymptotically, this is the same as Barabasi's P(t) ~ 1/t; it differs in the lower tail, i.e., for t < c where it scales more uniformly. As an originating mechanism, he suggests something related to a spin-glass model of human dynamics.
Although Johansen's paper raises other issues, which I'll discuss briefly in a moment, let's step back and think about this controversy from a scientific perspective. There are two slightly different approaches to modeling that are being employed to understand the response-time function of human behavior. The first is a purely "fit-the-data" approach, which is largely what Johansen has done, and certainly what Amaral's group has done. The other, employed by Barabasi, uses enough data analysis to extract some interesting features, posits a mechanism for the origin of those and then sets about connecting the two. The advantage of developing such a mechanistic explanation is that (if done properly) it provides falsifiable hypotheses and can move the discussion past simple data-analysis techniques. The trouble begins, as I've mentioned before, when either a possible mechanistic model is declared to be "correct" before being properly vetted, or when an insufficient amount of data analysis is done before positing a mechanism. This latter kind of trouble allows for a debate over how much support the data really provides to the proposed mechanism, and is exactly the source of the exchange between Barabasi et al. and Stouffer et al.
I tend to agree with the idea implicitly put forward by Stouffer et al.'s comment that Barabasi should have done more thorough data analysis before publishing, or alternatively, been a little more cautious in his claims of the universality of his mechanism. In light of Johansen's paper and Johansen's statement that he and Barabasi spoke at the talk in 2003 where Johansen presented his results, there is now the specter that either previous work was not cited that should have been, or something more egregious happened. While not to say that this aspect of the story isn't an important issue in itself, it is a separate one from the issues regarding the modeling, and it is those with which I am primarily concerned. But, given the high profile of articles published in journals like Nature, this kind of gross error in attribution does little to reassure me that such journals are not aggravating certain systemic problems in the scientific publication system. This will probably be a topic of a later post, if I ever get around to it. But let's get back to the modeling questions.
Seeking to be more physics and less statistics, the ultimate goal of such a study of human behavior should be to understand the mechanism at play, and at least Barabasi did put forward and analyze a plausible suggestion there, even if a) he may not have done enough data analysis to properly support it or his claims of universality, and b) his model assumes some reasonably unrealistic behavior on the part of humans. Indeed, the former is my chief complaint about his paper, and why I am grateful for the Stouffer et al. comment and the ensuing discussion. With regard to the latter, my preference would have been for Barabasi to have discussed the fragility of his model with respect to the particular assumptions he describes. That is, although he assumes it, humans probably don't assign priorities to their tasks with anything like a uniformly random distribution and nor do humans always execute their highest priority task next. For instance, can you decide, right now without thinking, what the most important email in your inbox is at this moment? Instead, he commits the crime of hubris and neglects these details in favor of the suggestiveness of his model given the data. On the other hand, regardless of their implausibility, both of these assumptions about human behavior can be tested through experiments with real people and through numerical simulation. That is, these assumptions become predictions about the world that, if they fail to agree with experiment, would falsify the model. This seems to me an advantage of Barabasi's mechanism over that proposed by Johansen, which, by relying on a spin glass model of human behavior, seems quite trickier to falsify.
But let's get back to the topic of the data analysis and the argument between Stouffer et al. and Barabasi et al. (now also Johansen) over whether the data better supports a log-normal or a power-law distribution. The importance of this point is that if the log-normal is the better fit, then the mathematical model Barabasi proposes cannot be the originating mechanism. From my experience with distributions with heavy tails, it can be difficult to statistically (let alone visually) distinguish between a log-normal and various kinds of power laws. In human systems, there is almost never enough data (read: orders of magnitude) to distinguish these without using standard (but sophisticated) statistical tools. This is because for any finite sample of data from an asymptotic distribution, there will be deviations that will blur the functional form just enough to look rather like the other. For instance, if you look closely at the data of Barabasi or Johansen, there are deviations from the power-law distribution in the far upper tail. Stouffer et al. cite these as examples of the poor fit of the power law and as evidence supporting the log-normal. Unfortunately, they could simply be due to deviations due to finite-sample effects (not to be confused with finite-size effects), and the only way to determine if they could have been is to try resampling the hypothesized distribution and measuring the sample deviation against the observed one.
The approach that I tend to favor for resolving this kind of question combines a goodness-of-fit test with a statistical power test to distinguish between alternative models. It's a bit more labor-intensive than the Bayesian model selection employed by Stouffer et al., but this approach offers, in addition to others that I'll describe momentarily, the advantage of being able to say that, given the data, neither model is good or that both models are good.
Using Monte Carlo simulation and something like the Kolmogorov-Smirnov goodness-of-fit test, you can quantitatively gauge how likely a random sample drawn from your hypothesized function F (which can be derived using maximum likelihood parameter estimation or by something like a least-squares fit; it doesn't matter) will have a deviation from F at least as big as the one observed in the data. By then comparing the deviations with an alternative function G (e.g., a power law versus a log-normal), you get a measure of the power of F over G as an originating model of the data. For heavy-tailed distributions, particularly those with a sample-mean that converges slowly or never at all (as is the case for something like P(t) ~ 1/t), sampling deviations can cause pretty significant problems with model selection, and I suspect that the Bayesian model selection approach is sensitive to these. On the other hand, by incorporating sampling variation into the model selection process itself, one can get an idea of whether it is even possible to select one model over another. If someone were to use this approach to analyze the data of human response times, I suspect that the pure power law would be a poor fit (the data looks too curved for that), but that the power law suggested in Johansen's paper would be largely statistically indistinguishable from a log-normal. With this knowledge in hand, one is then free to posit mechanisms that generate either distribution and then proceed to validate the theory by testing its predictions (e.g., its assumptions).
So, in the end, we may not have gained much in arguing about which heavy-tailed distribution the data likely came from, and instead should consider whether or not an equally plausible mechanism for generating the response-time data could be derived from the standard mechanisms for producing log-normal distributions. If we had such an alternative mechanism, then we could devise some experiments to distinguish between them and perhaps actually settle this question like scientists.
As a closing thought, my interest in this debate is not particularly in its politics. Rather, I think this story suggests some excellent questions about the practice of modeling, the questions a good modeler should ponder on the road to truth, and some of the pot holes strewn about the field of complex systems. It also, unfortunately, provides some anecdotal evidence of some systemic problems with attribution, the scientific publishing industry and the current state of peer-review at high-profile, fast turn-around-time journals.
References for those interested in reading the source material.
A. Johansen, "Probing human response times." Physica A 338 (2004) 286-291.
A.-L. Barabasi, "The origin of bursts and heavy tails in human dynamics." Nature 435 (2005) 207-211.
D. B. Stouffer, R. D. Malmgren and L. A. N. Amaral "Comment on 'The origin of bursts and heavy tails in human dynamics'." e-print (2005).
J.-P. Eckmann, E. Moses and D. Sergi, "Entropy of dialogues creates coherent structures in e-mail traffic." PNAS USA 101 (2004) 14333-14337.
A.-L. Barabasi, K.-I. Goh, A. Vazquez, "Reply to Comment on 'The origin of bursts and heavy tails in human dynamics'." e-print (2005).
posted December 19, 2005 04:32 PM in Scientifically Speaking | permalink | Comments (0)
November 27, 2005
Irrational exuberance plus indelible sniping yields delectable entertainment
In a past entry (which sadly has not yet scrolled off the bottom of the front page - sad because it indicates how infrequently I am posting these days), I briefly discussed the amusing public debate by Barabasi et al. and Souffer et al. over Barabasi's model of correspondence. At that point, I found the exchange amusing and was inclined to agree with the response article. However, let me rehash this topic and expose a little more light on the subject.
From the original abstract of the article posted on arxiv.org by Barabasi:
Current models of human dynamics, used from risk assessment to communications, assume that human actions are randomly distributed in time and thus well approximated by Poisson processes. In contrast, ... the timing of many human activities, ranging from communication to entertainment and work patterns, [are] ... characterized by bursts of rapidly occurring events separated by long periods of inactivity. Here we show that the bursty nature of human behavior is a consequence of a decision based queuing process: when individuals execute tasks based on some perceived priority, the timing of the tasks will be heavy tailed, most tasks being rapidly executed, while a few experience very long waiting times.
(Emphasis is mine.) Barabasi is not one to shy away from grand claims of universality. As such, he epitomizes the thing that many of those outside of the discipline hate about physicists, i.e., their apparent arrogance. My opinion is that most physicists accused of intellectual arrogant are misunderstood, but that's a topic for another time.
Stouffer et al. responded a few months after Barabasi's original idea, as published in Nature, with the following (abstract):
In a recent letter, Barabasi claims that the dynamics of a number of human activities are scale-free. He specifically reports that the probability distribution of time intervals tau between consecutive e-mails sent by a single user and time delays for e-mail replies follow a power-law with an exponent -1, and proposes a priority-queuing process as an explanation of the bursty nature of human activity. Here, we quantitatively demonstrate that the reported power-law distributions are solely an artifact of the analysis of the empirical data and that the proposed model is not representative of e-mail communication patterns.
(Emphasis is mine.) In this comment, Stouffer et al. strongly criticize the data analysis that Barabasi uses to argue for the plausibility and, indeed, the correctness of his priority-based queueing model. I admit that when I first read Barabasi's queueing model, I thought that surely the smart folks who have been dealing with queueing theory (a topic nearly a century old!) knew something like this already. Even if that were the case, the idea certainly qualifies as interesting, and I'm happy to see a) the idea published, although Nature was likely not the appropriate place and b) the press attention that Barabasi has brought to the discipline of complex systems and modeling. Anyway, the heart of the data-analysis based critique of Barabasi's work lies in distinguishing two different kinds of heavy-tailed distributions: the log-normal and the power law. Because of a heavy tail is an asymptotic property, these two distributions can be extremely difficult to differentiate when the data only spans a few orders of magnitude (as is the case here). Fortunately, statisticians (and occasionally, myself) enjoy this sort of thing. Stouffer et al. employ such statistical tools in the form of Bayesian model selection to choose between the two hypotheses and find the evidence of the power law lacking. It was quite dissatisfying, however, that Stouffer et al. neglected to discuss their model selection procedure in detail, and instead chose to discuss the politicking over Barabasi's publication in Nature.
And so, it should come as no surprise that a rejoinder from Barabasi was soon issued. With each iteration of this process, the veneer of professionalism cracks away a little more:
[Stouffer et al.] revisit the datasets [we] studied..., making four technical observations. Some of [their] observations ... are based on the authors' unfamiliarity with the details of the data collection process and have little relevance to [our] findings ... and others are resolved in quantitative fashion by other authors.
In the response, Barabasi discusses the details of the dataset that Stouffer et al. fixated on: that the extreme short-time behavior of the data is actually an artifact of the way messages to multiple recipients were logged. They rightly emphasize that it is the existence of a heavy tail that is primarily interesting, rather than its exact form (of course, Barabasi made some noise about the exact form in the original paper). However, it is not sufficient to simply observe a heavy tail, posit an apparently plausible model that produces some kind of such tail and then declare victory, universality and issue a press release. (I'll return to this thought in a moment.) As a result, Barabasi's response, while clarifying a few details, does not address the fundamental problems with the original work. Problems that Stouffer et al. seem to intuit, but don't directly point out.
A month ago, Suresh over at the Geomblog published a comment on the controversy by Michael Mitzenmacher (whose work I greatly enjoy) in which he touches briefly on the real issue here.
While the rebuttal suggests the data is a better fit for the lognormal distribution, I am not a big believer in the fit-the-data approach to distinguish these distributions. The Barabasi paper actually suggested a model, which is nice, although the problem of how to verify such a model is challenge... This seems to be the real problem. Trust me, anyone can come up with a power law model. The challenge is figuring out how to show your model is actually right.
That is, first and foremost, the bursty nature of human activity is odd and, in that alluring voice only those fascinated by complex systems can hear, begs for an explanation. Second, a priority-based queueing process is merely one possible explanation (out of perhaps many) for the heaviness and burstiness. The emphasis is to point out that there is a real difficulty in nailing down causal mechanisms in human systems. often the best we can do is concoct a theory and see if the data supports it. That is, it is exceedingly difficult to go beyond mere plausibility without an overwhelming weight of empirical evidence and, preferably, the vetting of falsifiable hypotheses. The theory of natural selection is an excellent example that has been validated by just such a method (and continues to be). Unfortunately, simply looking at the response time statistics for email or letters by Darwin or Einstein, while interesting from the socio-historical perspective, does not prove the model. On the contrary: it merely suggests it.
That is, Barabasi's work demonstrates the empirical evidence (heavy-tails in the response times of correspondence) and offers a mathematical model that generates statistics of a similar form. It does not show causality, nor does it provide falsifiable hypotheses by which it could be invalidated. Barabasi's work in this case is suggestive but not explanatory, and should be judged accordingly. To me, it seems that the contention over the result derives partly from the overstatement of its generality, i.e., the authors claims their model to be explanatory. Thus, the argument over the empirical data is really just an argument about how much plausibility it imparts to the model. Had Barabasi gone beyond suggestion, I seriously doubt the controversy would exist.
Considering the issues raised here, personally, I think it's okay to publish a results that is merely suggestive so long as it is honestly made, diligently investigated and embodies a compelling and plausible story. That is to say that, ideally, authors should discuss the weakness of their model, empirical results and/or mathematical analysis, avoid overstating the generality of the result (sadly, a frequent problem in many of the papers I referee), carefully investigate possible biases and sources of error, and ideally, discuss alternative explanations. Admittedly, this last one may be asking a bit much. In a sense, these are the things I think about when I read any paper, but particularly when I referee something. This thread of thought seems to be fashionable right now, as I just noticed that Cosma's latest post discusses criteria for accepting or rejecting papers in the peer review process.
posted November 27, 2005 05:00 AM in Scientifically Speaking | permalink | Comments (0)
November 13, 2005
Graduate students are cool.
The sign of a good teacher is being able to convey the importance of their subject in a manner that engages their audience, making them walk away knowing (not just believing) that they've learned something valuable and novel, and wondering what other interesting things may lay down the path just revealed to them. I like to call this the "Wow"-factor, and it's what gets people engaged in a subject, whether they are just beginning their intellectual journey or have worn through several pairs of shoes already (although, probably for different reasons and in different ways).
The Value of Control Groups in Causal Inference (and Breakfast Cereal) by Gary King of The Social Science Statistics Blog, which I may have to add to my regular list now. King describes a great way to teach a fundamentally important piece of knowledge - the importance of a null-model (or a control group).
A few years ago, I taught the following lesson in my daughter's kindergarden class and my graduate methods class in the same week. It worked pretty well in both. Anyone who has a kid in kindergarten, some good graduate students, or both, might want to try this. It was especially fun for the instructor.
To start, I hold up some nails and ask "does everyone likes to eat nails?" The kindergarten kids scream, "Nooooooo." The graduate students say "No," trying to look cool. I say I'm going to convince them otherwise.
King also recommends Teaching Statistics: A Bag of Tricks for those of us interested in more compelling demos to dislodge our students from their cynicism.
posted November 13, 2005 04:29 AM in Humor | permalink | Comments (0)
October 27, 2005
Links, links, links.
The title is perhaps a modern variation on Hamlet's famous "words, words, words" quip to Lord Polonius. Some things I've read recently, with mild amounts of editorializing:
Tim Burke (History professor at Swarthmore College) recently discussed (again) his thoughts on the future of academia. That is, why would it take for college costs to actually decrease. I assume this arises at least partially as a result of the recent New York Times article on the ever increasing tuition rates for colleges in this country. He argues that modern college costs rise at least partially as a result of pressure from lawsuits and parents to provide in loco parentis to the kids attending. Given the degree of hand-holding I experienced at Haverford, perhaps the closest thing to Swarthmore without actually being Swat, this makes a lot of sense. I suspect, however, that tuition prices will continue to increase apace for the time being, if only because enrollment rates continue to remain high.
Speaking of high enrollment rates, Burke makes the interesting point
... the more highly selective a college or university is in its admission policies, the more useful it is for an employer as a device for identifying potentially valuable employees, even if the employer doesn’t know or care what happened to the potential employee while he or she was a student.
This assertion belies an assumption about whose pervasiveness I wonder. Basically, Burke is claiming that selectivity is an objective measure of something. Indeed, it is. It's an objective measure of the popularity of the school, filtered through the finite size of a freshman class that the school can reasonably admit, and nothing else. A huge institution could catapult itself higher in the selectivity rankings simply by cutting the number of students it admits.
Barabasi's recent promotion of his ideas about the relationship between "bursty behavior" among humans and our managing a queue of tasks to accomplish continues to generate press. New Scientist and Physics Web both picked the piece of work on Darwin's, Einstein's and modern email-usage communication patterns. To briefly summarize from Barabasi's own paper:
Here we show that the bursty nature of human behavior is a consequence of a decision based queueing process: when individuals execute tasks based on some perceived priority, the timing of the tasks will be heavy tailed, most tasks being rapidly executed, while a few experience very long waiting times.
A.-L. Barabasi (2005) "The origin of bursts and heavy tails in human dynamics." Nature 435, 207.
That is, the response times are described by a power law with exponent between 1.0 and 1.5. Once again, power laws are everywhere. (NB: In the interest of full disclosure, power laws are one focus of my research, although I've gone on record saying that there's something of an irrational exuberance for them these days.) To those of you experiencing power-law fatigue, it may not come as any surprise that last night in the daily arXiv mailing of new work, a very critical (I am even tempted to say scathing) comment on Barabasi's work appeared. Again, to briefly summarize from the comment:
... we quantitatively demonstrate that the reported power-law distributions are solely an artifact of the analysis of the empirical data and that the proposed model is not representative of e-mail communication patterns.
D. B. Stouffer, R. D. Malmgren and L. A. N. Amaral (2005) "Comment on The origin of bursts and heavy tails in human dynamics." e-print.
There are several interesting threads imbedded in this discussion, the main one being on the twin supports of good empirical research: 1) rigorous quantitative tools for data analysis, and 2) a firm basis in empirical and statistical methods to support whatever conclusions you draw with aforementioned tools. In this case, Stouffer, Malmgren and Amaral utilize Bayesian model selection to eliminate the power law as a model, and instead show that the distributions are better described by a log-normal distribution. This idea of the importance of good tools and good statistics is something I've written on before. Cosma Shalizi is a continual booster of these issues, particularly among physicists working in extremal statistics and social science.
And finally, Carl Zimmer, always excellent, on the evolution of language.
[Update: After Cosma linked to my post, I realized it needed a little bit of cleaning up.]
posted October 27, 2005 01:23 AM in Thinking Aloud | permalink | Comments (0)
October 17, 2005
Some assembly required.
While browsing the usual selection of online venues for news about the world, I came across a reference to a recent statistical study of American and European knowledge of science and technology, conducted in part by the National Science Foundation. The results, as you my dear reader may guess, were depressing. Here are a few choice excerpts.
Conclusions about technology and science:
Technology has become so user friendly it is largely "invisible." Americans use technology with a minimal comprehension of how or why it works or the implications of its use or even where it comes from. American adults and children have a poor understanding of the essential characteristics of technology, how it influences society, and how people can and affect its development.
and
NSF surveys have asked respondents to explain in their own words what it means to study something scientifically. Based on their answers, it is possible to conclude that most Americans (two-thirds in 2001) do not have a firm grasp of what is meant by the scientific process. This lack of understanding may explain why a substantial portion of the population believes in various forms of pseudoscience.
Regarding evolution (a topical topic; see also several of my entries):
Response to one of the questions, "human beings, as we know them today, developed from earlier species of animals," may reflect religious beliefs rather than actual knowledge about science. In the United States, 53 percent of respondents answered "true" to that statement in 2001, the highest level ever recorded by the NSF survey. (Before 2001, no more than 45 percent of respondents answered "true.") The 2001 result represented a major change from past surveys and brought the United States more in line with other industrialized countries about the question of evolution.
Yet, there is hope
... the number of people who know that antibiotics do not kill viruses has been increasing. In 2001, for the first time, a majority (51 percent) of U.S. respondents answered this question correctly, up from 40 percent in 1995. In Europe, 40 percent of respondents answered the question correctly in 2001, compared with only 27 percent in 1992.
Also, the survey found that belief in devil possession declined between 1990 and 2001. On the other hand, belief in other paranormal phenomena increased, and
... at least a quarter of the U.S. population believes in astrology, i.e., that the position of the stars and planets can affect people's lives. Although the majority (56 percent) of those queried in the 2001 NSF survey said that astrology is "not at all scientific," 9 percent said it is "very scientific" and 31 percent thought it is "sort of scientific".
In the United States, skepticism about astrology is strongly related to level of education [snip]. In Europe, however, respondents with college degrees were just as likely as others to claim that astrology is scientific.
Aside from being thoroughly depressing for a booster of science and rationalism such as myself, this suggests that, not only do Westerners have little conception of what it means to be "scientific" or what "technology" actually is, but Western life does not require people to have any mastery of scientific or technological principles. That is, one can get along just fine in life while being completely ignorant of why things actually happen or how to rigorously test hypotheses. Of course, this is a little bit of a circular problem, since if no one understands how things work, people will design user-friendly things that don't need to be understood in order to function. That is, those who are not ignorant of how the world works provide no incentive to those who are to change their ignorant ways. Of course, aren't we all ignorant of the complicated details of many of the wonders that surround us? Perhaps the crucial difference lies not in being ignorant itself, but in being unwilling to seek out the truth (especially when it matters).
The conclusions of the surveys do nothing except bolster my belief that rational thinking and careful curiosity are not the natural mode of human thought, and that the Enlightenment was a weird and unnatural turn of events. Perhaps one of the most frightening bits of the survey was the following statement
there is no evidence to suggest that legislators or their staff are any more technologically literate than the general public.
posted October 17, 2005 08:52 PM in Thinking Aloud | permalink | Comments (0)
July 26, 2005
Global patterns in terrorism; part III
Neil Johnson, a physicist at Lincoln College of Oxford University, with whom I've been corresponding about the mathematics of terrorism for several months, has recently put out a paper that considers the evolution of the conflicts in Iraq and Colombia. The paper (on arxiv, here) relies heavily on the work Maxwell Young and I did on the power law relationship between the frequency and severity of terrorist attacks worldwide.
Neil's article, much like our original one, has garnered some attention among the popular press, so far yielding an article at The Economist (July 21st) that also heavily references our previous work. I strongly suspect that there will be more, particularly considering the July 7th terrorist bombings in London, and Britain's continued conflicted relationship with its own involvement in the Iraq debacle.
Given the reasonably timely attention these analyses are garnering, the next obvious step in this kind of work is to make it more useful for policy-makers. What does it mean for law enforcement, for the United Nations, for forward-looking politicians that terrorism (and, if Neil is correct in his conjecture, the future of modern armed geopolitical conflict) has this stable mathematical structure? How should countries distribute their resources so as to minimize the fallout from the likely catastrophic terrorist attacks of the future? These are the questions that scientific papers typically stay as far from as possible - attempting to answer them takes one out of the scientific world and into the world of policy and politics (shark infested waters for sure). And yet, in order for this work to be relevant outside the world of intellectual edification, some sort of venture must be made.
posted July 26, 2005 12:57 AM in Scientifically Speaking | permalink | Comments (0)
May 05, 2005
The joys of unfettered research
Although I diligently try to keep up with the new papers in my field, there are just so many to read... yet, some are more pleasant than others. In fact, some could be said to be downright pleasurable, such as a mathematical model of scientists writing papers (and citing other papers). Or an empirical study of the frequency of natural numbers on the World Wide Web. Who says science is humorless?
Update: In following the power-law exuberance, power laws exist in the income distribution of movies in the United States. This doesn't suprise me one bit actually, since there is pretty strong evidence that humans prefer a power law in the popularity of things (e.g., power-law degree distribution in the sales of books, etc.). Somehow, I doubt that power laws of this kind will not continue to make headlines...
Friend Dennis Chao (of psdoom fame; another shining example of the fruits of unfettered research) pointed me to this amusing bit of research in 2003 by a pair of Canadian psychologists on the effects of a pretty female face on heterosexual men's ability to accurately estimate the future value of goods. From the article:
A sex difference in discounting is predictable. Because men have always had some chance of gaining fitness from short-term expenditures of mating effort, whereas successful reproduction typically requires more prolonged parental investment by women, men should have evolved to discount the future more steeply than women, and sex differences in age-specific mortality confirm this expectation (e.g. Arias 2002). Men also have higher discount rates than women in choices of monetary rewards (Kirby & Marakovic 1996).
and, on their results,
As predicted, discounting increased signif icantly in men who viewed attractive women, but not in men who viewed unattractive women or women who viewed men; viewing cars produced a different pattern of results.
Straight men's weaknesses: cars and pretty girls...
posted May 5, 2005 04:23 AM in Scientifically Speaking | permalink | Comments (0)
April 30, 2005
Dawkins and Darwin and Zebra Finches
As if it weren't already painfully obvious from my previous posts, let me make it more obvious now:
Salon.com has an excellent and entertaining interview with the indomitable Richard Dawkins. I've contemplated picking up several of his books (e.g., The Selfish Gene, and The Blind Watchmaker), but have not ever quite gotten around to it. Dawkins speaks a little about his new book, sure to inflame more hatred among religious bigots, and the trend of human society toward enlightenment. (Which I'm not so confident about, these days. Dawkins does make the point that it's largely the U.S. that's having trouble keeping both feet on the road to enlightenment.)
In a similar vein, science write Carl Zimmer (NYTimes, etc.) keeps a well-written blog called The Loom in which he discusses the ongoing battle between the forces of rationality and the forces of ignorance. A particularly excellent piece of his writing concerns the question of gaps in the fossil record and how the immune system provides a wealth of evidence for evolution. Late last year, this research article appeared in the Proceedings of the National Academy of Science, which is the article which Zimmer discusses.
Finally, in my continuing facination with the things that bird brains do, scientists at MIT recently discovered that a small piece of the bird brain (in this case, the very agreeable zebra finch) helps young songbirds learn their species' songs by regularly jolting their understanding of the song pattern so as to keep it from settling down to quickly (for the physicists, this sounds oddly like simulated annealing, does it not?). That is, the jolts keep the young bird brain creative and trying new ways to imitate the elders. This reminds me of a paper I wrote for my statistical mechanics course at Haverford in which I learned that spin-glass models of recurrent neural networks with Hebbian learning require some base level of noise in order to function properly (and not settle into a glassy state with fixed domain boundaries). Perhaps the reason we have greater difficulty learning new things as we get older is because the level of mental noise decreases with time?
posted April 30, 2005 04:15 AM in Obsession with birds | permalink | Comments (0)
March 13, 2005
The virtues of playing dice
In physics, everyone (well, almost) assumes that true randomness does exist, because so much of modern physics is built on this utilitarian assumption. Despite some people being very determined to do so, physicists have not determined that determinism isn't the rule of the universe; all they have is a bunch of empirical evidence against it (which for most physicists, is enough). So-called "hidden variable" models have been a popular way (e.g., here, here and here) to probe this question in a principled fashion. They're based on the premise that if in, for instance quantum mechanics, there was some hidden variable that we've just been too stupid to figure out yet, then there must be regularities (correlations) in physical reality that betray its existence. Yet so far, no hidden variable model has prevailed against quantum mechanics and apparent true randomness. (For an excellent discussion of randomness and physics, see Stephen Hawkings' lectures on the subject, especially if you wonder about things like black holes.)
In computer science, everyone knows that there's no way to deterministically create a truly random number. Amusingly, computer scientists often assume that physicists have settled the existence of randomness; yet, why hasn't anyone yet stuck a black-body radiation device inside of each computer (which would be ever-so-useful)? Perhaps getting true randomness is trickier than they have come to believe. In the meantime, those of us who want randomness in computer programs have to make do with the aptly-named pseudo-random number generators (some of which are extremely sophisticated) that create strings of numbers that only appear to be random. (It can be very dangerous to forget that pseudo-random number generators are completely deterministic, as I've blogged about before.) It frequently surprises me that in computer science, most people appear to believe that randomness is Bad in computer programs, but maybe that's just the systems and languages people who want machines to be predictable. This is a silly idea, really, as with randomness, you can beat adversaries that (even extremely sophisticated) determinism cannot. Also, it's often a lot easier to be random than it is to be deterministic in a complicated fashion. These things seem rather important for topics like oh, computer security. Perhaps the coolest use for pseudo-random number generators is in synchronization of wireless devices via frequency hopping.
There are a couple of interesting points derived from Shannon's information theory about randomness and determinism. For instance, my esteemed advisor showed that when a technologies uses electromagnetic radiation (like radio waves) to transmit information, it has the same power spectrum as black-body radiation. This little result apparently ended up in a science-fiction book by Ian Stewart in which the cosmic microwave background radiation was actually a message from someone-or-other - was it God or an ancient alien race? (Stewart has also written a book on randomness, chaos and mathematics, which I'll have to pick up sometime.)
Here's an interesting gedanken experiment with regard to competition and randomness. Consider the case where you are competing against some adversary (e.g., your next-door neighbor, or, if you like, gay-married terrorists) in a game of dramatic consequence. Let's assume that you both will pursue strategies that are not completely random (that is, you can occasionally rely upon astrology or dice to make a decision, but not all the time). If you both are using sufficiently sophisticated strategies (and perhaps have infinite computational power to analyze your opponent's past behavior and make predictions about future behavior), then your opponent's actions will appear as if to be drawn from a completely random strategy; as will your own. That is, if you can detect some correlation or pattern in your opponent's strategy, then naturally you can use that to your advantage. But if your opponent knows that you will do this, which is a logical assumption, then your opponent will eliminate that structure. (This point raises an interesting question for stock marketers - because we have limited computational power, are we bound to create exploitable structure in the way we buy and sell stocks?)
The symmetry between really complicated determinism and apparent randomness is a much more universally useful property than I think it's given credit for, particularly in the world of modeling of complex systems (like the stock market, navigation on a network, and avalanches). When faced with such a system that you want to model, the right strategy to pursue is probably something like: 1) select the most simple mechanisms that are required to produce the desired behavior and then 2) use randomness for everything else. You could say that your model then has "zero intelligence", but in light of our gedanken experiment, perhaps that's a misnomer. Ultimately, if the model works, you have successfully demonstrated that a lot of the fine structure of the world that may appear to matter to some people doesn't actually matter at all (at least for the property you modeled), and that the assumed mechanisms are, at most, the necessary set for your modeled property. The success of such random models is very impressive and begs the question that does it make any difference in the end if we believe that human society (or whatever system was modeled) is not largely random? This is exactly the kind of idea that Jared Diamond explores in his recent book on the failure of past great civilizations - maybe life really is just random, and we're too stubborn to admit it.
posted March 13, 2005 12:26 AM in Thinking Aloud | permalink | Comments (0)
February 21, 2005
Global patterns in terrorism; follow-up
Looks like my article with Maxwell Young is picking up some more steam. Phillip Ball, a science writer who often writes for the Nature Publishing Group, authored a very nice little piece which draws heavily on our results. You can read the piece itself here. It's listed under the "muse@nature" section, and I'm not quite sure what that means, but the article is nicely thought-provoking. Here's an excerpt from it:
And the power-law relationship implies that the biggest terrorist attacks are not 'outliers': one-off events somehow different from the all-too-familiar suicide bombings that kill or maim just a few people. Instead, it suggests that they are somehow driven by the same underlying mechanism.
Similar power-law relationships between size and frequency apply to other phenomena, such as earthquakes and fluctuations in economic markets. This indicates that even the biggest, most infrequent earthquakes are created by the same processes that produce hordes of tiny ones, and that occasional market crashes are generated by the same internal dynamics of the marketplace that produce daily wobbles in stock prices. Analogously, Clauset and Young's study implies some kind of 'global dynamics' of terrorism.
Moreover, a power-law suggests something about that mechanism. If every terrorist attack were instigated independently of every other, their size-frequency relationship should obey the 'gaussian' statistics seen in coin-tossing experiments. In gaussian statistics, very big fluctuations are extremely rare - you will hardly ever observe ten heads and ninety tails when you toss a coin 100 times. Processes governed by power-law statistics, in contrast, seem to be interdependent. This makes them far more prone to big events, which is why giant tsunamis and market crashes do happen within a typical lifetime. Does this mean that terrorist attacks are interdependent in the same way?
Here's a bunch of other places that have picked it up or are discussing it; the sites with original coverage about our research are listed first, while the rest are (mostly) just mirroring other sites:
(February 10) Physics Web (news site [original])
(February 18) Nature News (news site [original])
(March 2) World Science (news site [original])
(March 5) Watching America (news? (French) (Google cache) [original])
(March 5) Brookings Institute (think tank [original])
(March 19) Die Welt (one of the big three German daily newspapers (translation) [original] (in German))
3 Quarks Daily (blog, this is where friend Cosma Shalizi originally found the Nature pointer)
Science Forum (a lovely discussion)
The Anomalist (blog, Feb 18 news)
Science at ORF.at (news blog (in German))
Indymedia@UK (blog)
Wissenschaft-online (news (in German))
Economics Roundtable (blog)
Physics Forum (discussion)
Spektrum Direkt (news (in German))
Manila Times (Philippines news)
Rantburg (blog, good discussion in the comments)
Unknown (blog (in Korean))
Neutrino Unbound (reading list)
Discarded Lies (blog)
Global Guerrilas (nice blog by John Robb who references our work)
TheMIB (blog)
NewsTrove (news blog/archive)
The Green Man (blog, with some commentary)
Sapere (newspaper (in Italian)
Almanacco della Scienza (news? (in Italian))
Logical Meme (conservative blog)
Money Science (discussion forum (empty))
Always On (blog with discussion)
Citebase ePrint (citation information)
Crumb Trail (blog, thoughtful coverage)
LookSmart's Furl (news mirror?)
A Dog That Can Read Physics Papers (blog (in Japanese))
Tyranny Response Unit News (blog)
Chiasm Blog (blog)
Focosi Politics (blog?)
vsevcosmos LJ (blog)
larnin carves the blogck (blog (German?))
Brothers Judd (blog)
Gerbert (news? (French))
Dr. Frantisek Slanina (homepage, Czech)
Ryszard Benedykt (some sort of report/paper (Polish))
Mohammad Khorrami (translation of Physics Web story (Persian))
CyberTribe (blog)
Feedz.com (new blog)
The Daily Grail (blog)
Wills4223 (blog)
Mathematics Magazine
Physics Forum (message board)
CiteULike
Tempers Ball (message board)
A lot of these places reference each other. Seems like most of them are getting their information from either our arxiv posting, the PhysicsWeb story, or now the Nature story. I'll keep updating this list, as more places pick it up.
Update: It's a little depressing to me that the conservatives seem to be latching onto the doom-and-gloom elements of our paper as being justification for the ill-named War on Terrorism.
Update: A short while ago, we were contacted by a reporter for Die Welt, one of the three big German daily newspapers, who wanted to do a story on our research. If the story ever appears online, I'll post a link to it.
posted February 21, 2005 12:33 PM in Scientifically Speaking | permalink | Comments (1)
February 09, 2005
Global patterns in terrorism
Although the severity of terrorist attacks may seem to be either random or highly planned in nature, it turns out that in the long-run, it is neither. By studying the set of all terrorist attacks worldwide between 1968 and 2004, we show that a simple mathematical rule, a power law with exponent close to two, governs the frequency and severity of attacks. Thus, if history is any basis to predict the future, we can predict with some confidence how long it will be before the next catastrophic attack will occur somewhere in the world.
In joint work with Max Young, we've discovered the appearance of a surprising global pattern in terrorism over the past 37 years. The brief write up of our findings is on arXiv.org, and can be found here.
Update: PhysicsWeb has done a brief story covering this work as well. The story is fairly reasonable, although the writer omitted a statement I made about caution with respect to this kind of work. So, here it is:
Generally, one should be cautious when applying the tools of one field (e.g., physics) to make statements in another (e.g., political science) as in this case. The results here turned out to be quite nice, but in approaching similar questions in the future, we will continue to exercise that caution.
posted February 9, 2005 10:30 PM in Scientifically Speaking | permalink | Comments (2)
February 03, 2005
Our ignorance of intelligence
A recent article in the New York Times, which is itself a review of a review article that recently appeared in Nature Neuroscience Reviews by the oddly named Avian Brain Nomenclature Consortium, about the incredible intelligence of certain bird species has prompted me to dump some thoughts about the abstract quality of intelligence, and more importantly, where it comes from. Having also recently finished reading On Intelligence by Jeff Hawkins (yes, that one), I've returned to my once and future fascination with that ephemeral and elusive quality that is "intelligence". We'll return to that shortly, but first let's hear some amazing things, from the NYTimes article, about what smart birds can do.
"Magpies, at an earlier age than any other creature tested, develop an understanding of the fact that when an object disappears behind a curtain, it has not vanished.
At a university campus in Japan, carrion crows line up patiently at the curb waiting for a traffic light to turn red. When cars stop, they hop into the crosswalk, place walnuts from nearby trees onto the road and hop back to the curb. After the light changes and cars run over the nuts, the crows wait until it is safe and hop back out for the food.
Pigeons can memorize up to 725 different visual patterns, and are capable of what looks like deception. Pigeons will pretend to have found a food source, lead other birds to it and then sneak back to the true source.
Parrots, some researchers report, can converse with humans, invent syntax and teach other parrots what they know. Researchers have claimed that Alex, an African gray, can grasp important aspects of number, color concepts, the difference between presence and absence, and physical properties of objects like their shapes and materials. He can sound out letters the same way a child does."
Amazing. What is even more surprising is that the structure of the avian brain is not like the mammalian brain at all. In mammals (and especially so in humans), the so-called lower regions of the brain have been enveloped by a thin sheet of cortical cells called the neo-cortex. This sheet is the base of human intelligence and is incredibly plastic. Further, it's assumed most of the control for many basic functions like breathing and hunger. The neocortex's pre-eminence is what allows people to consciously starve themselves to death. Arguably, it's the seat of free will (which I will blog about on a later date).
So how is it that birds, without a neocortex, can be so intelligent? Apparently, they have evolved an set of neurological clusters that are functionally equivalent to the mammal's neocortex, and this allow them to learn and predict complex phenomena. The equivalence is an important point in support of the belief that intelligence is independent of the substrate on which it is based; here, we mean specifically the types of supporting structures, but this independence is a founding principle of the dream of artificial intelligence (which is itself a bit of a misnomer). If there is more than one way that brains can create intelligent behavior, it is reasonable to wonder if there is more than one kind of substance from which to build those intelligent structures, e.g., transitors and other silicon parts.
It is this idea of independence that lies at the heart of Hawkins' "On Intelligence", in which he discusses his dream of eventually understanding the algorithm that runs on top of the neurological structures in the neocortex. Once we understand that algorithm, he dreams that humans will coexist with and cultivate a new species of intelligent machines that never get cranky, never have to sleep and can take care of mundanities like driving humans around, and crunching through data. Certainly a seductive and utopian future, quite unlike the uninterestingly, technophobic, distopian futures that Hollywood dreams up (at some point, I'll blog about popular culture's obsession with technophobia and its connection with the ancient fear of the unknown).
But can we reasonably expect that the engine of science, which has certainly made some astonishing advances in recent years, will eventually unravel the secret of intelligence? Occasionally, my less scientifically-minded friends have asked me to make my prediction on this topic (see previous reference to the fear-of-the-unknown). My response is, and will continue to be, that "intelligence" is, first of all, a completely ill-defined term as whenever we make machines do something surprisingly clever, critics just change the definition of intelligence. But excepting that slipperiness, I do not think we will realize Hawkins' dream of intelligent machines within my lifetime, and perhaps not within my children's either. What the human brain does is phenomenally complicated, and we are just now beginning to understand its most basic functions, let alone understand how they interact or even how they adapt over time. Combined with the complicated relationship between genetics and brain-structure (another interesting question: how does the genome store the algorithms that allow the brain to learn?), it seems like the quest of understanding human intelligence will keep many scientists employed for many many years. That all being said, I would love to be proved wrong.
Computer: tea; Earl Grey; hot.
Update 3 October 2012: In the news today is a new study at PNAS on precisely this topic, by Dugas-Ford, Rowell, and Ragsdale, "Cell-type homologies and the origins of the neocortex." The authors use a clever molecular marker approach to show that the cells that become the neocortex in mammals form different, but identifiable structures in birds and lizards, with all three neural structures performing similar neurological functions. That is, they found convergent evolution in the functional behavior of different neurological architectures in these three groups of species. What seems so exciting about this discovery is that having multiple solutions to the same basic problem should help us identify the underlying symmetries that form the basis for intelligent behavior.
posted February 3, 2005 02:17 AM in Obsession with birds | permalink | Comments (0)